Increased-Range Unsupervised Monocular Depth Estimation
arxiv(2020)
摘要
Unsupervised deep learning methods have shown promising performance for single-image depth estimation. Since most of these methods use binocular stereo pairs for self-supervision, the depth range is generally limited. Small-baseline stereo pairs provide small depth range but handle occlusions well. On the other hand, stereo images acquired with a wide-baseline rig cause occlusions-related errors in the near range but estimate depth well in the far range. In this work, we propose to integrate the advantages of the small and wide baselines. By training the network using three horizontally aligned views, we obtain accurate depth predictions for both close and far ranges. Our strategy allows to infer multi-baseline depth from a single image. This is unlike previous multi-baseline systems which employ more than two cameras. The qualitative and quantitative results show the superior performance of multi-baseline approach over previous stereo-based monocular methods. For 0.1 to 80 meters depth range, our approach decreases the absolute relative error of depth by 24 on a single Nvidia1080 GPU, making it useful for practical applications.
更多查看译文
关键词
depth,estimation,increased-range
AI 理解论文
溯源树
样例
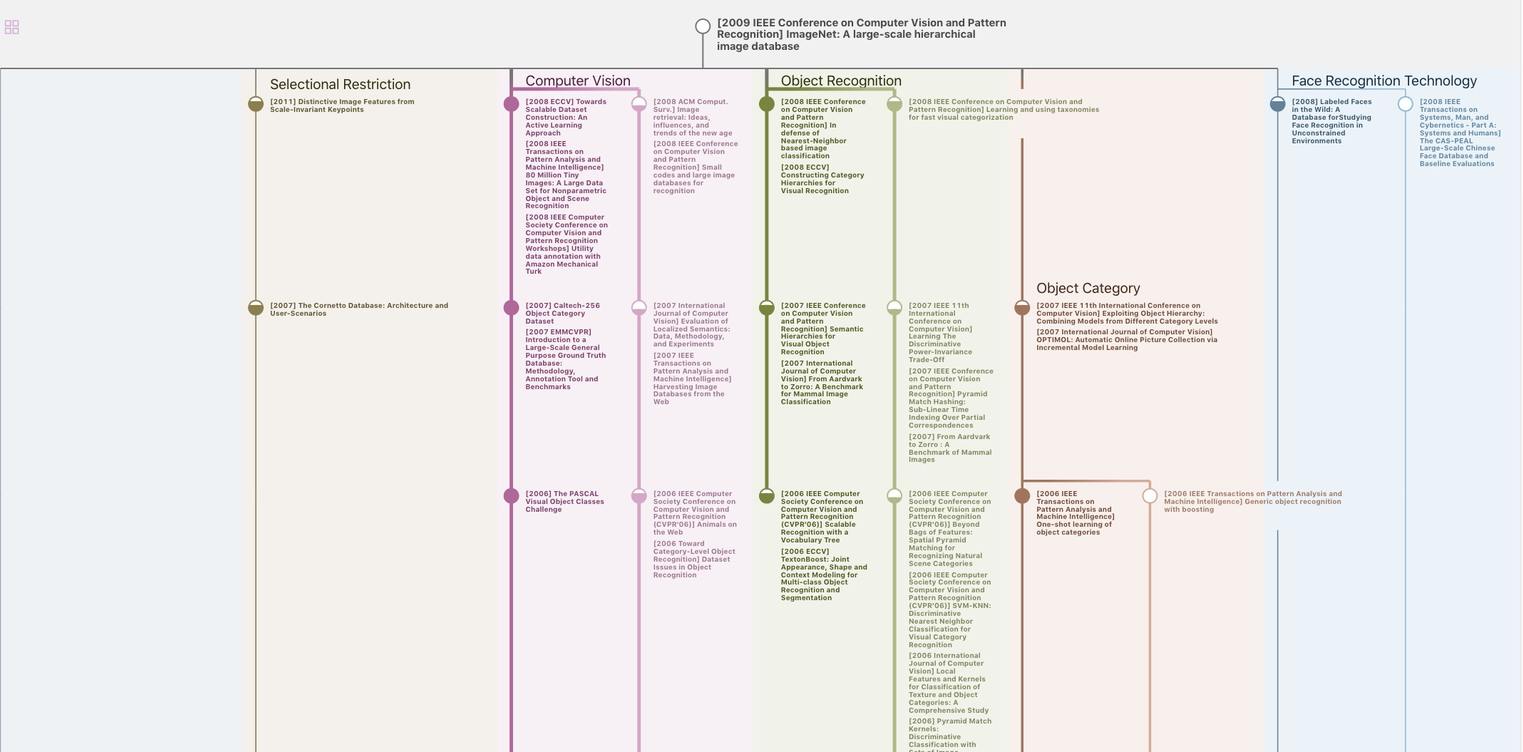
生成溯源树,研究论文发展脉络
Chat Paper
正在生成论文摘要