Machine-Learning Classifiers in Discrimination of Lesions Located in the Anterior Skull Base.
FRONTIERS IN ONCOLOGY(2020)
摘要
Purpose:The aim of this study was to investigate the diagnostic value of machine-learning models with radiomic features and clinical features in preoperative differentiation of common lesions located in the anterior skull base. Methods:A total of 235 patients diagnosed with pituitary adenoma, meningioma, craniopharyngioma, or Rathke cleft cyst were enrolled in the current study. The discrimination was divided into three groups: pituitary adenoma vs. craniopharyngioma, meningioma vs. craniopharyngioma, and pituitary adenoma vs. Rathke cleft cyst. In each group, five selection methods were adopted to select suitable features for the classifier, and nine machine-learning classifiers were employed to build discriminative models. The diagnostic performance of each combination was evaluated with area under the receiver operating characteristic curve (AUC), accuracy, sensitivity, and specificity calculated for both the training group and the testing group. Results:In each group, several classifiers combined with suitable selection methods represented feasible diagnostic performance with AUC of more than 0.80. Moreover, the combination of least absolute shrinkage and selection operator as the feature-selection method and linear discriminant analysis as the classification algorithm represented the best comprehensive discriminative ability. Conclusion:Radiomics-based machine learning could potentially serve as a novel method to assist in discriminating common lesions in the anterior skull base prior to operation.
更多查看译文
关键词
pituitary adenoma,meningioma,craniopharyngioma,Rathke cleft cyst,anterior skull base,radiomics,machine learning
AI 理解论文
溯源树
样例
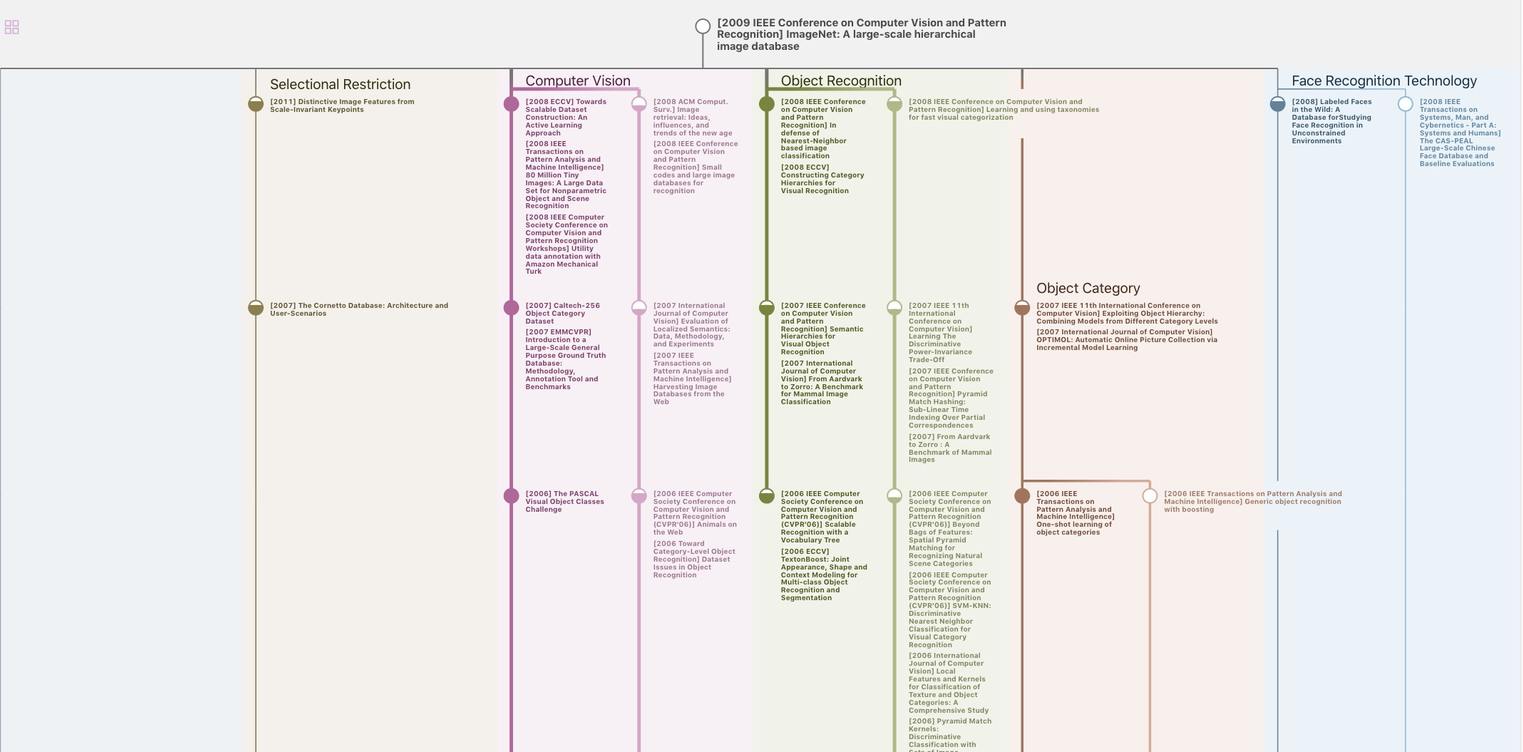
生成溯源树,研究论文发展脉络
Chat Paper
正在生成论文摘要