Deep Variational Autoencoder for Modeling Functional Brain Networks and ADHD Identification
2020 IEEE 17TH INTERNATIONAL SYMPOSIUM ON BIOMEDICAL IMAGING (ISBI 2020)(2020)
摘要
In the neuroimaging and brain mapping communities, researchers have proposed a variety of computational methods and tools to learn functional brain networks (FBNs). Recently, it has already been proven that deep learning can be applied on fMRI data with superb representation power over traditional machine learning methods. Limited by the high-dimension of fMRI volumes, deep learning suffers from the lack of data and overfitting. Generative models are known to have intrinsic ability of modeling small dataset and a Deep Variational Autoencoder (DVAE) is proposed in this work to tackle the challenge of insufficient data and incomplete supervision. The FBNs learned from fMRI were examined to be interpretable and meaningful and it was proven that DVAE has better performance on neuroimaging dataset over traditional models. With an evaluation on ADHD-200 dataset, DVAE performed excellent on classification accuracies on 4 sites.
更多查看译文
关键词
Resting-state fMRI, Variational Autoencoder, ADHD, Deep Learning, Generative Learning
AI 理解论文
溯源树
样例
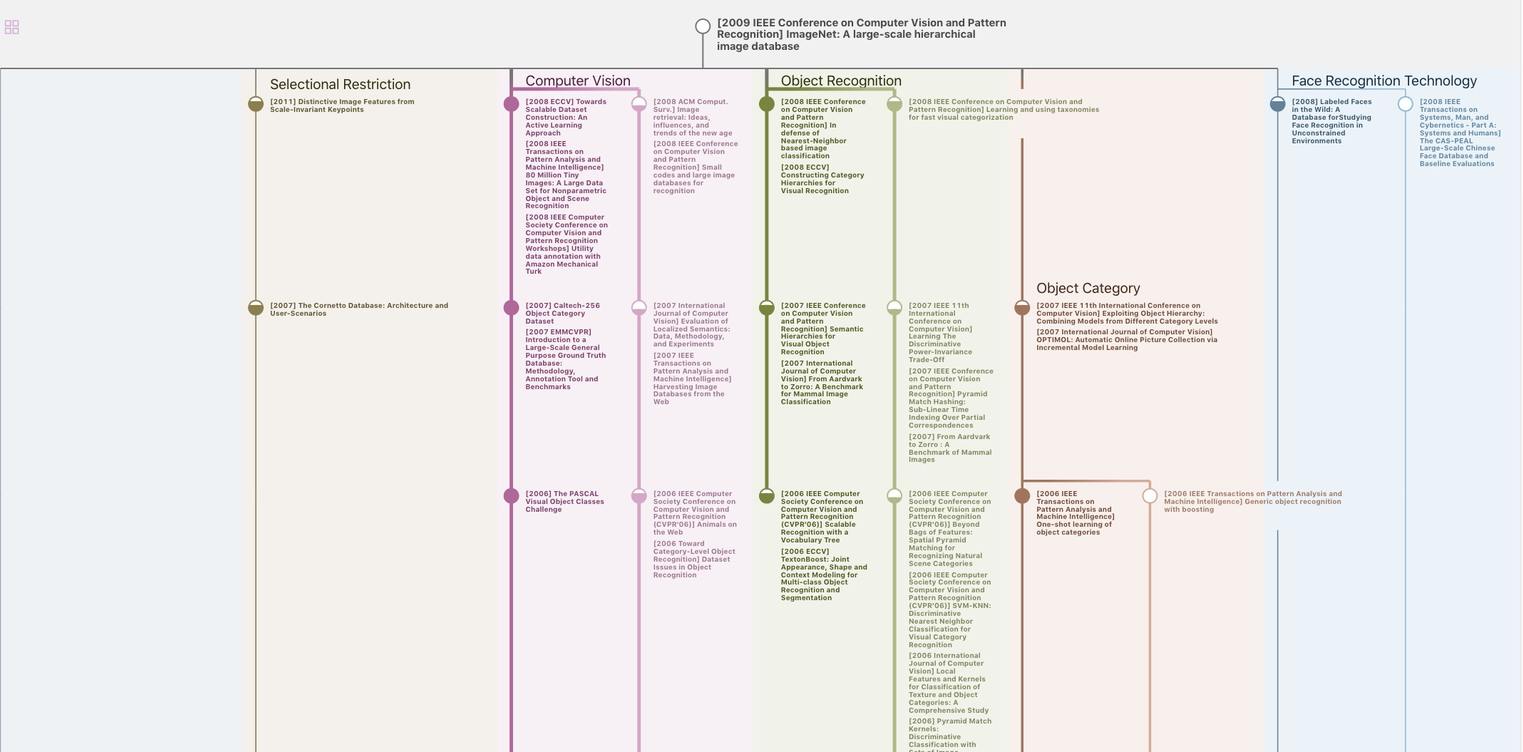
生成溯源树,研究论文发展脉络
Chat Paper
正在生成论文摘要