Additive Poisson Process: Learning Intensity of Higher-Order Interaction in Stochastic Processes
arxiv(2020)
摘要
We present the Additive Poisson Process (APP), a novel framework that can model the higher-order interaction effects of the intensity functions in stochastic processes using lower dimensional projections. Our model combines the techniques in information geometry to model higher-order interactions on a statistical manifold and in generalized additive models to use lower-dimensional projections to overcome the effects from the curse of dimensionality. Our approach solves a convex optimization problem by minimizing the KL divergence from a sample distribution in lower dimensional projections to the distribution modeled by an intensity function in the stochastic process. Our empirical results show that our model is able to use samples observed in the lower dimensional space to estimate the higher-order intensity function with extremely sparse observations.
更多查看译文
关键词
Poisson Process,Log-Linear Model,Energy-Based Model,Generalized Additive Models,Information Geometry
AI 理解论文
溯源树
样例
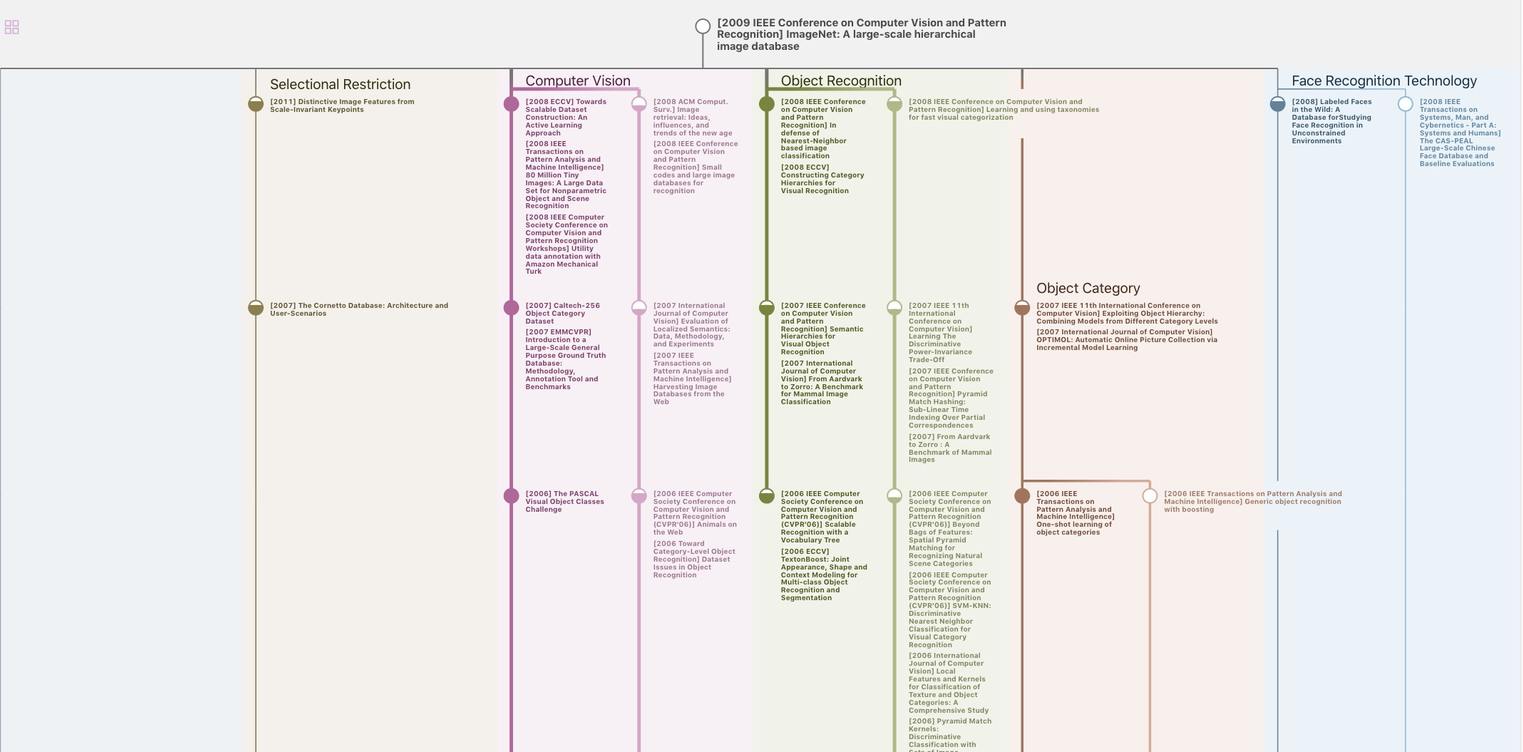
生成溯源树,研究论文发展脉络
Chat Paper
正在生成论文摘要