Texture analysis in susceptibility-weighted imaging may be useful to differentiate acute from chronic multiple sclerosis lesions
EUROPEAN RADIOLOGY(2020)
摘要
Objectives To evaluate the diagnostic performance of texture analysis (TA) applied on non-contrast-enhanced susceptibility-weighted imaging (SWI) to differentiate acute (enhancing) from chronic (non-enhancing) multiple sclerosis (MS) lesions. Methods We analyzed 175 lesions from 58 patients with relapsing-remitting MS imaged on a 3.0 T MRI scanner and applied TA on T2-w and SWI images to extract texture features. We evaluated the presence or absence of lesion enhancement on T1-w post-contrast images and performed a computational statistical analysis to assess if there was any significant correlation between the texture features and the presence of lesion activity. ROC curves and leave-one-out cross-validation were used to evaluate the performance of individual features and multiparametric models in the identification of active lesions. Results Multiple TA features obtained from SWI images showed a significantly different distribution in acute and chronic lesions (AUC, 0.617–0.720). Multiparametric predictive models based on logistic ridge regression and partial least squares regression yielded an AUC of 0.778 and 0.808, respectively. Results from T2-w images did not show any significant predictive ability of neither individual features nor multiparametric models. Conclusions Texture analysis on SWI sequences may be useful to differentiate acute from chronic MS lesions. The good diagnostic performance could help to reduce the need of intravenous contrast agent administration in follow-up MRI studies. Key Points • Texture analysis applied on SWI sequences may be useful to differentiate acute from chronic multiple sclerosis lesions • The good diagnostic performance could help to minimize the need of intravenous contrast agent administration in follow-up MRI studies
更多查看译文
关键词
Multiple sclerosis, Magnetic resonance imaging, Contrast agent, Logistic models
AI 理解论文
溯源树
样例
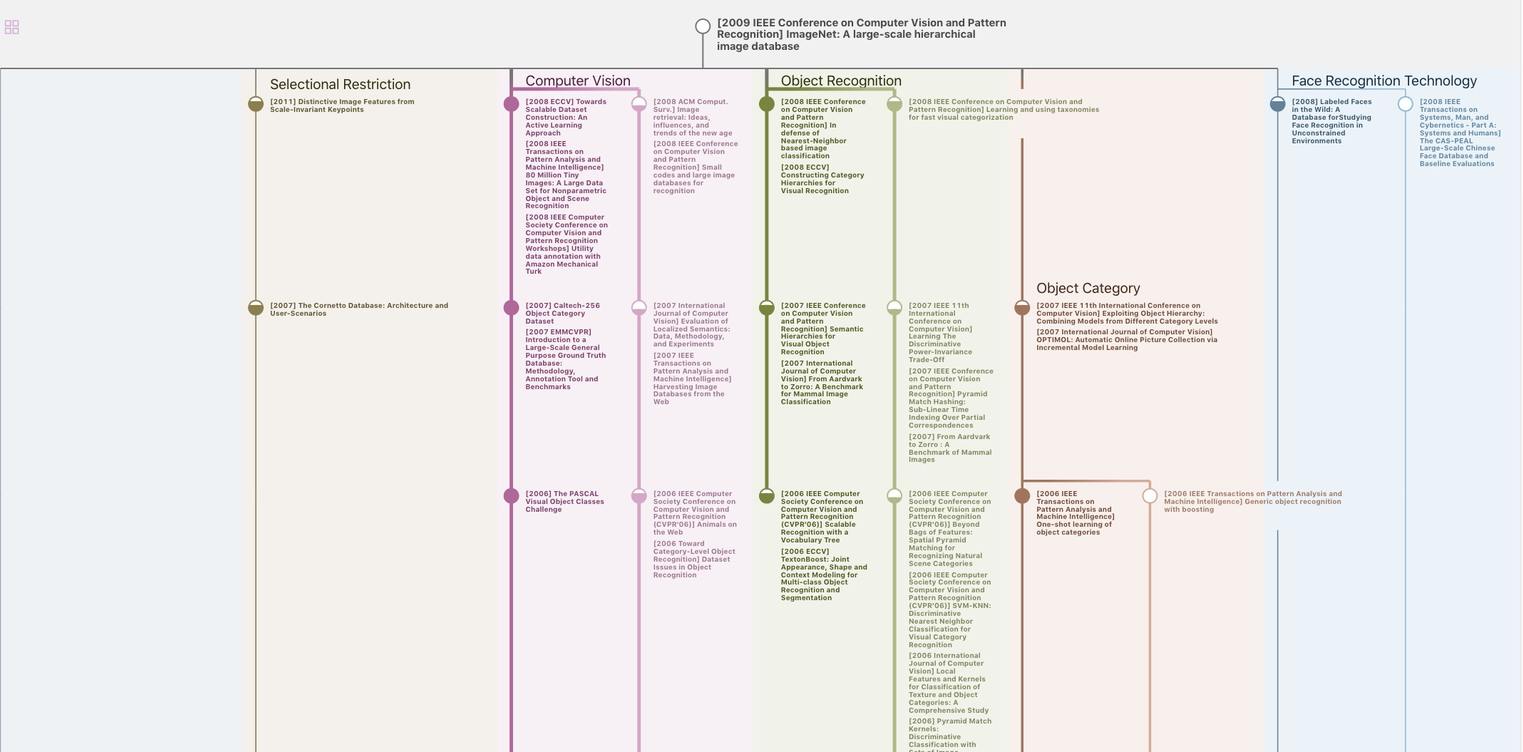
生成溯源树,研究论文发展脉络
Chat Paper
正在生成论文摘要