An Unsupervised Machine Learning Approach to Assess the ZIP Code Level Impact of COVID-19 in NYC
ACS Nano(2020)
摘要
New York City has been recognized as the world's epicenter of the novel Coronavirus pandemic. To identify the key inherent factors that are highly correlated to the Increase Rate of COVID-19 new cases in NYC, we propose an unsupervised machine learning framework. Based on the assumption that ZIP code areas with similar demographic, socioeconomic, and mobility patterns are likely to experience similar outbreaks, we select the most relevant features to perform a clustering that can best reflect the spread, and map them down to 9 interpretable categories. We believe that our findings can guide policy makers to promptly anticipate and prevent the spread of the virus by taking the right measures.
更多查看译文
关键词
zip code level impact,unsupervised machine learning approach,machine learning
AI 理解论文
溯源树
样例
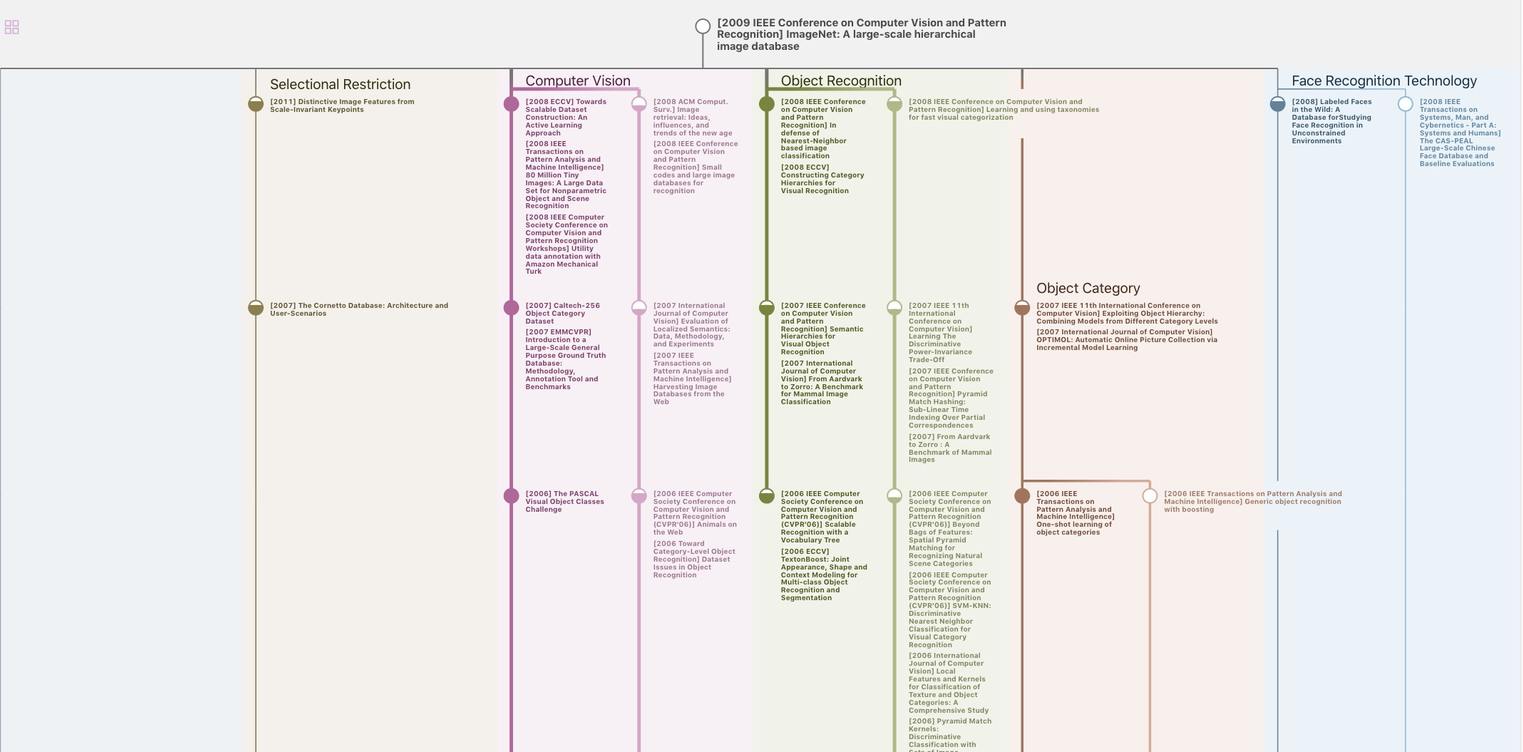
生成溯源树,研究论文发展脉络
Chat Paper
正在生成论文摘要