Guided Transformer: Leveraging Multiple External Sources for Representation Learning in Conversational Search
SIGIR '20: The 43rd International ACM SIGIR conference on research and development in Information Retrieval Virtual Event China July, 2020(2020)
摘要
Asking clarifying questions in response to ambiguous or faceted queries has been recognized as a useful technique for various information retrieval systems, especially conversational search systems with limited bandwidth interfaces. Analyzing and generating clarifying questions have been studied recently but the accurate utilization of user responses to clarifying questions has been relatively less explored. In this paper, we enrich the representations learned by Transformer networks using a novel attention mechanism from external information sources that weights each term in the conversation. We evaluate this Guided Transformer model in a conversational search scenario that includes clarifying questions. In our experiments, we use two separate external sources, including the top retrieved documents and a set of different possible clarifying questions for the query. We implement the proposed representation learning model for two downstream tasks in conversational search; document retrieval and next clarifying question selection. Our experiments use a public dataset for search clarification and demonstrate significant improvements compared to competitive baselines.
更多查看译文
关键词
representation learning,multiple external sources,search
AI 理解论文
溯源树
样例
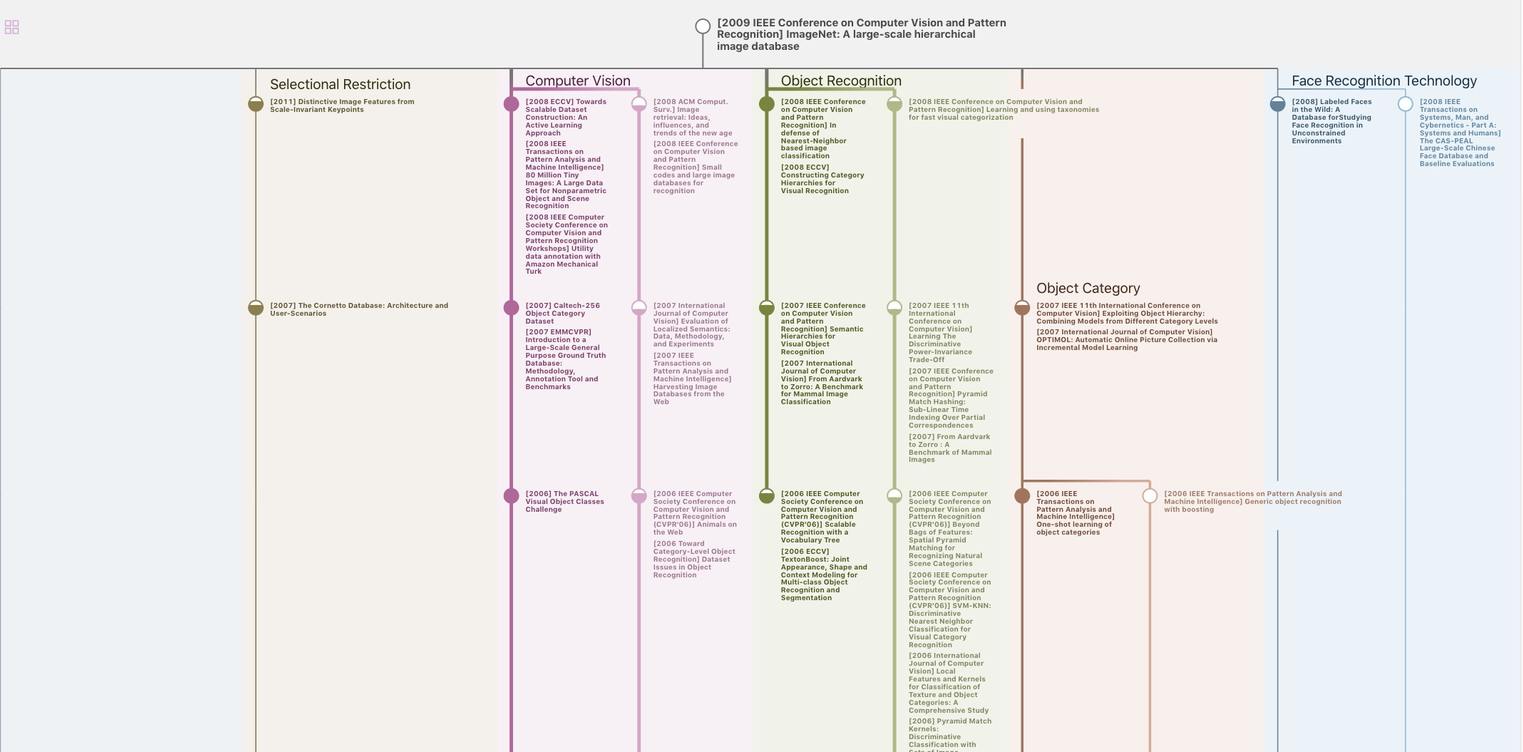
生成溯源树,研究论文发展脉络
Chat Paper
正在生成论文摘要