Decoupling Shrinkage and Selection in Gaussian Linear Factor Analysis
BAYESIAN ANALYSIS(2024)
摘要
Factor analysis is a popular method for modeling dependence in mul-tivariate data. However, determining the number of factors and obtaining a sparse orientation of the loadings are still major challenges. In this paper, we propose a decision-theoretic approach that brings to light the relationship between model fit, factor dimension, and sparse loadings. This relation is done through a summary of the information contained in the multivariate posterior. A two-step strategy is used in our method. First, given the posterior samples from the Bayesian factor analysis model, a series of point estimates with a decreasing number of factors and different levels of sparsity are recovered by minimizing an expected penalized loss function. Second, the degradation in model fit between the posterior of the full model and the recovered estimates is displayed in a summary. In this step, a criterion is proposed for selecting the factor model with the best trade-off be-tween fit, sparseness, and factor dimension. The findings are illustrated through a simulation study and an application to personality data. We used different prior choices to show the flexibility of the proposed method.
更多查看译文
关键词
Bayesian factor analysis,model selection,sparse loadings,factor dimension,loss function
AI 理解论文
溯源树
样例
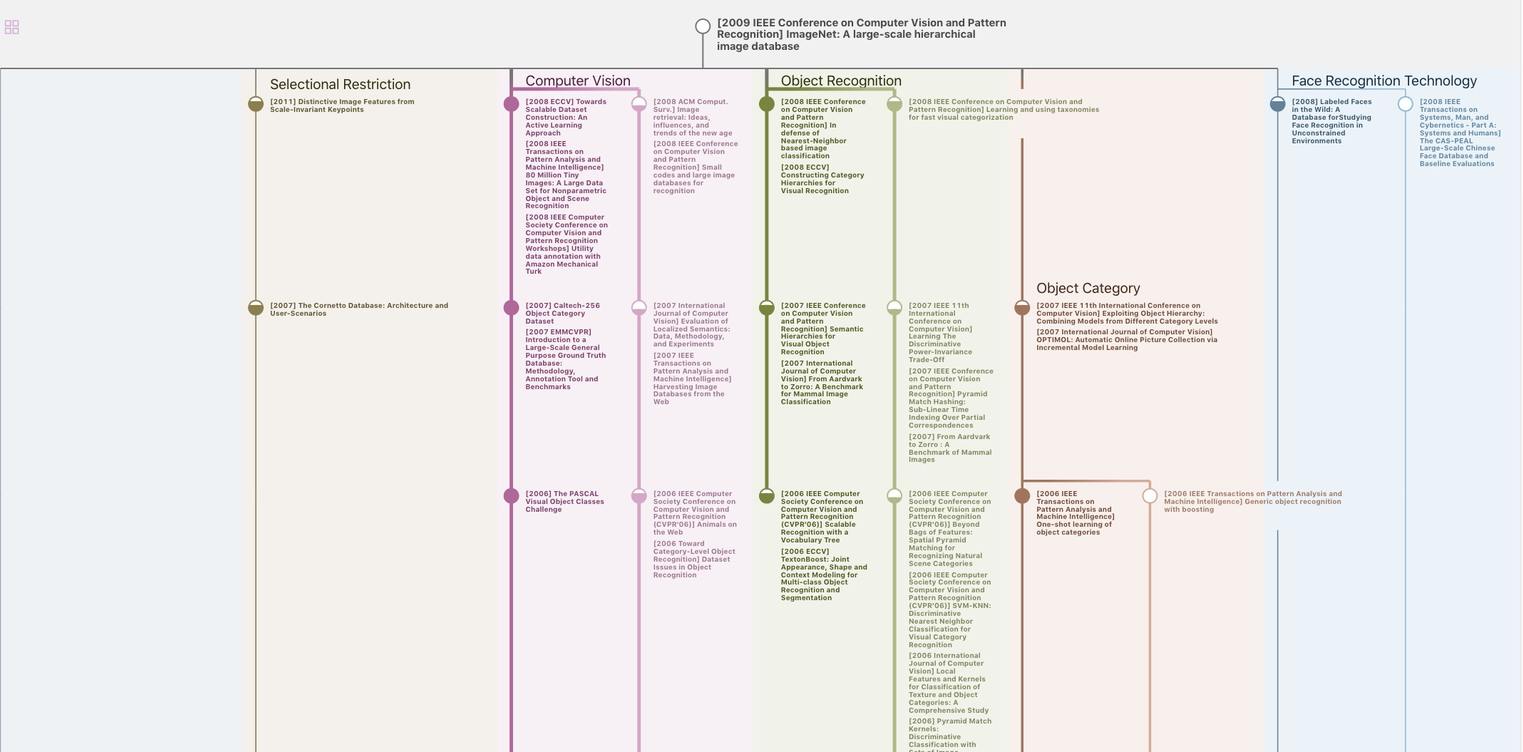
生成溯源树,研究论文发展脉络
Chat Paper
正在生成论文摘要