Feature Space Saturation during Training
arxiv(2020)
摘要
We propose layer saturation - a simple, online-computable method for analyzing the information processing in neural networks. First, we show that a layer's output can be restricted to the eigenspace of its variance matrix without performance loss. We propose a computationally lightweight method for approximating the variance matrix during training. From the dimension of its lossless eigenspace we derive \textit{layer saturation} - the ratio between the eigenspace dimension and layer width. We show that saturation seems to indicate which layers contribute to network performance. We demonstrate how to alter layer saturation in a neural network by changing network depth, filter sizes and input resolution. Furthermore, we show that well-chosen input resolution increases network performance by distributing the inference process more evenly across the network.
更多查看译文
关键词
training,saturation,feature,space
AI 理解论文
溯源树
样例
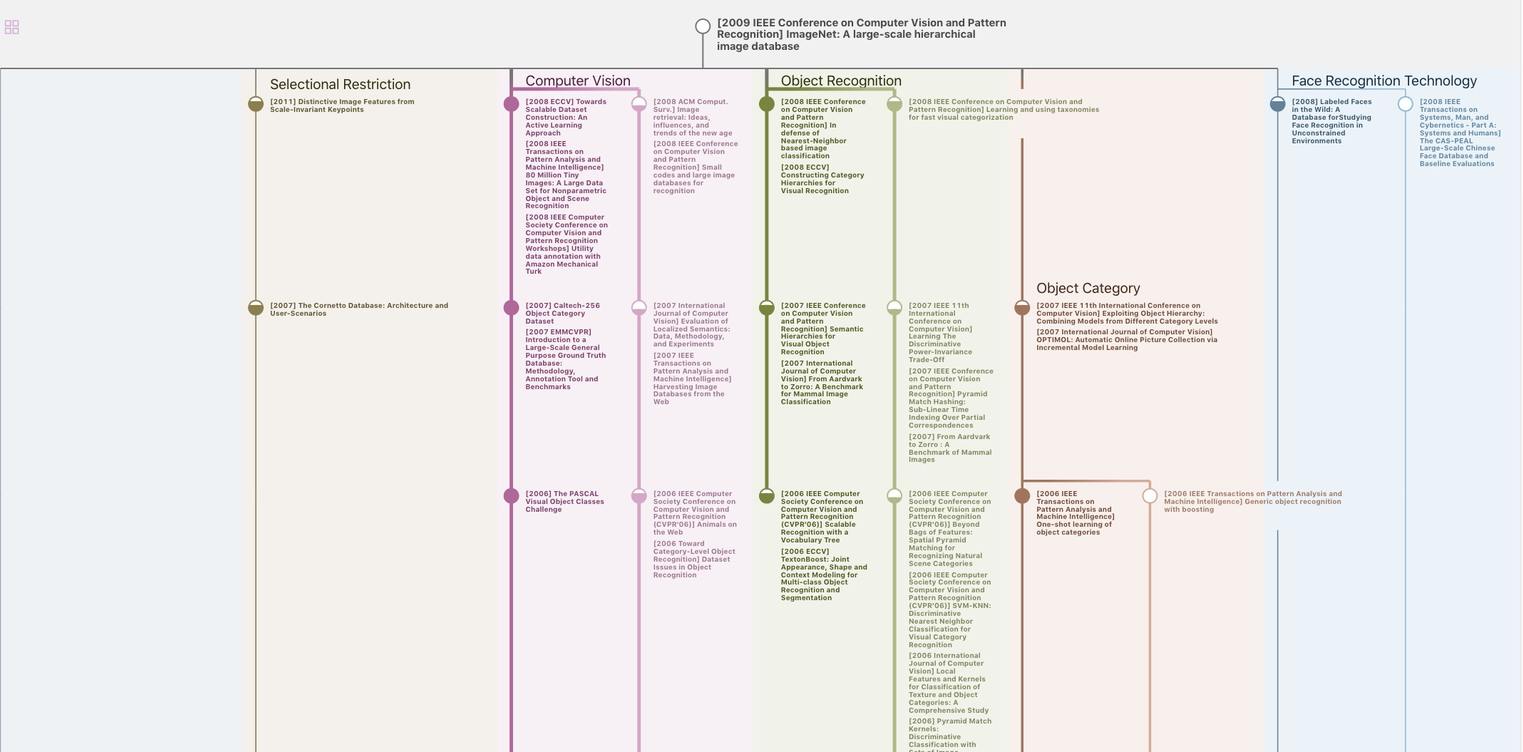
生成溯源树,研究论文发展脉络
Chat Paper
正在生成论文摘要