Fast Objective & Duality Gap Convergence for Non-Convex Strongly-Concave Min-Max Problems with PL Condition
JOURNAL OF MACHINE LEARNING RESEARCH(2023)
摘要
This paper focuses on stochastic methods for solving smooth non-convex strongly-concave min-max problems, which have received increasing attention due to their potential applica-tions in deep learning (e.g., deep AUC maximization, distributionally robust optimization). However, most of the existing algorithms are slow in practice, and their analysis revolves around the convergence to a nearly stationary point. We consider leveraging the Polyak-Lojasiewicz (PL) condition to design faster stochastic algorithms with stronger convergence guarantee. Although PL condition has been utilized for designing many stochastic mini-mization algorithms, their applications for non-convex min-max optimization remain rare. In this paper, we propose and analyze a generic framework of proximal stage-based method with many well-known stochastic updates embeddable. Fast convergence is established in terms of both the primal objective gap and the duality gap. Compared with existing studies, (i) our analysis is based on a novel Lyapunov function consisting of the primal objective gap and the duality gap of a regularized function, and (ii) the results are more comprehensive with improved rates that have better dependence on the condition number under different assumptions. We also conduct deep and non-deep learning experiments to verify the effectiveness of our methods.
更多查看译文
关键词
Min-Max Problems,Non-Convex Optimization,Stochastic Optimization,PL Condition,Proximal Stage-Based Method
AI 理解论文
溯源树
样例
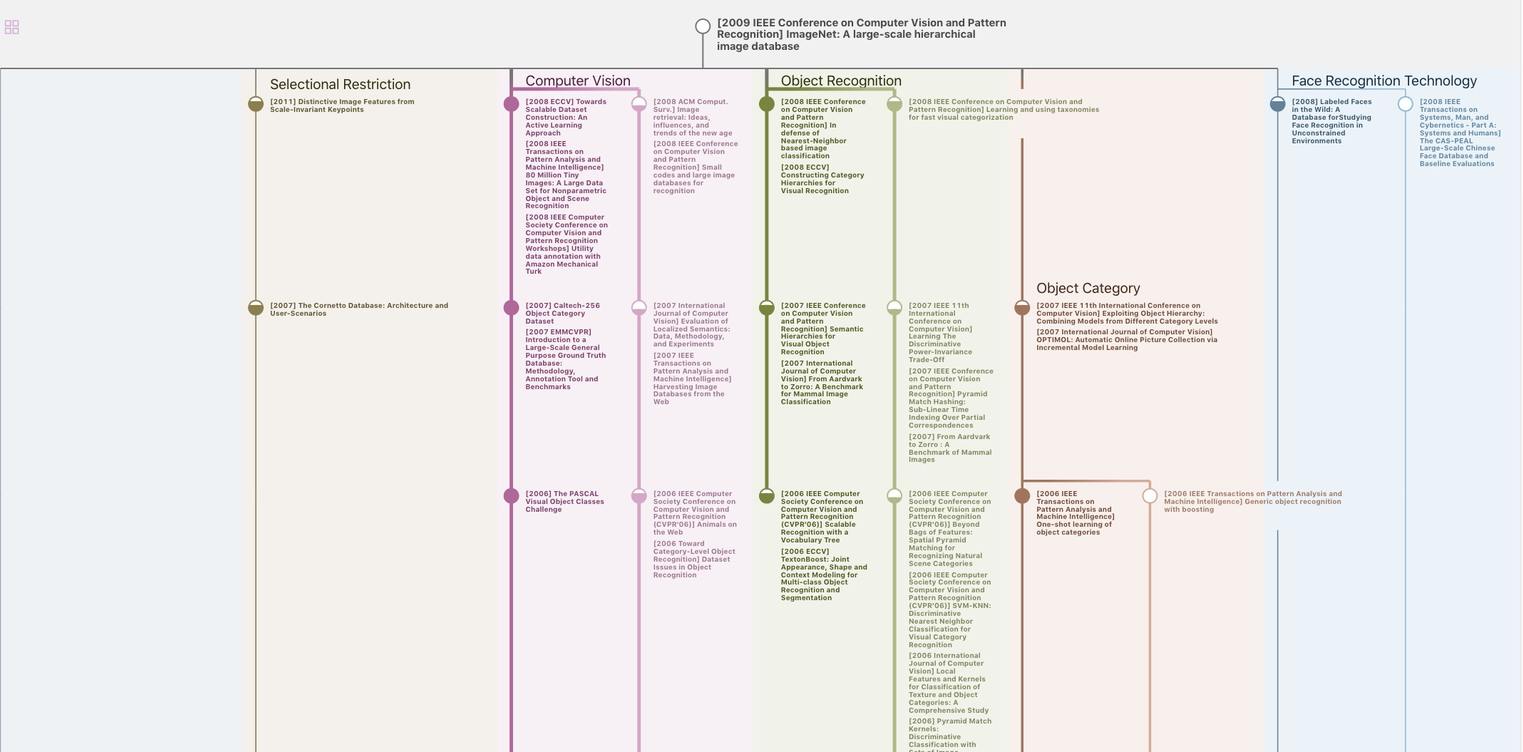
生成溯源树,研究论文发展脉络
Chat Paper
正在生成论文摘要