Improved complexities for stochastic conditional gradient methods under interpolation-like conditions
Operations Research Letters(2022)
摘要
We analyze stochastic conditional gradient methods for constrained optimization problems arising in over-parametrized machine learning. We show that one could leverage the interpolation-like conditions satisfied by such models to obtain improved oracle complexities. Specifically, when the objective function is convex, we show that the conditional gradient method requires O(ϵ−2) calls to the stochastic gradient oracle to find an ϵ-optimal solution. Furthermore, by including a gradient sliding step, we show that the number of calls reduces to O(ϵ−1.5).
更多查看译文
关键词
Stochastic conditional gradient,Oracle complexity,Overparametrization,Zeroth-order optimization
AI 理解论文
溯源树
样例
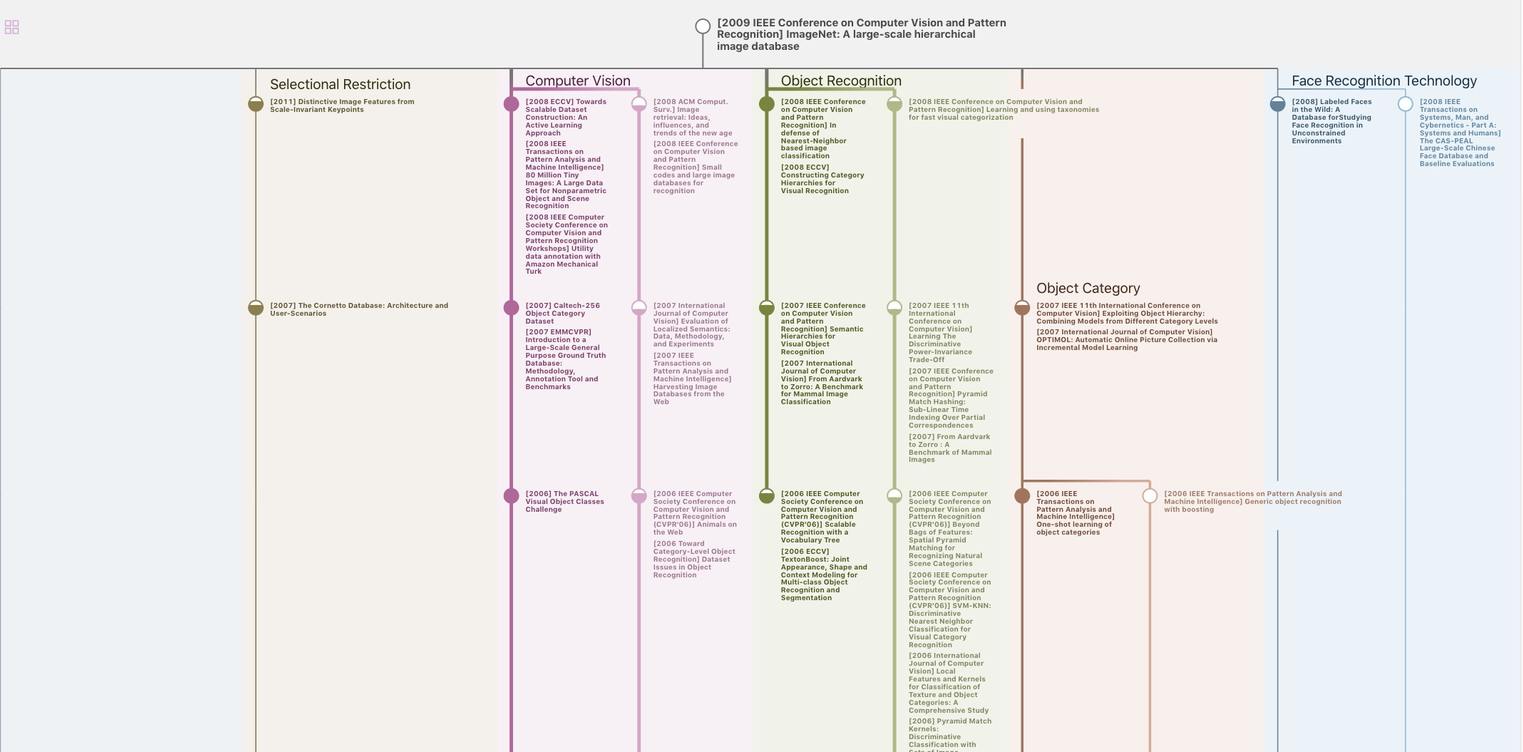
生成溯源树,研究论文发展脉络
Chat Paper
正在生成论文摘要