Robust estimation of the effect of an exposure on the change in a continuous outcome
BMC Medical Research Methodology(2020)
摘要
Background The change in two measurements of a continuous outcome can be modelled directly with a linear regression model, or indirectly with a random effects model (REM) of the individual measurements. These methods are susceptible to model misspecifications, which are commonly addressed by applying monotonic transformations (e.g., Box-Cox transformation) to the outcomes. However, transforming the outcomes complicates the data analysis, especially when variable selection is involved. We propose a robust alternative through a novel application of the conditional probit (cprobit) model. Methods The cprobit model analyzes the ordered outcomes within each subject, making the estimate invariant to monotonic transformation on the outcome. By scaling the estimate from the cprobit model, we obtain the exposure effect on the change in the observed or Box-Cox transformed outcome, pending the adequacy of the normality assumption on the raw or transformed scale. Results Using simulated data, we demonstrated a similar good performance of the cprobit model and REM with and without transformation, except for some bias from both methods when the Box-Cox transformation was applied to scenarios with small sample size and strong effects. Only the cprobit model was robust to skewed subject-specific intercept terms when a Box-Cox transformation was used. Using two real datasets from the breast cancer and inpatient glycemic variability studies which utilize electronic medical records, we illustrated the application of our proposed robust approach as a seamless three-step workflow that facilitates the use of Box-Cox transformation to address non-normality with a common underlying model. Conclusions The cprobit model provides a seamless and robust inference on the change in continuous outcomes, and its three-step workflow is implemented in an R package for easy accessibility.
更多查看译文
关键词
Box-Cox transformation,Conditional probit model,Normal errors,Random effects model
AI 理解论文
溯源树
样例
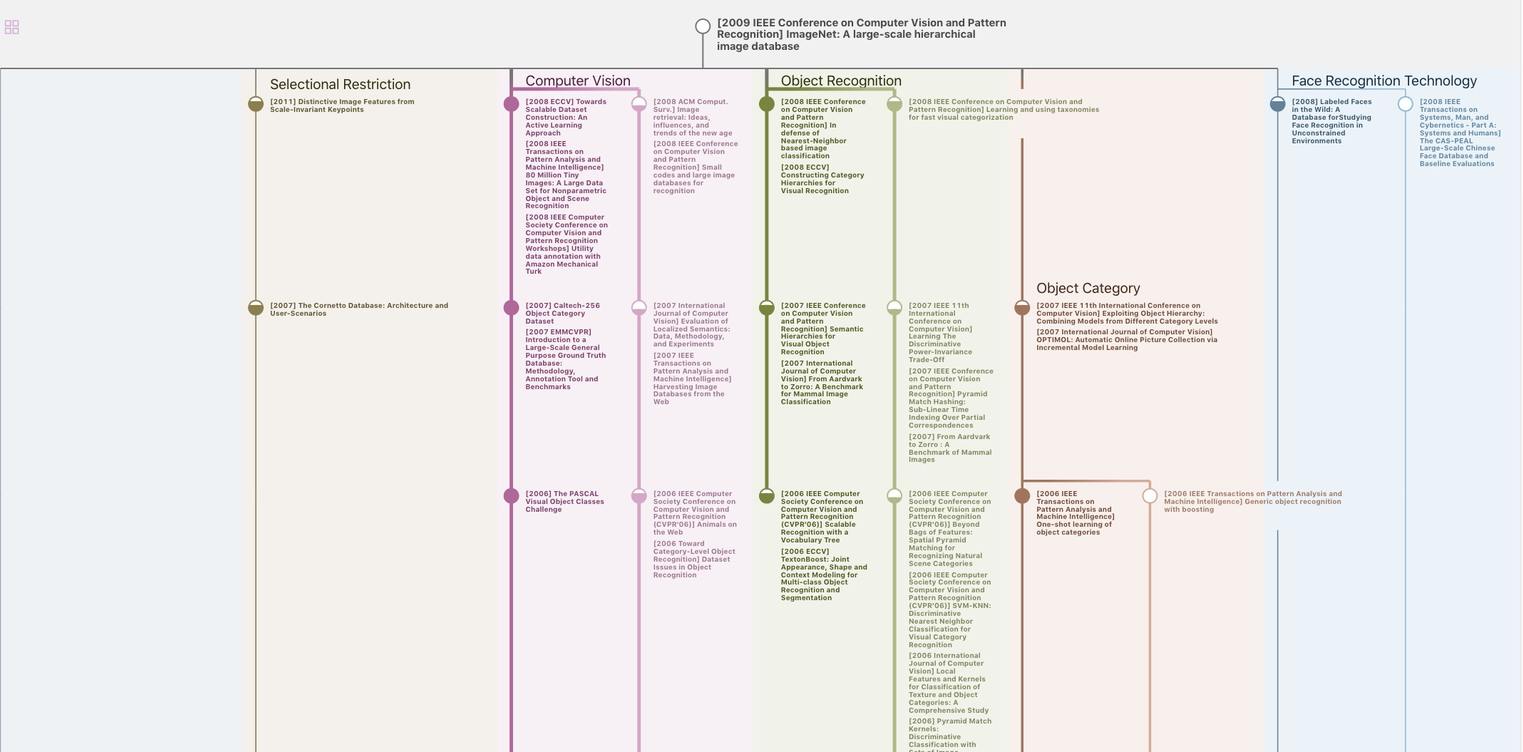
生成溯源树,研究论文发展脉络
Chat Paper
正在生成论文摘要