COT-GAN: Generating Sequential Data via Causal Optimal Transport
NIPS 2020(2020)
摘要
We introduce COT-GAN, an adversarial algorithm to train implicit generative models optimized for producing sequential data. The loss function of this algorithm is formulated using ideas from Causal Optimal Transport (COT), which combines classic optimal transport methods with an additional temporal causality constraint. Remarkably, we find that this causality condition provides a natural framework to parameterize the cost function that is learned by the discriminator as a robust (worst-case) distance, and an ideal mechanism for learning time dependent data distributions. Following Genevay et al.\ (2018), we also include an entropic penalization term which allows for the use of the Sinkhorn algorithm when computing the optimal transport cost. Our experiments show effectiveness and stability of COT-GAN when generating both low- and high-dimensional time series data. The success of the algorithm also relies on a new, improved version of the Sinkhorn divergence which demonstrates less bias in learning.
更多查看译文
关键词
causal optimal transport,sequential data,cot-gan
AI 理解论文
溯源树
样例
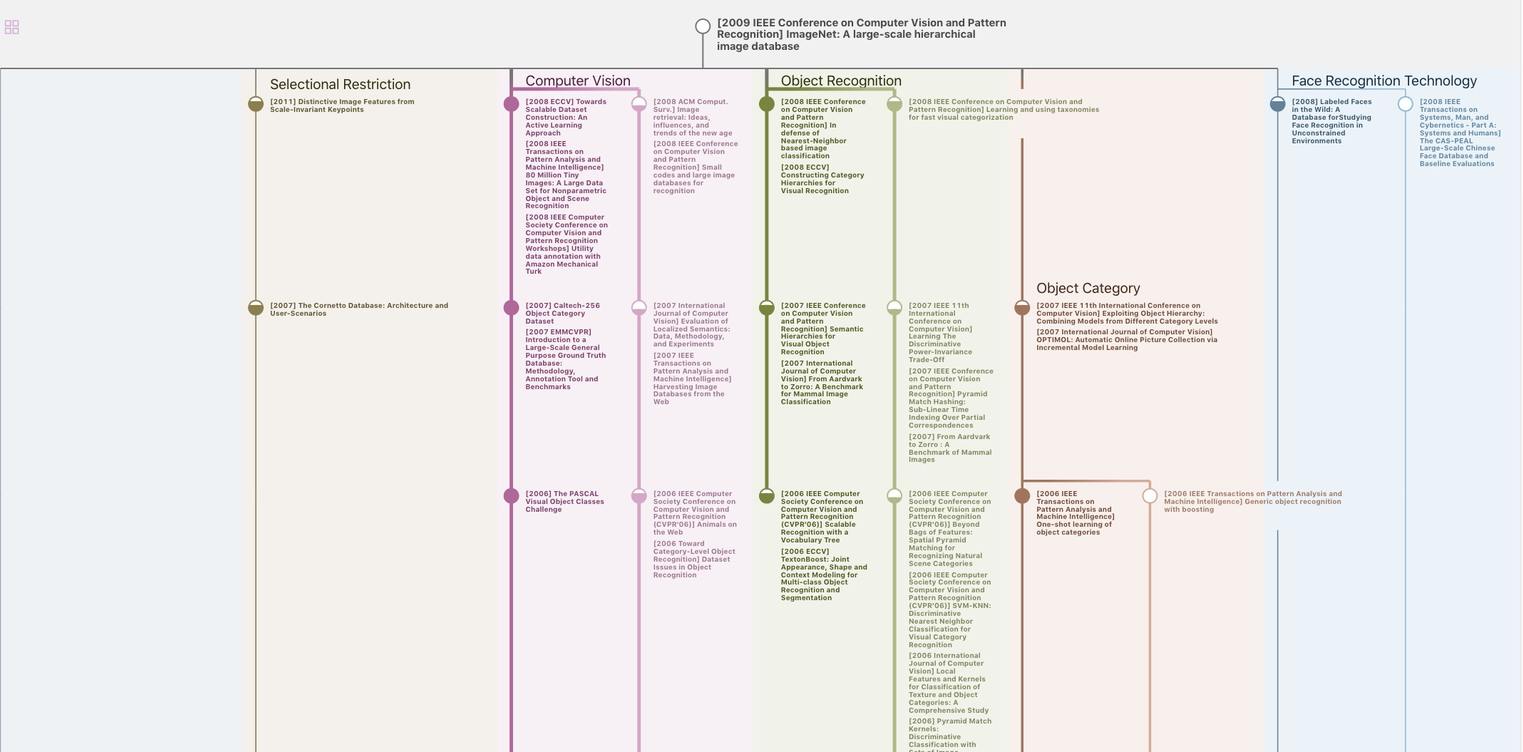
生成溯源树,研究论文发展脉络
Chat Paper
正在生成论文摘要