Confnet2seq Full Length Answer Generation From Spoken Questions
TEXT, SPEECH, AND DIALOGUE (TSD 2020)(2020)
摘要
Conversational and task-oriented dialogue systems aim to interact with the user using natural responses through multi-modal interfaces, such as text or speech. These desired responses are in the form of full-length natural answers generated over facts retrieved from a knowledge source. While the task of generating natural answers to questions from an answer span has been widely studied, there has been little research on natural sentence generation over spoken content. We propose a novel system to generate full length natural language answers from spoken questions and factoid answers. The spoken sequence is compactly represented as a confusion network extracted from a pre-trained Automatic Speech Recognizer. This is the first attempt towards generating full-length natural answers from a graph input (confusion network) to the best of our knowledge. We release a large-scale dataset of 259,788 samples of spoken questions, their factoid answers and corresponding full-length textual answers. Following our proposed approach, we achieve comparable performance with best ASR hypothesis.
更多查看译文
关键词
Confusion network, Pointer-generator, Copy attention, Natural answer generation, Question answering
AI 理解论文
溯源树
样例
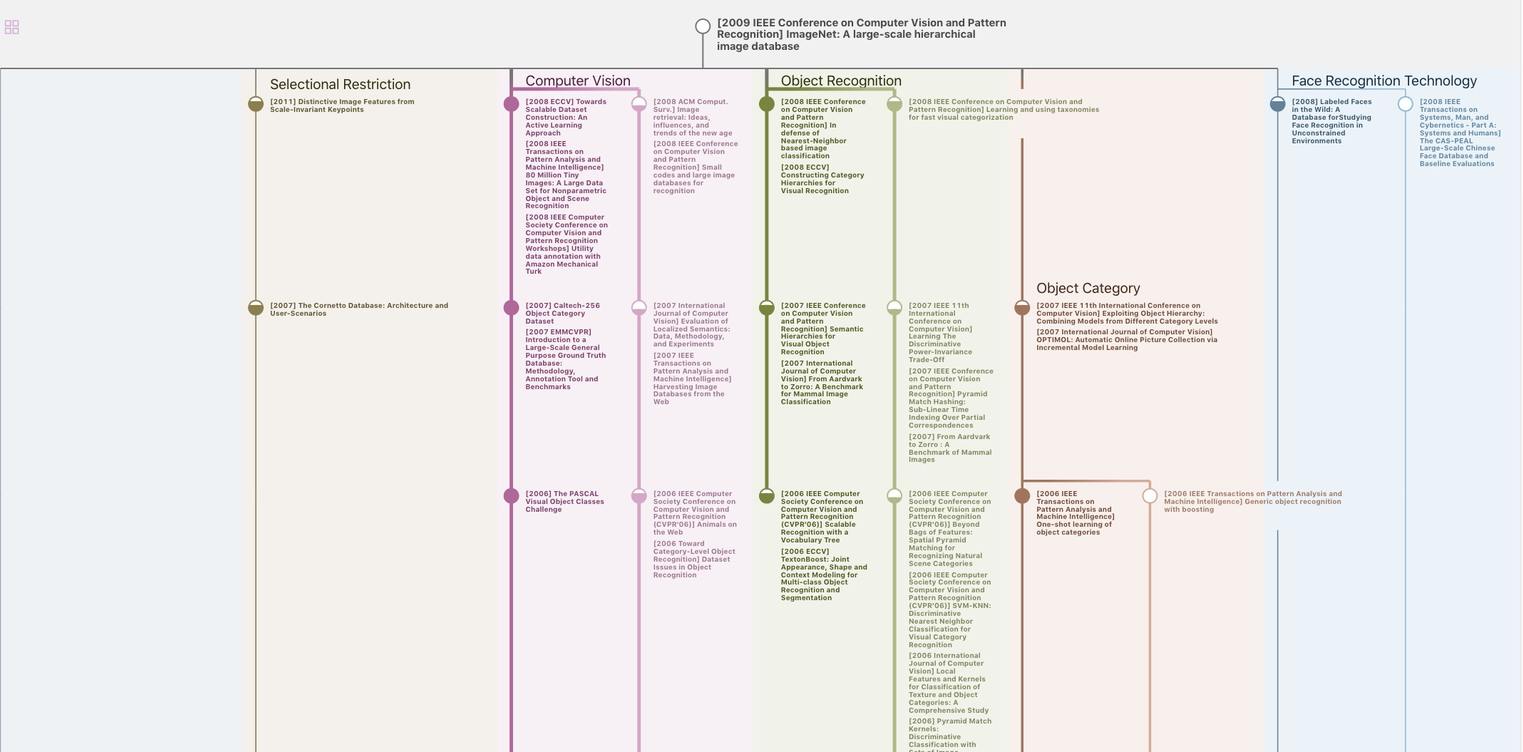
生成溯源树,研究论文发展脉络
Chat Paper
正在生成论文摘要