Testing swampland conjectures with machine learning
The European Physical Journal C(2020)
摘要
We consider Type IIB compactifications on an isotropic torus T^6 threaded by geometric and non geometric fluxes. For this particular setup we apply supervised machine learning techniques, namely an artificial neural network coupled to a genetic algorithm, in order to obtain more than sixty thousand flux configurations yielding to a scalar potential with at least one critical point. We observe that both stable AdS vacua with large moduli masses and small vacuum energy as well as unstable dS vacua with small tachyonic mass and large energy are absent, in accordance to the refined de Sitter conjecture. Moreover, by considering a hierarchy among fluxes, we observe that perturbative solutions with small values for the vacuum energy and moduli masses are favored, as well as scenarios in which the lightest modulus mass is much smaller than the corresponding AdS vacuum scale. Finally we apply some results on random matrix theory to conclude that the most probable mass spectrum derived from this string setup is that satisfying the Refined de Sitter and AdS scale conjectures.
更多查看译文
关键词
swampland,machine learning,testing
AI 理解论文
溯源树
样例
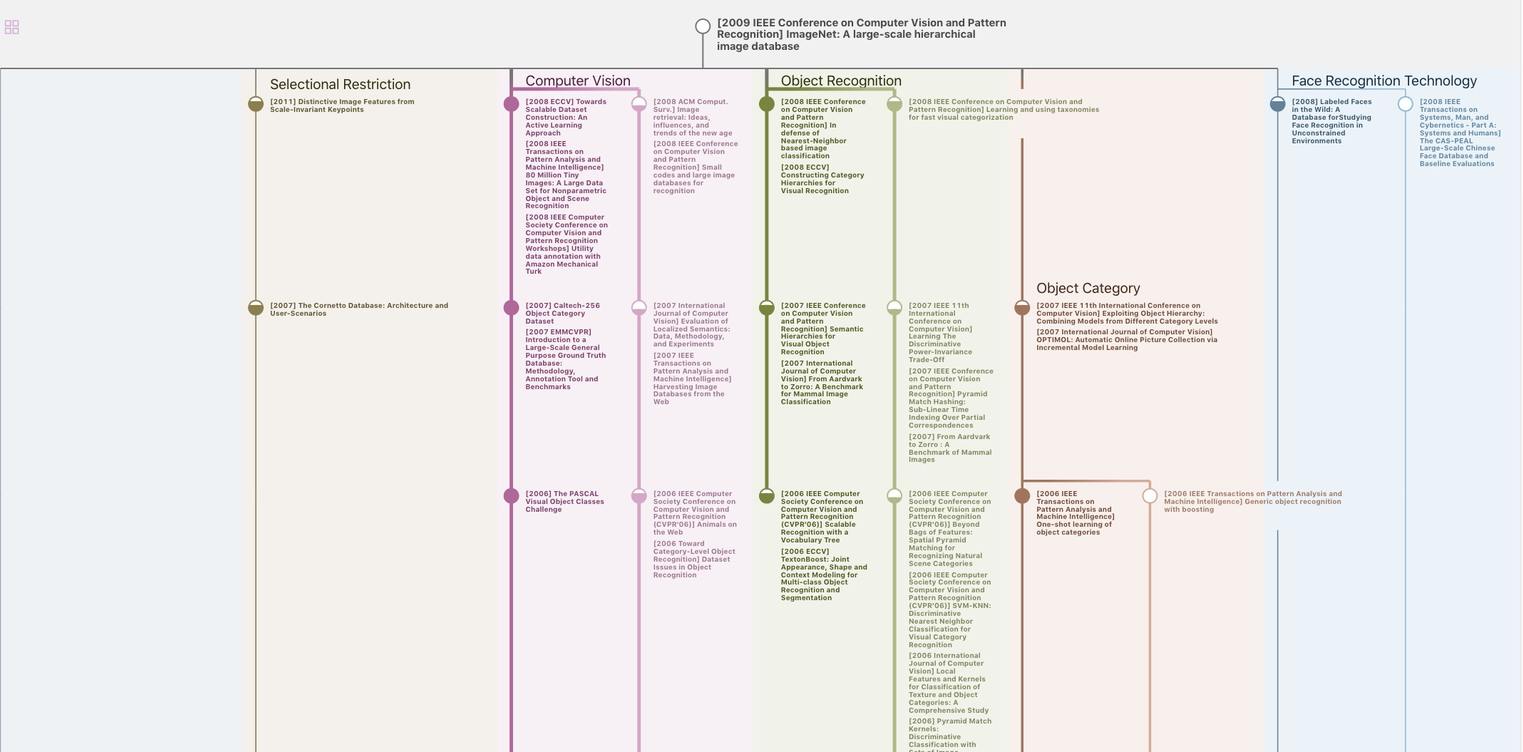
生成溯源树,研究论文发展脉络
Chat Paper
正在生成论文摘要