How to Avoid Being Eaten by a Grue: Structured Exploration Strategies for Textual Worlds
arxiv(2020)
摘要
Text-based games are long puzzles or quests, characterized by a sequence of sparse and potentially deceptive rewards. They provide an ideal platform to develop agents that perceive and act upon the world using a combinatorially sized natural language state-action space. Standard Reinforcement Learning agents are poorly equipped to effectively explore such spaces and often struggle to overcome bottlenecks---states that agents are unable to pass through simply because they do not see the right action sequence enough times to be sufficiently reinforced. We introduce Q*BERT, an agent that learns to build a knowledge graph of the world by answering questions, which leads to greater sample efficiency. To overcome bottlenecks, we further introduce MC!Q*BERT an agent that uses an knowledge-graph-based intrinsic motivation to detect bottlenecks and a novel exploration strategy to efficiently learn a chain of policy modules to overcome them. We present an ablation study and results demonstrating how our method outperforms the current state-of-the-art on nine text games, including the popular game, Zork, where, for the first time, a learning agent gets past the bottleneck where the player is eaten by a Grue.
更多查看译文
AI 理解论文
溯源树
样例
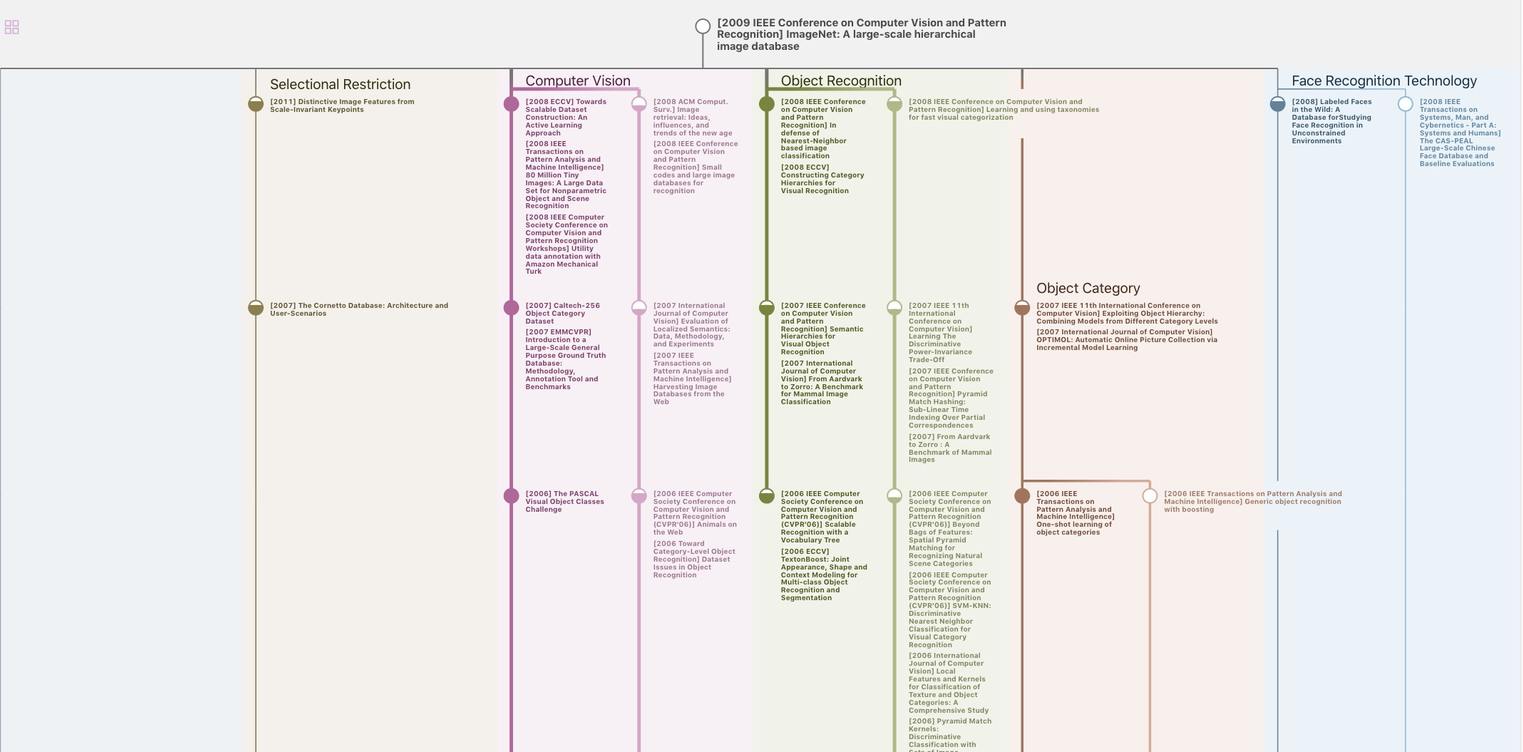
生成溯源树,研究论文发展脉络
Chat Paper
正在生成论文摘要