Revealing The Invisible With Model And Data Shrinking For Composite-Database Micro-Expression Recognition
IEEE TRANSACTIONS ON IMAGE PROCESSING(2020)
摘要
Composite-database micro-expression recognition is attracting increasing attention as it is more practical for real-world applications. Though the composite database provides more sample diversity for learning good representation models, the important subtle dynamics are prone to disappearing in the domain shift such that the models greatly degrade their performance, especially for deep models. In this article, we analyze the influence of learning complexity, including input complexity and model complexity, and discover that the lower-resolution input data and shallower-architecture model are helpful to ease the degradation of deep models in composite-database task. Based on this, we propose a recurrent convolutional network (RCN) to explore the shallower-architecture and lower-resolution input data, shrinking model and input complexities simultaneously. Furthermore, we develop three parameter-free modules (i.e., wide expansion, shortcut connection and attention unit) to integrate with RCN without increasing any learnable parameters. These three modules can enhance the representation ability in various perspectives while preserving not-very-deep architecture for lower-resolution data. Besides, three modules can further be combined by an automatic strategy (a neural architecture search strategy) and the searched architecture becomes more robust. Extensive experiments on the MEGC2019 dataset (composited of existing SMIC, CASME II and SAMM datasets) have verified the influence of learning complexity and shown that RCNs with three modules and the searched combination outperform the state-of-the-art approaches.
更多查看译文
关键词
Task analysis, Data models, Complexity theory, Feature extraction, Analytical models, Histograms, Optical imaging, Micro-expression recognition, composite database, recurrent convolutional network, model and data shrinking, parameter-free module, searchable architecture
AI 理解论文
溯源树
样例
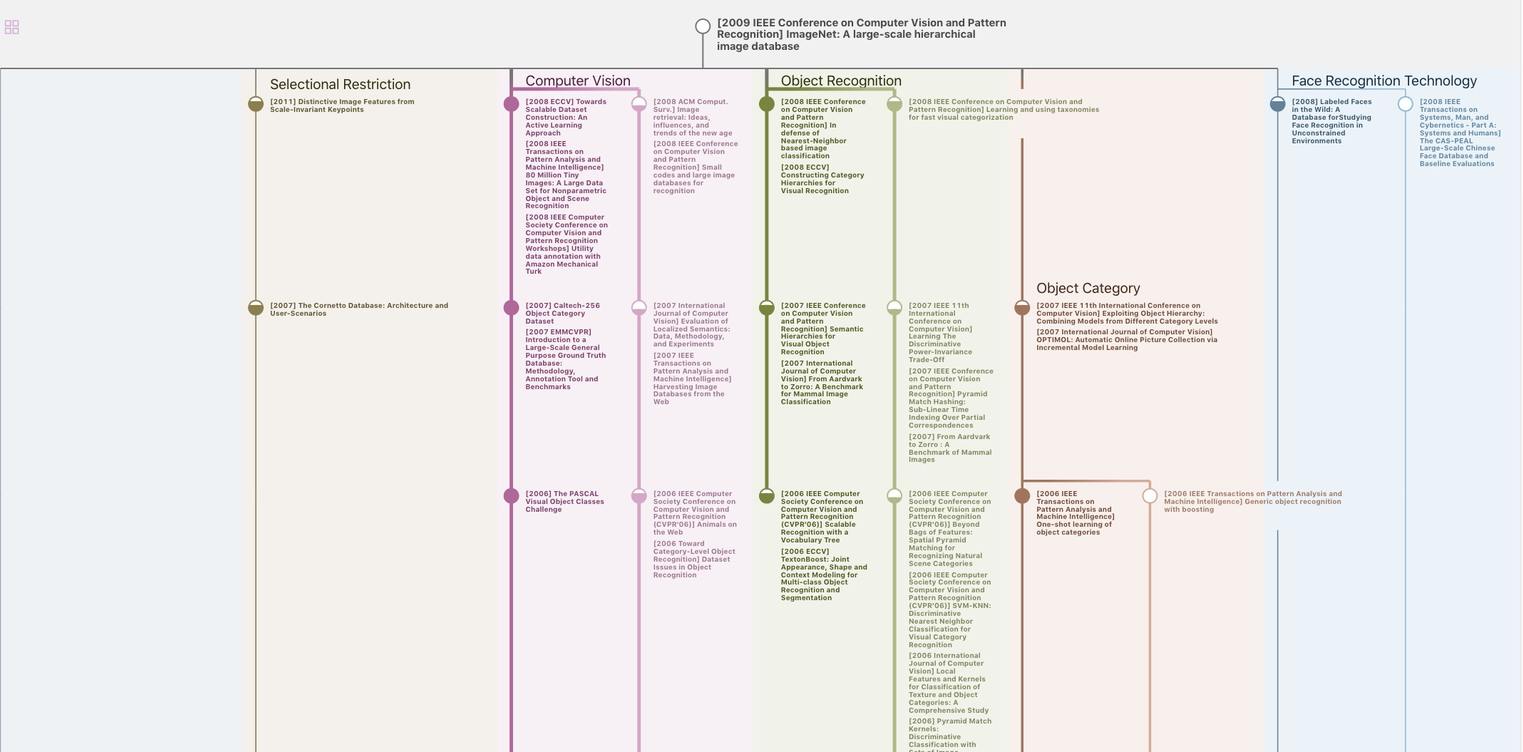
生成溯源树,研究论文发展脉络
Chat Paper
正在生成论文摘要