Fanok: Knockoffs In Linear Time
SIAM JOURNAL ON MATHEMATICS OF DATA SCIENCE(2021)
摘要
We describe a series of algorithms that efficiently implement Gaussian model-X knockoffs to control the false discovery rate on large-scale feature selection problems. Identifying the knockoff distribution requires solving a large-scale semidefinite program for which we derive several efficient methods. One handles generic covariance matrices and has a complexity scaling as O(p(3)), where p is the ambient dimension, while another assumes a rank-k factor model on the covariance matrix to reduce this complexity bound to O(pk(2)). We review an efficient procedure to estimate factor models and show that under a factor model assumption, we can sample knockoff covariates with complexity linear in the dimension. We test our methods on problems with p as large as 500 000.
更多查看译文
关键词
convex optimization, semidefinite programming, coordinate descent, false discovery rate
AI 理解论文
溯源树
样例
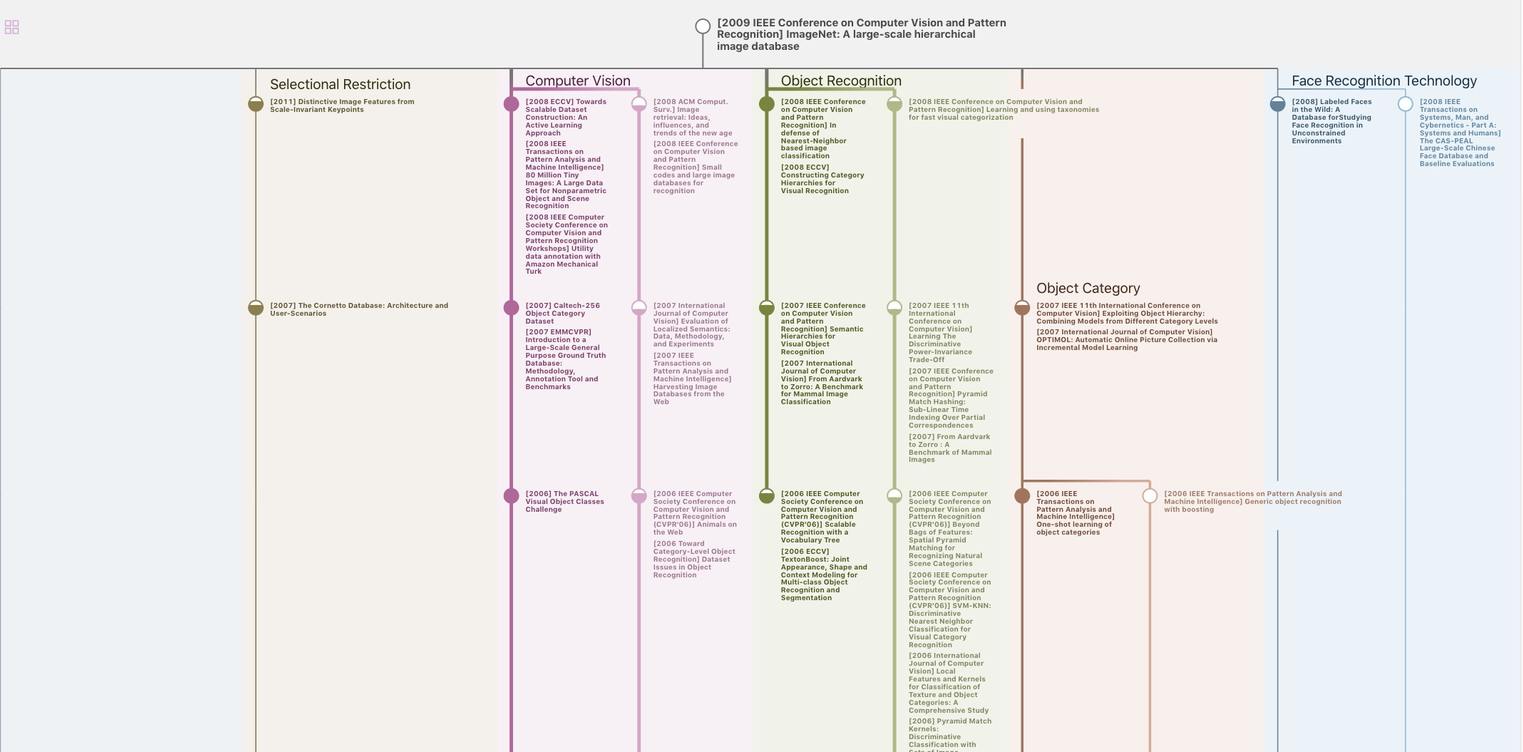
生成溯源树,研究论文发展脉络
Chat Paper
正在生成论文摘要