Low-rank tensor methods for Markov chains with applications to tumor progression models
Journal of Mathematical Biology(2022)
摘要
Cancer progression can be described by continuous-time Markov chains whose state space grows exponentially in the number of somatic mutations. The age of a tumor at diagnosis is typically unknown. Therefore, the quantity of interest is the time-marginal distribution over all possible genotypes of tumors, defined as the transient distribution integrated over an exponentially distributed observation time. It can be obtained as the solution of a large linear system. However, the sheer size of this system renders classical solvers infeasible. We consider Markov chains whose transition rates are separable functions, allowing for an efficient low-rank tensor representation of the linear system’s operator. Thus we can reduce the computational complexity from exponential to linear. We derive a convergent iterative method using low-rank formats whose result satisfies the normalization constraint of a distribution. We also perform numerical experiments illustrating that the marginal distribution is well approximated with low rank.
更多查看译文
关键词
Transient distribution, Stochastic Automata Networks, Mutual Hazard Networks, Hierarchical Tucker format, 15A69, 60J22, 60J28
AI 理解论文
溯源树
样例
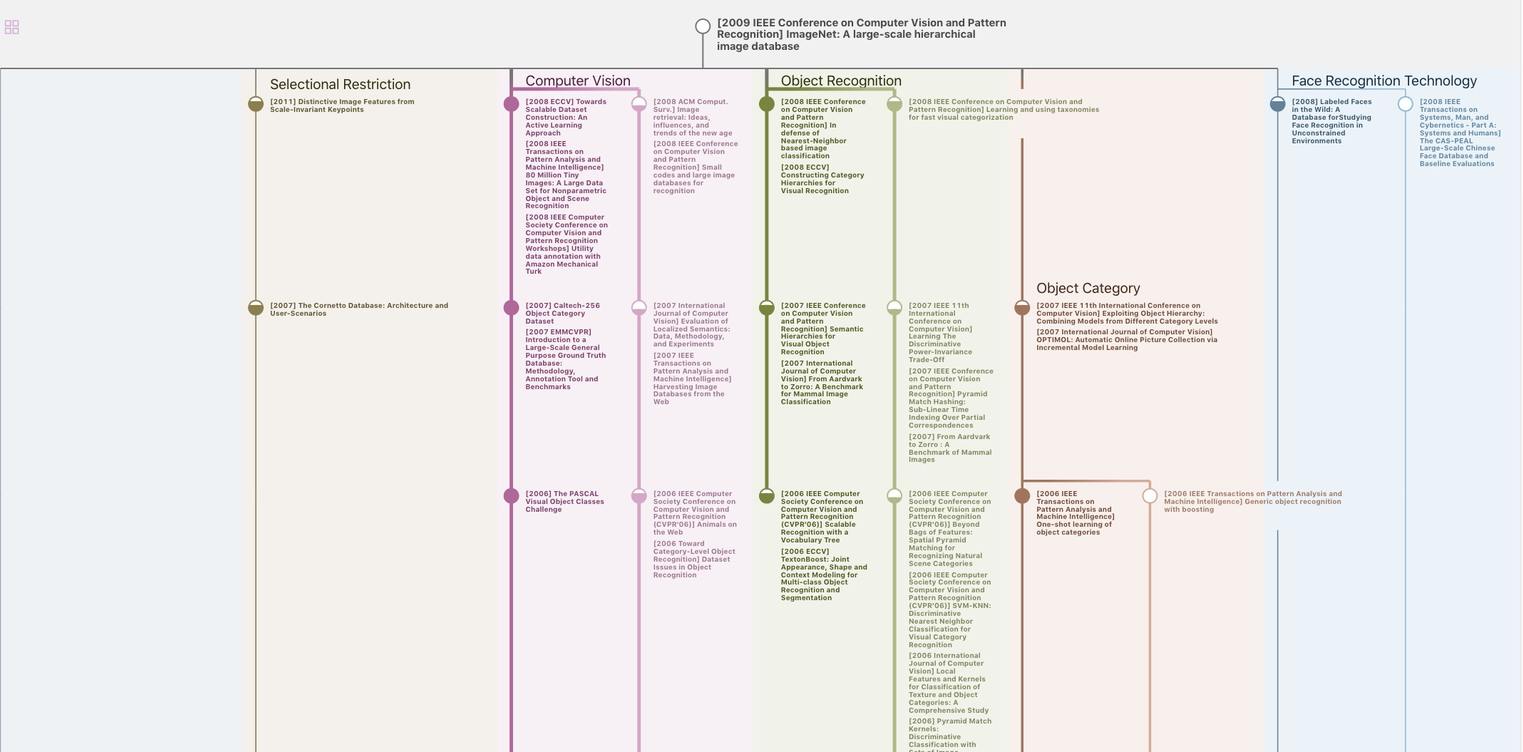
生成溯源树,研究论文发展脉络
Chat Paper
正在生成论文摘要