Unsupervised Detection of Anomalous Behavior in Wireless Devices based on Auto-Encoders.
NOMS(2020)
摘要
A major problem of wireless devices is the detection of security threats in an efficient manner. Several recent incidents show that malicious applications (apps) can find their ways to online markets (e.g., Google Play Store) and be available for download and installation. Such malicious apps can collect sensitive data from millions of users and send them to a third-party servers. In this paper, we propose a methodology that leverages the power consumption of wireless devices to build a model that makes them more robust to the presence of malicious apps. The method consists of two stages: (i) Feature Extraction where stacked Restricted Boltzmann Machine (RBM) AutoEncoders (AE) and Principal Component Analysis (PCA) are used to extract features vector based on AE’s reconstruction errors. (ii) Classifier where One-Class Support Vector Machine is trained to perform the classification task. The validation of the methodology is performed on a real measurements dataset. The obtained results show a good potential and prove that AEs’ reconstruction error can be used as a good discriminating feature. The obtained detection accuracy surpasses previously reported techniques, where it reaches up to ~ 98% in some scenarios.
更多查看译文
关键词
Malware Detection, Power Consumption Information, Denoising AutoEncoder, Wireless Devices
AI 理解论文
溯源树
样例
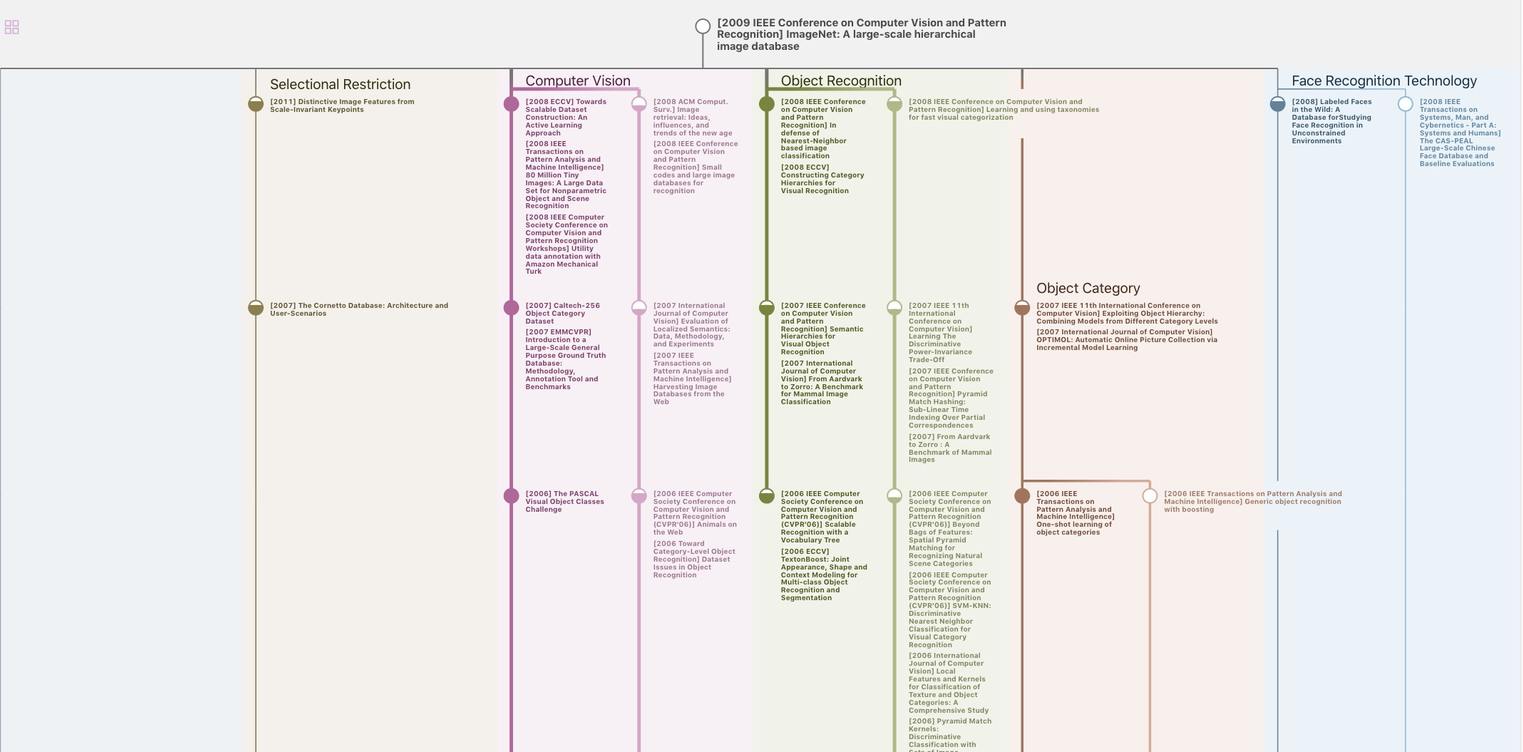
生成溯源树,研究论文发展脉络
Chat Paper
正在生成论文摘要