Improving 3-m Resolution Land Cover Mapping through Efficient Learning from an Imperfect 10-m Resolution Map.
REMOTE SENSING(2020)
摘要
Substantial progress has been made in the field of large-area land cover mapping as the spatial resolution of remotely sensed data increases. However, a significant amount of human power is still required to label images for training and testing purposes, especially in high-resolution (e.g., 3-m) land cover mapping. In this research, we propose a solution that can produce 3-m resolution land cover maps on a national scale without human efforts being involved. First, using the public 10-m resolution land cover maps as an imperfect training dataset, we propose a deep learning based approach that can effectively transfer the existing knowledge. Then, we improve the efficiency of our method through a network pruning process for national-scale land cover mapping. Our proposed method can take the state-of-the-art 10-m resolution land cover maps (with an accuracy of 81.24% for China) as the training data, enable a transferred learning process that can produce 3-m resolution land cover maps, and further improve the overall accuracy (OA) to 86.34% for China. We present detailed results obtained over three mega cities in China, to demonstrate the effectiveness of our proposed approach for 3-m resolution large-area land cover mapping.
更多查看译文
关键词
land cover mapping,high-resolution imagery,deep learning,urban environment
AI 理解论文
溯源树
样例
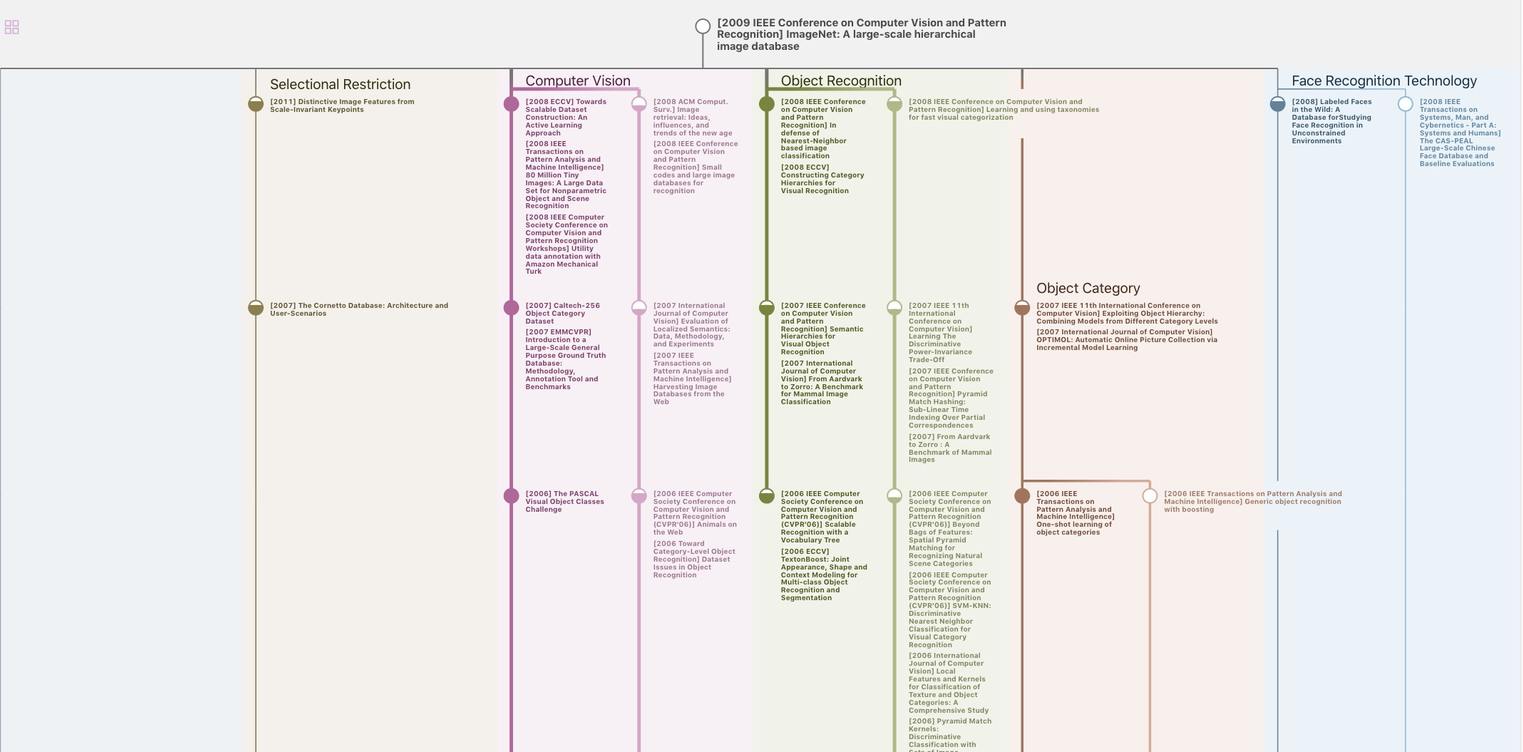
生成溯源树,研究论文发展脉络
Chat Paper
正在生成论文摘要