Machine Learning Explainability In Breast Cancer Survival
DIGITAL PERSONALIZED HEALTH AND MEDICINE(2020)
摘要
Machine Learning (ML) can improve the diagnosis, treatment decisions, and understanding of cancer. However, the low explainability of how "black box" ML methods produce their output hinders their clinical adoption. In this paper, we used data from the Netherlands Cancer Registry to generate a ML-based model to predict 10-year overall survival of breast cancer patients. Then, we used Local Interpretable Model-Agnostic Explanations (LIME) and SHapley Additive exPlanations (SHAP) to interpret the model's predictions. We found that, overall, LIME and SHAP tend to be consistent when explaining the contribution of different features. Nevertheless, the feature ranges where they have a mismatch can also be of interest, since they can help us identifying "turning points" where features go from favoring survived to favoring deceased (or vice versa). Explainability techniques can pave the way for better acceptance of ML techniques. However, their evaluation and translation to real-life scenarios need to be researched further.
更多查看译文
关键词
Artificial Intelligence, interpretability, oncology, prediction model
AI 理解论文
溯源树
样例
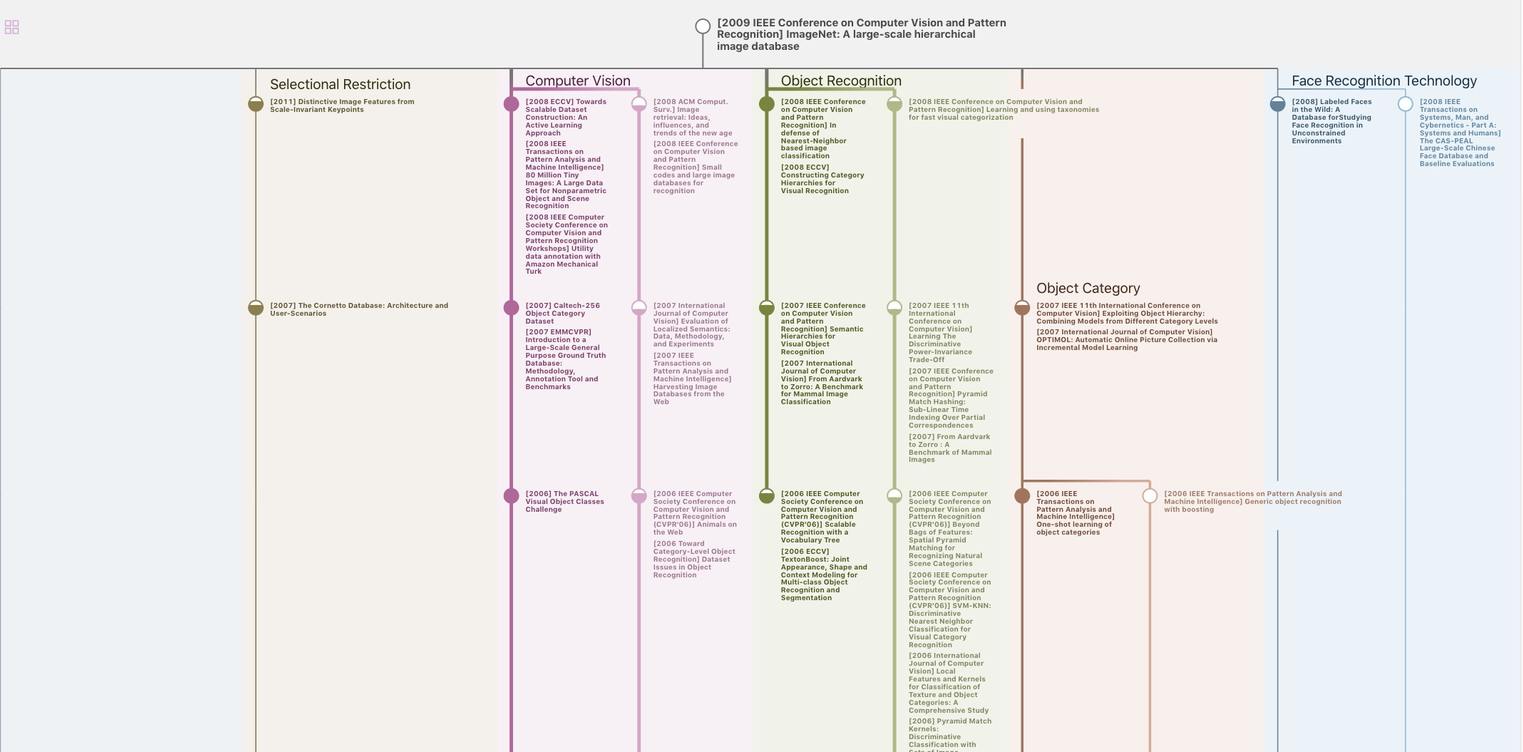
生成溯源树,研究论文发展脉络
Chat Paper
正在生成论文摘要