Faster MCMC for Gaussian latent position network models
NETWORK SCIENCE(2022)
摘要
Latent position network models are a versatile tool in network science; applications include clustering entities, controlling for causal confounders, and defining priors over unobserved graphs. Estimating each node's latent position is typically framed as a Bayesian inference problem, with Metropolis within Gibbs being the most popular tool for approximating the posterior distribution. However, it is well-known that Metropolis within Gibbs is inefficient for large networks; the acceptance ratios are expensive to compute, and the resultant posterior draws are highly correlated. In this article, we propose an alternative Markov chain Monte Carlo strategy-defined using a combination of split Hamiltonian Monte Carlo and Firefly Monte Carlo-that leverages the posterior distribution's functional form for more efficient posterior computation. We demonstrate that these strategies outperform Metropolis within Gibbs and other algorithms on synthetic networks, as well as on real information-sharing networks of teachers and staff in a school district.
更多查看译文
关键词
Hamiltonian Monte Carlo, network data, firefly Monte Carlo, latent space model, longitudinal network data, Bayesian computation
AI 理解论文
溯源树
样例
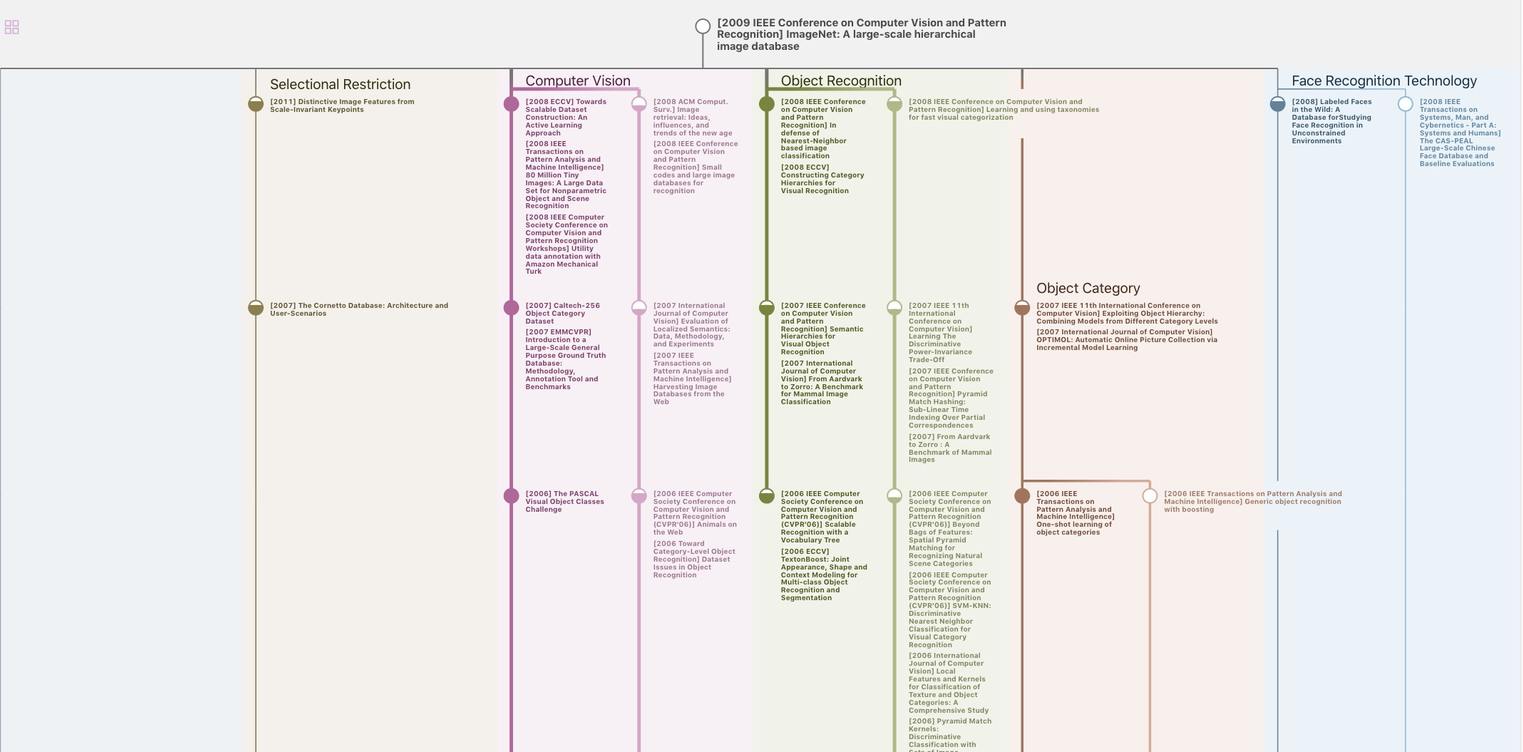
生成溯源树,研究论文发展脉络
Chat Paper
正在生成论文摘要