HGDom: Heterogeneous Graph Convolutional Networks for Malicious Domain Detection
NOMS 2020 - 2020 IEEE/IFIP Network Operations and Management Symposium(2020)
摘要
As a fundamental component of the Internet, Domain Name System (DNS) is widely abused by attackers in various cybercrimes, making malicious domain detection an essential task in network defenses. However, some well-crafted attacks with tricky techniques can not only bypass blacklists but also make some machine learning-based detection systems infeasible. In this paper, we design HGDom, an accurate and robust malicious domain detection system based on a heterogeneous graph convolutional network method. First, we jointly analyze domain features as well as the complex relations among domains, clients, and IP addresses. To capture richer information, we introduce a Heterogeneous Information Network (HIN) to model the DNS scene. Then, we propose a novel representation method named MAGCN. With a meta-path-based attention mechanism, it can handle node features and the graph structure in HIN at the same time. To our best knowledge, this is the first work to apply GCN in cyber security analysis. Comprehensive experiments over DNS data from TUNET and CERNET2 are conducted to validate the effectiveness and superiority of our proposed methods. The comparison results show that HGDom outperforms state-of-the-art approaches with promising performance. Besides, the system is decided to be deployed in production to assist with network security management for CERNET2.
更多查看译文
关键词
Malicious Domain Detection,Graph Convolutional Networks,Heterogeneous Information Networks,Metapath
AI 理解论文
溯源树
样例
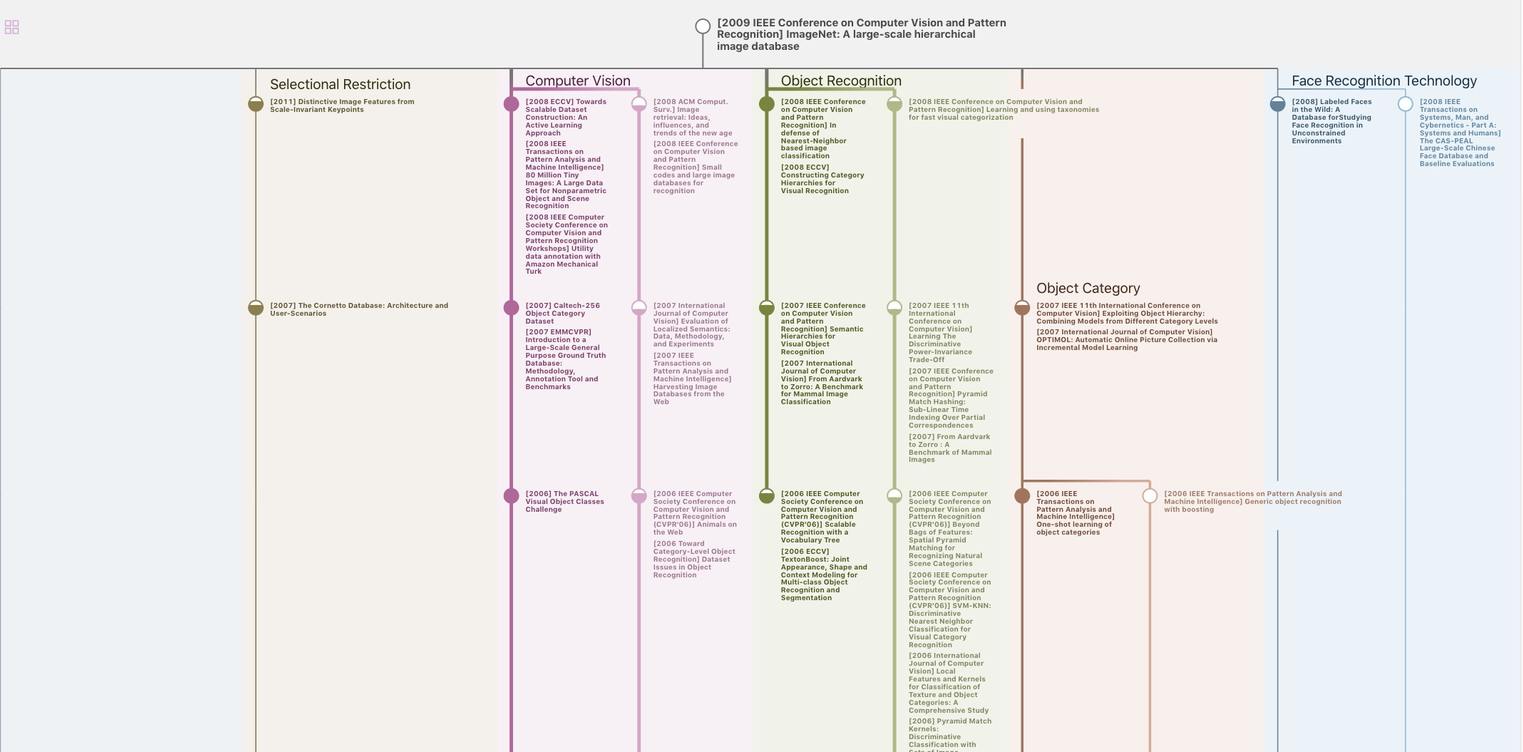
生成溯源树,研究论文发展脉络
Chat Paper
正在生成论文摘要