Predicting Length Of Stay In Hospital Using Electronic Records Available At The Time Of Admission
DIGITAL PERSONALIZED HEALTH AND MEDICINE(2020)
摘要
Predicting a patient's hospital length of stay (LoS) can help manage staffing. In this paper, we explore LoS prediction for a large group of patients admitted non-electively. We use information available at admission, including demographics, acute and long-term diagnoses and physiological tests results. Data were extracted from the electronic health records (EHR), so that the LoS prediction would not require additional data entry. Although the data can be accessed, the system does not present a unified view of the data for one patient: to resolve this we designed a process of cleaning and combining data for each patient. The data was used to fit semi-parametric, parametric and competing outcomes survival models. All models performed similarly, with concordance of approximately 0.7. Calibration results showed underestimation of predicted discharges for patients with high discharge probabilities and overestimation of predicted discharges for those with low discharge probabilities. The main challenges in operationalizing LoS predictions are delays in entering admissions data into EHR and absent data about non-medical factors determining discharges.
更多查看译文
关键词
Length of Stay, Bed management, Survival analysis, Medical data transformation
AI 理解论文
溯源树
样例
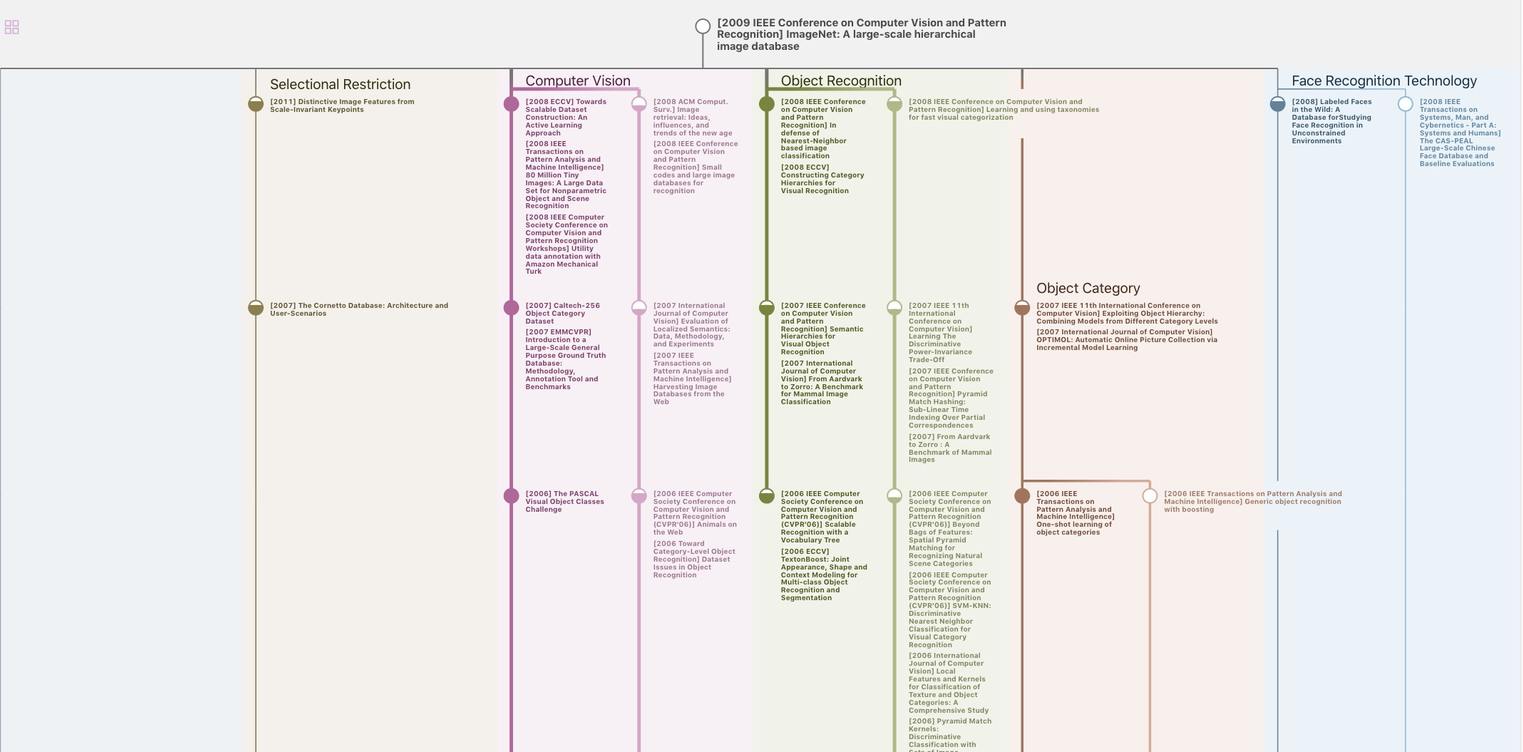
生成溯源树,研究论文发展脉络
Chat Paper
正在生成论文摘要