Aligning Time Series On Incomparable Spaces
24TH INTERNATIONAL CONFERENCE ON ARTIFICIAL INTELLIGENCE AND STATISTICS (AISTATS)(2021)
摘要
Dynamic time warping (DTW) is a useful method for aligning, comparing and combining time series, but it requires them to live in comparable spaces. In this work, we consider a setting in which time series live on different spaces without a sensible ground metric, causing DTW to become ill-defined. To alleviate this, we propose Gromov dynamic time warping (GDTW), a distance between time series on potentially incomparable spaces that avoids the comparability requirement by instead considering intra-relational geometry. We demonstrate its effectiveness at aligning, combining and comparing time series living on incomparable spaces. We further propose a smoothed version of GDTW as a differentiable loss and assess its properties in a variety of settings, including barycentric averaging, generative modeling and imitation learning.
更多查看译文
关键词
time series
AI 理解论文
溯源树
样例
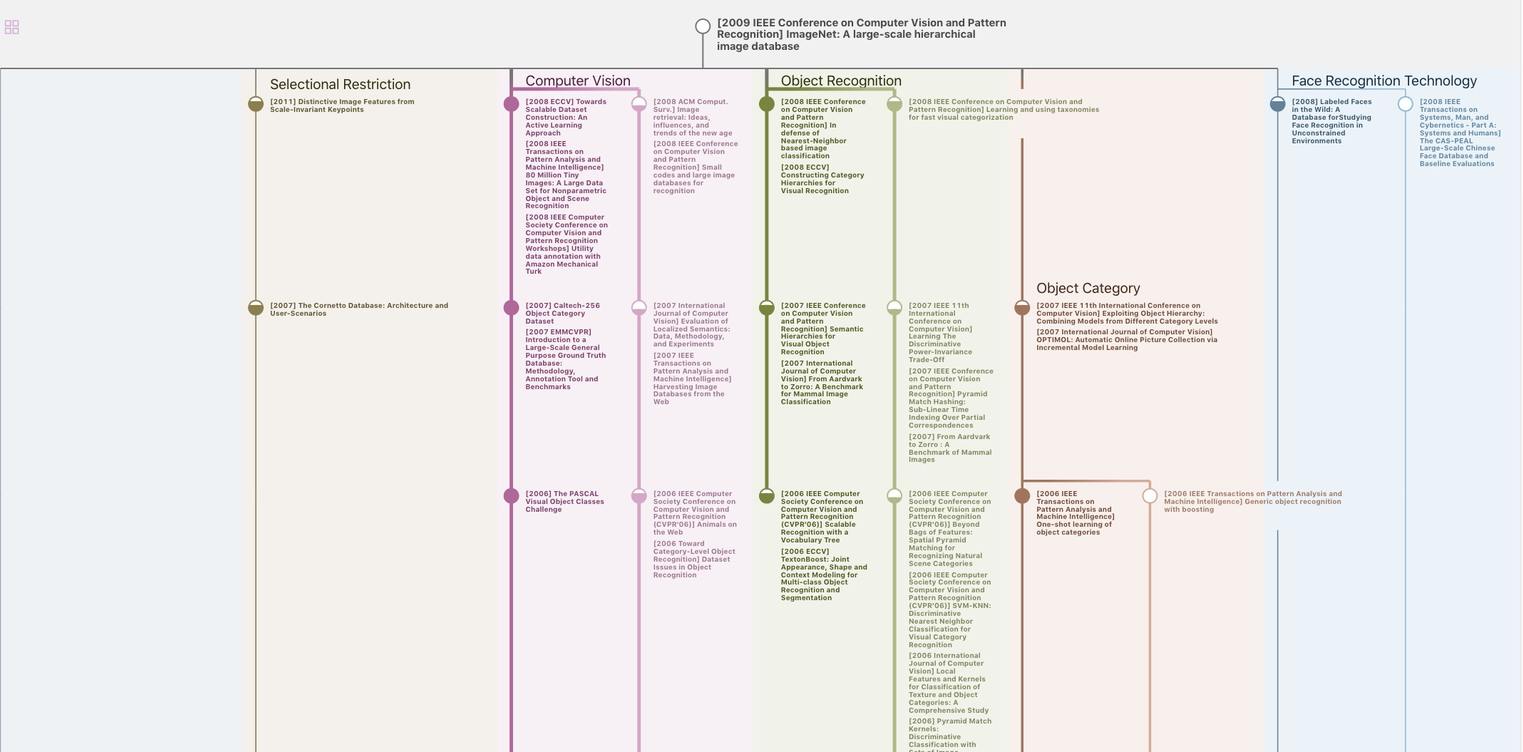
生成溯源树,研究论文发展脉络
Chat Paper
正在生成论文摘要