Efficient Real-Time Traffic Generation for 5G RAN.
NOMS(2020)
摘要
Modern telecommunication and mobile networks are increasingly complex from a resource management perspective, with diverse combinations of software and infrastructure elements that need to be configured and tuned for efficient operation with high quality of service. Increased real-time automation at all levels and time-frames is a critical tool in controlling this complexity. A key component in automation is practical and accurate simulation methods that can be used in live traffic scenarios. This paper introduces a new method with supporting algorithms for sampling key parameters from live or recorded traffic which can be used to generate large volumes of synthetic traffic with very similar rate distributions and temporal characteristics. Multiple spatial renewal processes are used to generate fractional Gaussian noise, which is scaled and transformed into a log-normal rate distribution with discrete arrival events, fitted to the properties observed in given recorded traces. This approach works well for modelling large user aggregates but is especially useful for medium sized and relatively small aggregates, where existing methods struggle to reproduce the most important properties of recorded traces. The technique is demonstrated through experimental comparisons with data collected from an operational LTE network to be highly useful in supporting self-learning and automation algorithms which can ultimately reduce complexity, increase energy efficiency, and reduce total network operation costs.
更多查看译文
关键词
Communication system traffic, Fractals, Machine learning, Parametric statistics, System simulation
AI 理解论文
溯源树
样例
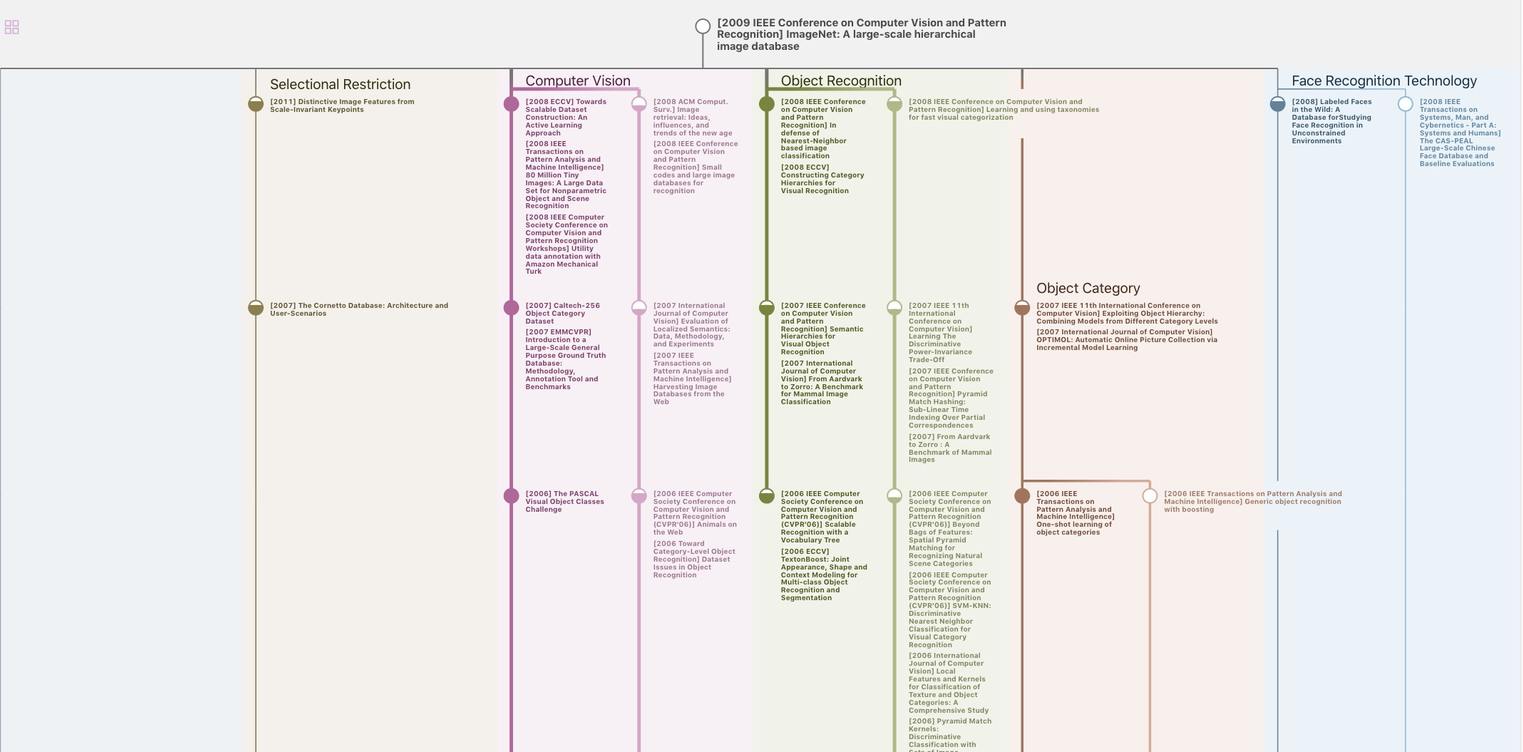
生成溯源树,研究论文发展脉络
Chat Paper
正在生成论文摘要