Team RUC_AIM3 Technical Report at Activitynet 2020 Task 2: Exploring Sequential Events Detection for Dense Video Captioning
arxiv(2020)
摘要
Detecting meaningful events in an untrimmed video is essential for dense video captioning. In this work, we propose a novel and simple model for event sequence generation and explore temporal relationships of the event sequence in the video. The proposed model omits inefficient two-stage proposal generation and directly generates event boundaries conditioned on bi-directional temporal dependency in one pass. Experimental results show that the proposed event sequence generation model can generate more accurate and diverse events within a small number of proposals. For the event captioning, we follow our previous work to employ the intra-event captioning models into our pipeline system. The overall system achieves state-of-the-art performance on the dense-captioning events in video task with 9.894 METEOR score on the challenge testing set.
更多查看译文
关键词
sequential events detection,activitynet,video
AI 理解论文
溯源树
样例
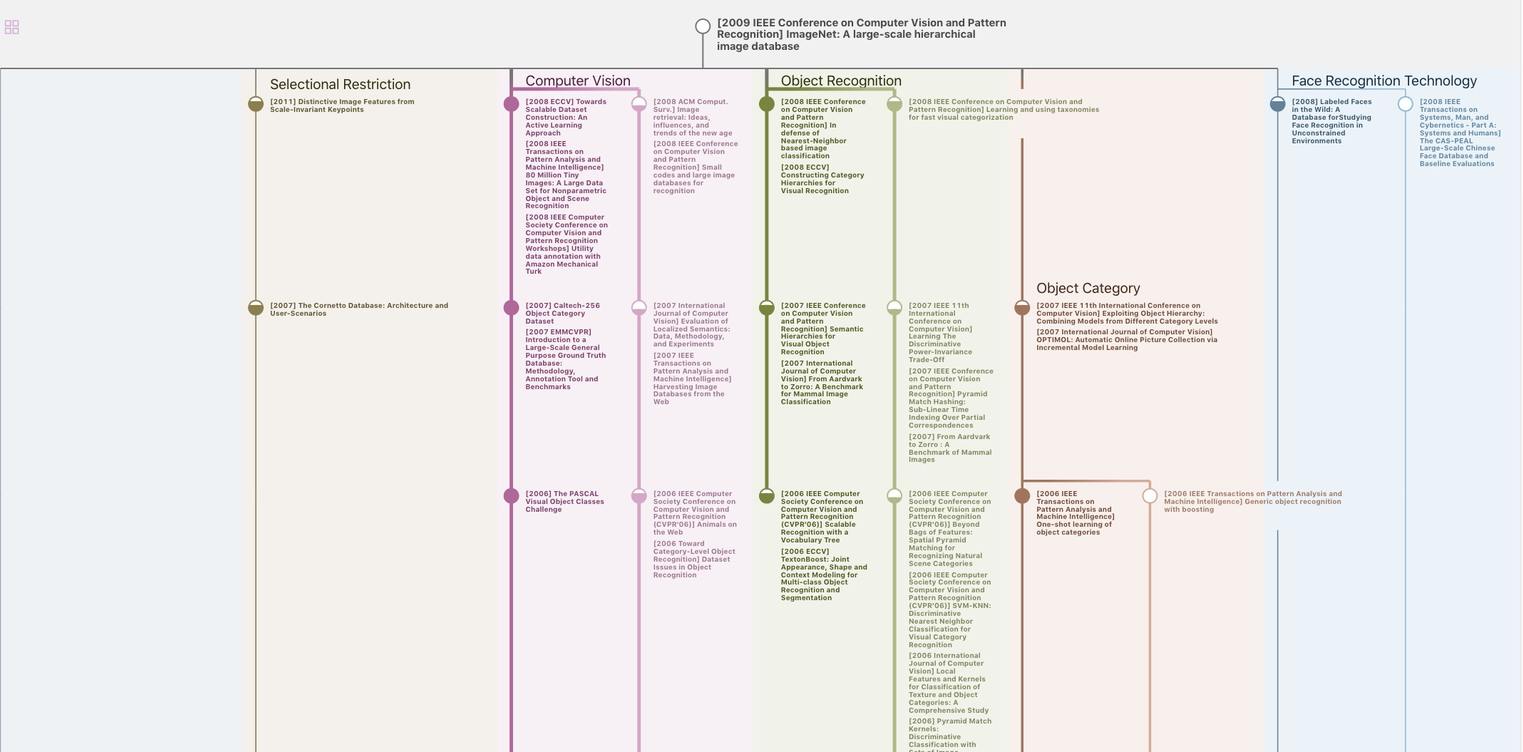
生成溯源树,研究论文发展脉络
Chat Paper
正在生成论文摘要