Identification of therapeutic targets from genetic association studies using hierarchical component analysis
BioData Mining(2020)
摘要
Background Mapping disease-associated genetic variants to complex disease pathophysiology is a major challenge in translating findings from genome-wide association studies into novel therapeutic opportunities. The difficulty lies in our limited understanding of how phenotypic traits arise from non-coding genetic variants in highly organized biological systems with heterogeneous gene expression across cells and tissues. Results We present a novel strategy, called GWAS component analysis, for transferring disease associations from single-nucleotide polymorphisms to co-expression modules by stacking models trained using reference genome and tissue-specific gene expression data. Application of this method to genome-wide association studies of blood cell counts confirmed that it could detect gene sets enriched in expected cell types. In addition, coupling of our method with Bayesian networks enables GWAS components to be used to discover drug targets. Conclusions We tested genome-wide associations of four disease phenotypes, including age-related macular degeneration, Crohn’s disease, ulcerative colitis and rheumatoid arthritis, and demonstrated the proposed method could select more functional genes than S-PrediXcan, the previous single-step model for predicting gene-level associations from SNP-level associations.
更多查看译文
关键词
Genome-wide association study,Network biology,Gene candidate discovery
AI 理解论文
溯源树
样例
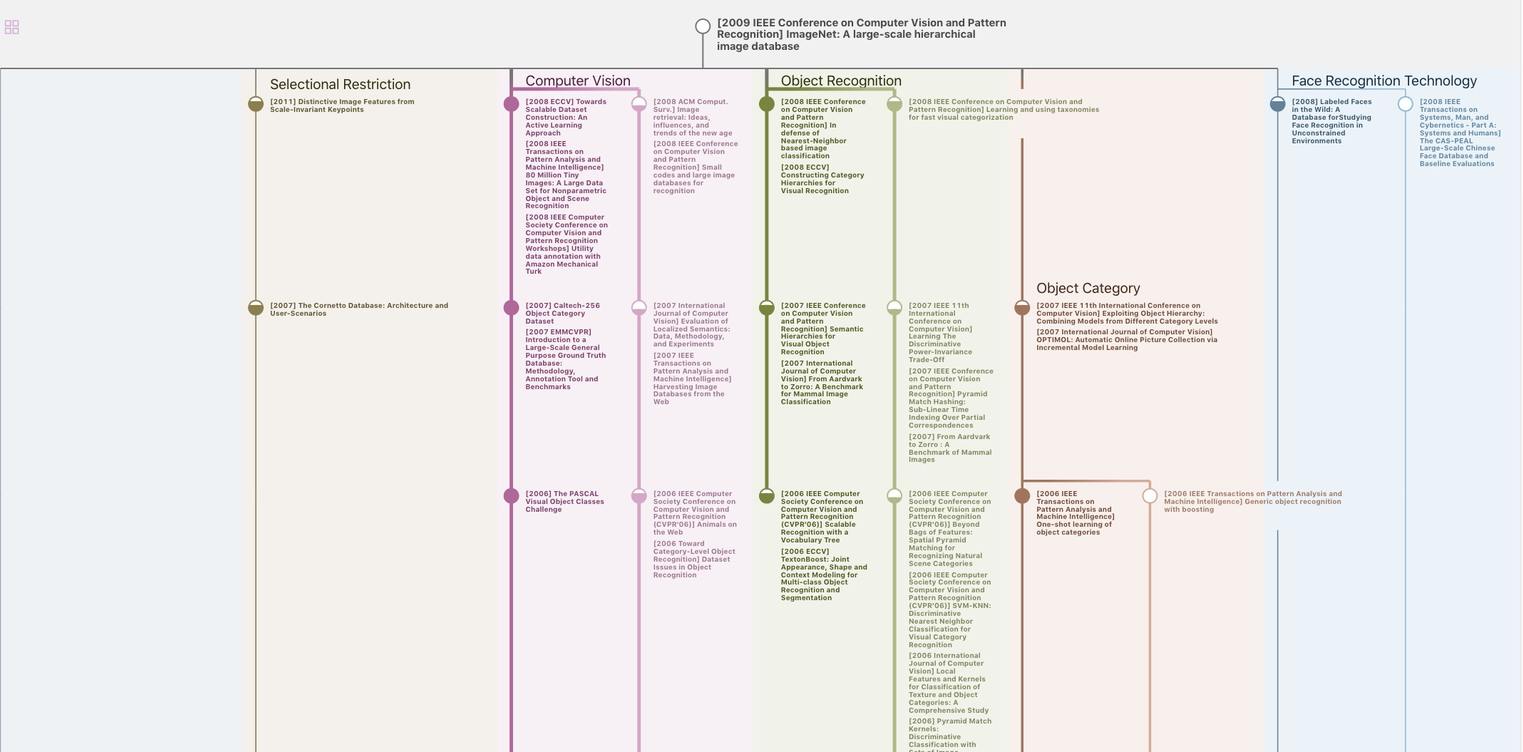
生成溯源树,研究论文发展脉络
Chat Paper
正在生成论文摘要