Deep Adversarial Koopman Model for Reaction-Diffusion systems
arxiv(2020)
摘要
Reaction-diffusion systems are ubiquitous in nature and in engineering applications, and are often modeled using a non-linear system of governing equations. While robust numerical methods exist to solve them, deep learning-based reduced ordermodels (ROMs) are gaining traction as they use linearized dynamical models to advance the solution in time. One such family of algorithms is based on Koopman theory, and this paper applies this numerical simulation strategy to reaction-diffusion systems. Adversarial and gradient losses are introduced, and are found to robustify the predictions. The proposed model is extended to handle missing training data as well as recasting the problem from a control perspective. The efficacy of these developments are demonstrated for two different reaction-diffusion problems: (1) the Kuramoto-Sivashinsky equation of chaos and (2) the Turing instability using the Gray-Scott model.
更多查看译文
关键词
model,reaction-diffusion
AI 理解论文
溯源树
样例
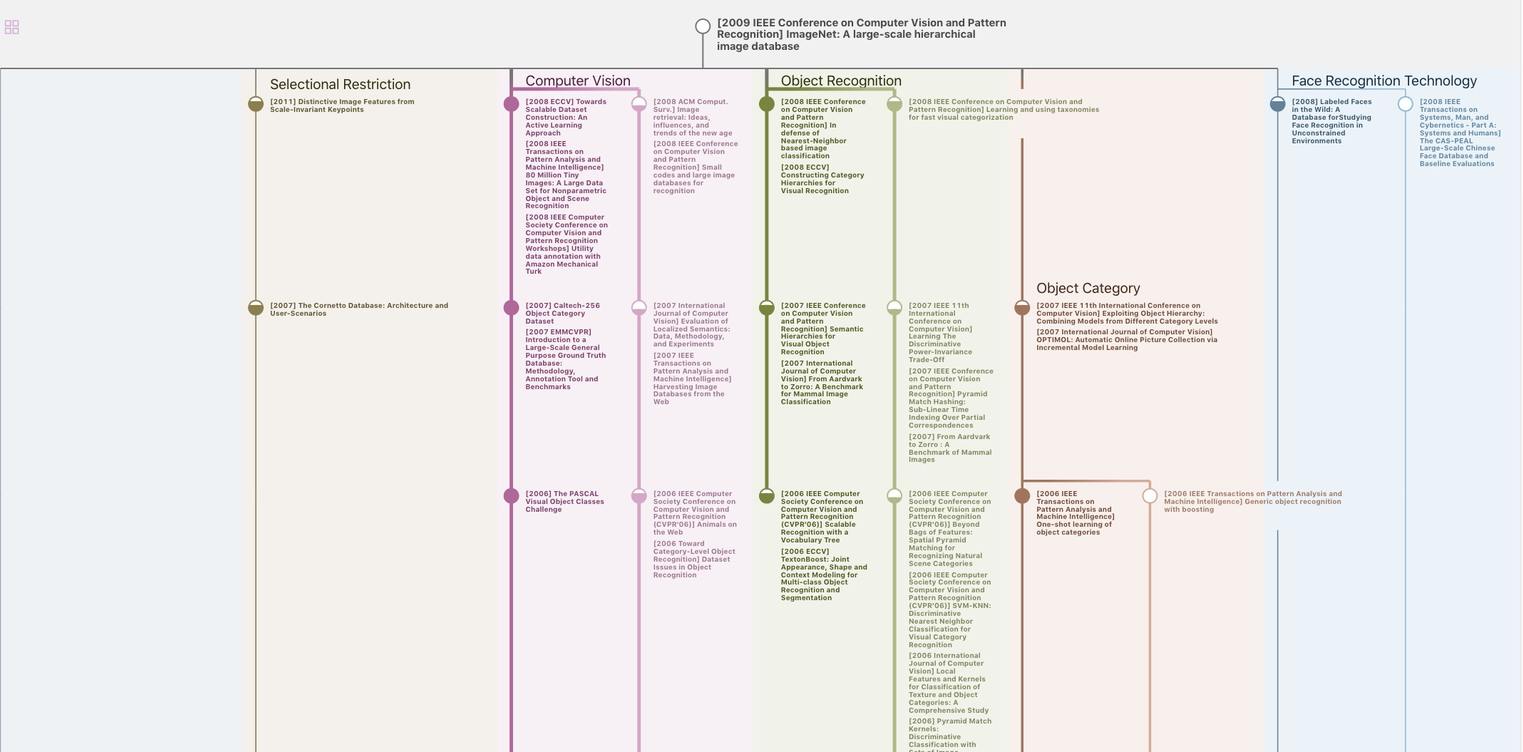
生成溯源树,研究论文发展脉络
Chat Paper
正在生成论文摘要