Joint Object Detection and Multi-Object Tracking with Graph Neural Networks
arxiv(2020)
摘要
Object detection and data association are critical components in multi-object tracking (MOT) systems. Despite the fact that the two components are dependent on each other, prior works often design detection and data association modules separately which are trained with separate objectives. As a result, one cannot back-propagate the gradients and optimize the entire MOT system, which leads to sub-optimal performance. To address this issue, recent works simultaneously optimize detection and data association modules under a joint MOT framework, which has shown improved performance in both modules. In this work, we propose a new instance of joint MOT approach based on Graph Neural Networks (GNNs). The key idea is that GNNs can model relations between variable-sized objects in both the spatial and temporal domains, which is essential for learning discriminative features for detection and data association. Through extensive experiments on the MOT15/16/17/20 datasets, we demonstrate the effectiveness of our GNN-based joint MOT approach and show state-of-the-art performance for both detection and MOT tasks. Our code is available at: https://github.com/yongxinw/GSDT
更多查看译文
关键词
joint object detection,graph neural networks,critical components,multiobject tracking systems,data association modules,separate objectives,MOT system,sub-optimal performance,MOT framework,GNN-based joint MOT approach,discriminative feature learning
AI 理解论文
溯源树
样例
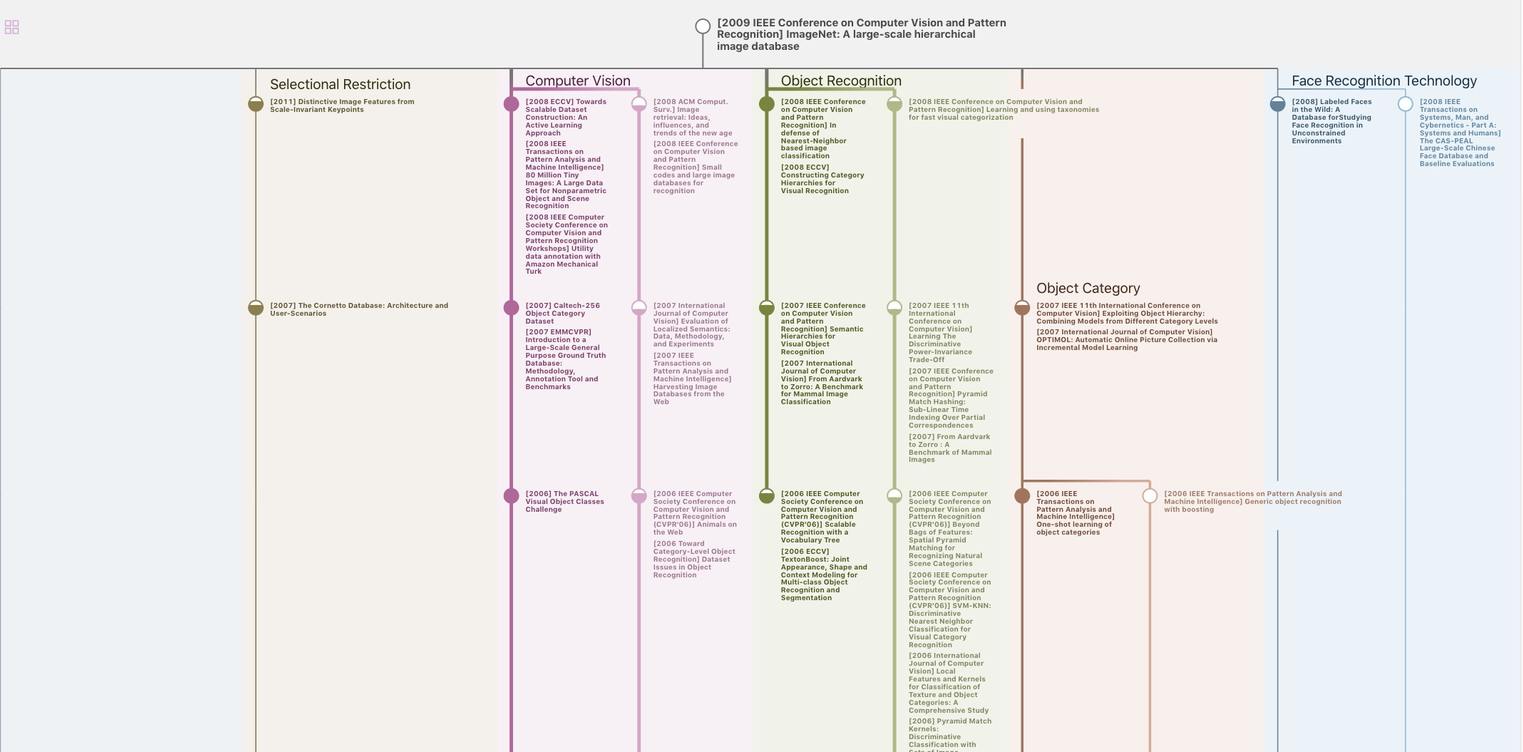
生成溯源树,研究论文发展脉络
Chat Paper
正在生成论文摘要