Bidirectionally Self-Normalizing Neural Networks
arxiv(2022)
摘要
The problem of vanishing and exploding gradients has been a long-standing obstacle that hinders the effective training of neural networks. Despite various tricks and techniques that have been employed to alleviate the problem in practice, there still lacks satisfactory theories or provable solutions. In this paper, we address the problem from the perspective of high-dimensional probability theory. We provide a rigorous result that shows, under mild conditions, how the vanishing/exploding gradients problem disappears with high probability if the neural networks have sufficient width. Our main idea is to constrain both forward and backward signal propagation in a nonlinear neural network through a new class of activation functions, namely Gaussian-Poincaré normalized functions, and orthogonal weight matrices. Experiments on both synthetic and real-world data validate our theory and confirm its effectiveness on very deep neural networks when applied in practice.
更多查看译文
关键词
Neural networks,Vanishing/exploding gradient problem,Training,Optimization
AI 理解论文
溯源树
样例
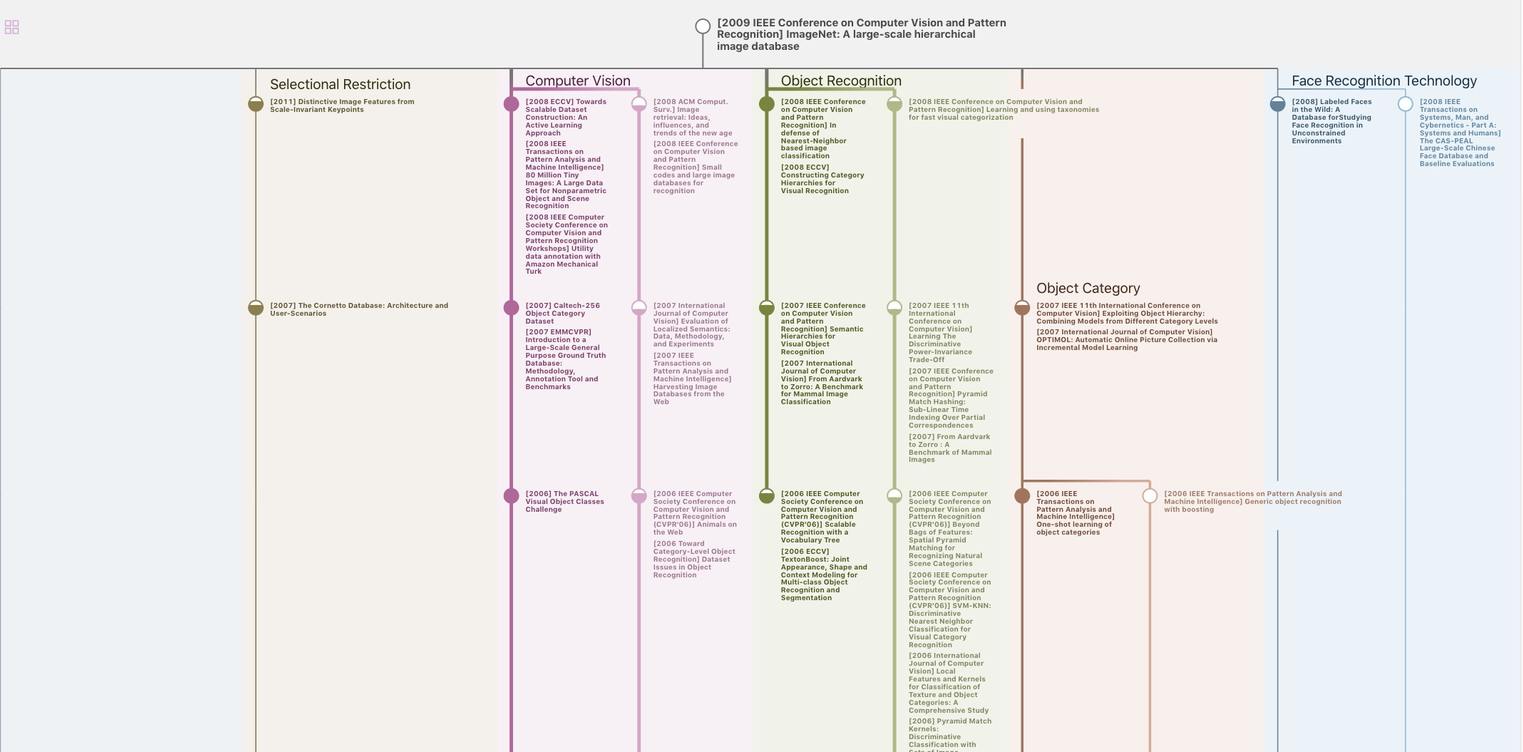
生成溯源树,研究论文发展脉络
Chat Paper
正在生成论文摘要