Active Imitation Learning from Multiple Non-Deterministic Teachers: Formulation, Challenges, and Algorithms
arxiv(2020)
摘要
We formulate the problem of learning to imitate multiple, non-deterministic teachers with minimal interaction cost. Rather than learning a specific policy as in standard imitation learning, the goal in this problem is to learn a distribution over a policy space. We first present a general framework that efficiently models and estimates such a distribution by learning continuous representations of the teacher policies. Next, we develop Active Performance-Based Imitation Learning (APIL), an active learning algorithm for reducing the learner-teacher interaction cost in this framework. By making query decisions based on predictions of future progress, our algorithm avoids the pitfalls of traditional uncertainty-based approaches in the face of teacher behavioral uncertainty. Results on both toy and photo-realistic navigation tasks show that APIL significantly reduces the numbers of interactions with teachers without compromising on performance. Moreover, it is robust to various degrees of teacher behavioral uncertainty.
更多查看译文
关键词
active imitation learning,teachers,non-deterministic
AI 理解论文
溯源树
样例
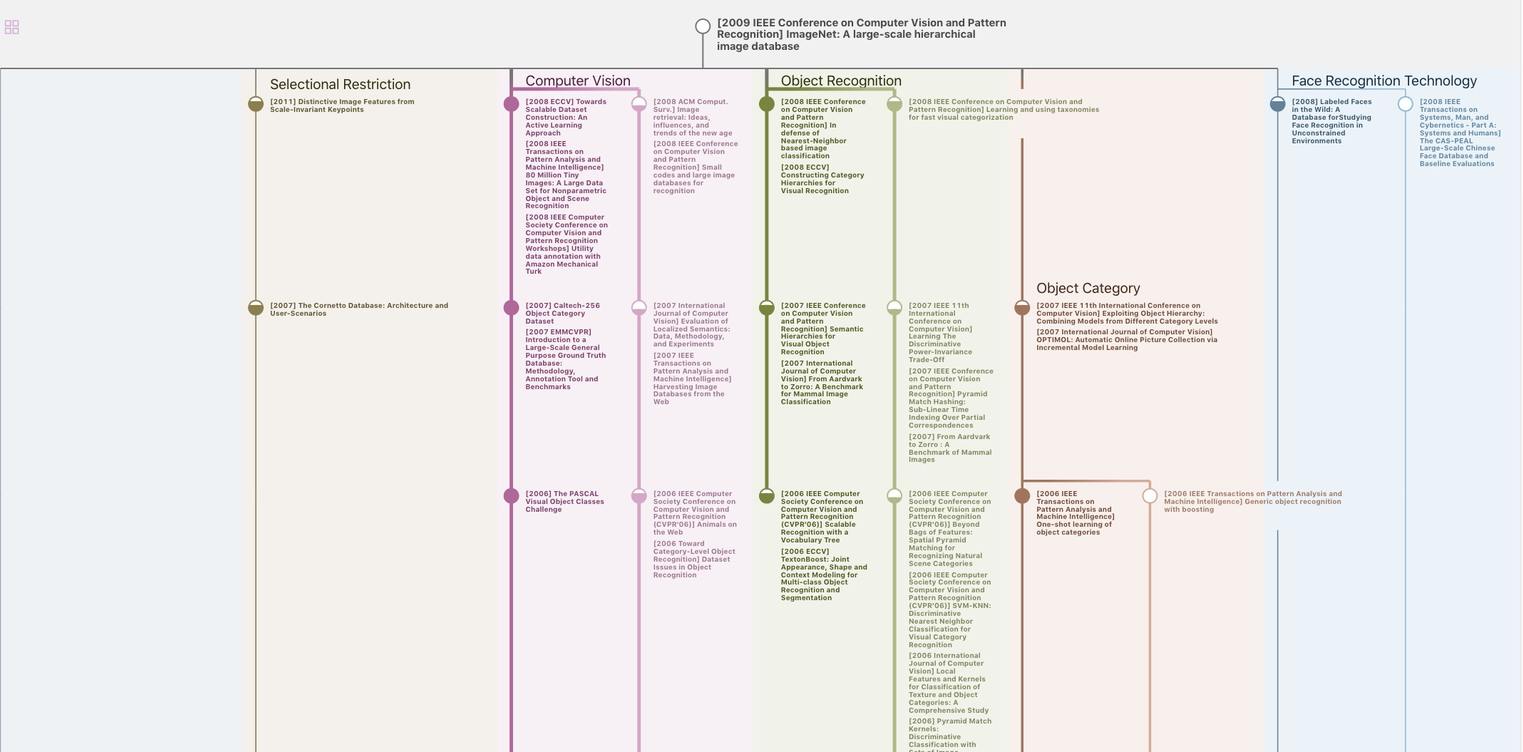
生成溯源树,研究论文发展脉络
Chat Paper
正在生成论文摘要