Stochastic matrix games with bandit feedback
arxiv(2020)
摘要
We study a version of the classical zero-sum matrix game with unknown payoff matrix and bandit feedback, where the players only observe each others actions and a noisy payoff. This generalizes the usual matrix game, where the payoff matrix is known to the players. Despite numerous applications, this problem has received relatively little attention. Although adversarial bandit algorithms achieve low regret, they do not exploit the matrix structure and perform poorly relative to the new algorithms. The main contributions are regret analyses of variants of UCB and K-learning that hold for any opponent, e.g., even when the opponent adversarially plays the best response to the learner's mixed strategy. Along the way, we show that Thompson fails catastrophically in this setting and provide empirical comparison to existing algorithms.
更多查看译文
关键词
stochastic matrix games,bandit feedback
AI 理解论文
溯源树
样例
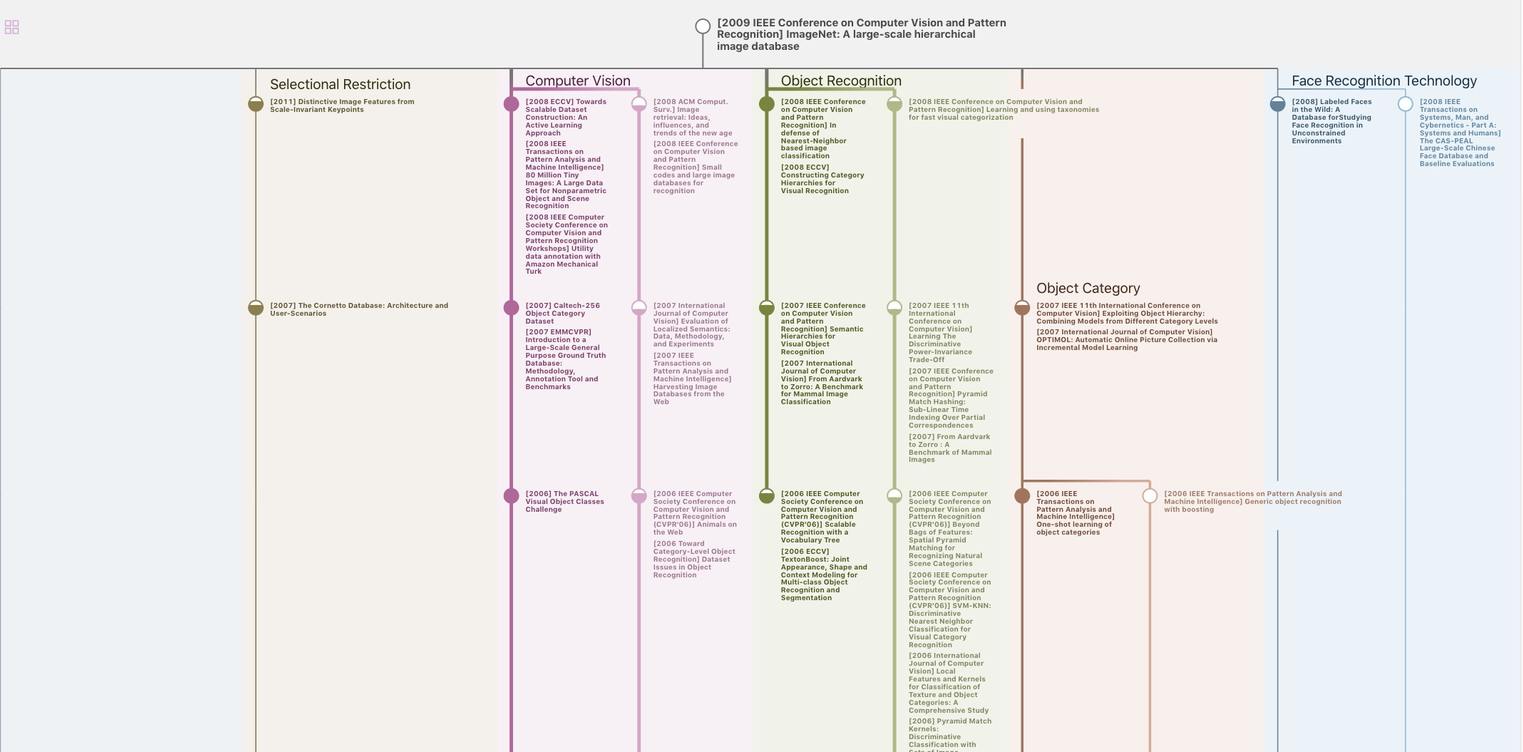
生成溯源树,研究论文发展脉络
Chat Paper
正在生成论文摘要