Semi-Supervised Cervical Dysplasia Classification With Learnable Graph Convolutional Network
2020 IEEE 17TH INTERNATIONAL SYMPOSIUM ON BIOMEDICAL IMAGING (ISBI 2020)(2020)
摘要
Cervical cancer is the second most prevalent cancer affecting women today. As the early detection of cervical carcinoma relies heavily upon screening and pre-clinical testing, digital cervicography has great potential as a primary or auxiliary screening tool, especially in low-resource regions due to its low cost and easy access. Although an automated cervical dysplasia detection system has been desirable, traditional fully-supervised training of such systems requires large amounts of annotated data which are often labor-intensive to collect. To alleviate the need for much manual annotation, we propose a novel graph convolutional network (GCN) based semi-supervised classification model that can be trained with fewer annotations. In existing GCNs, graphs are constructed with fixed features and can not be updated during the learning process. This limits their ability to exploit new features learned during graph convolution. In this paper, we propose a novel and more flexible GCN model with a feature encoder that adaptively updates the adjacency matrix during learning and demonstrate that this model design leads to improved performance. Our experimental results on a cervical dysplasia classification dataset show that the proposed framework outperforms previous methods under a semi-supervised setting, especially when the labeled samples are scarce.
更多查看译文
关键词
Semi-supervised learning, Graph convolutional network, Cervical cancer classification
AI 理解论文
溯源树
样例
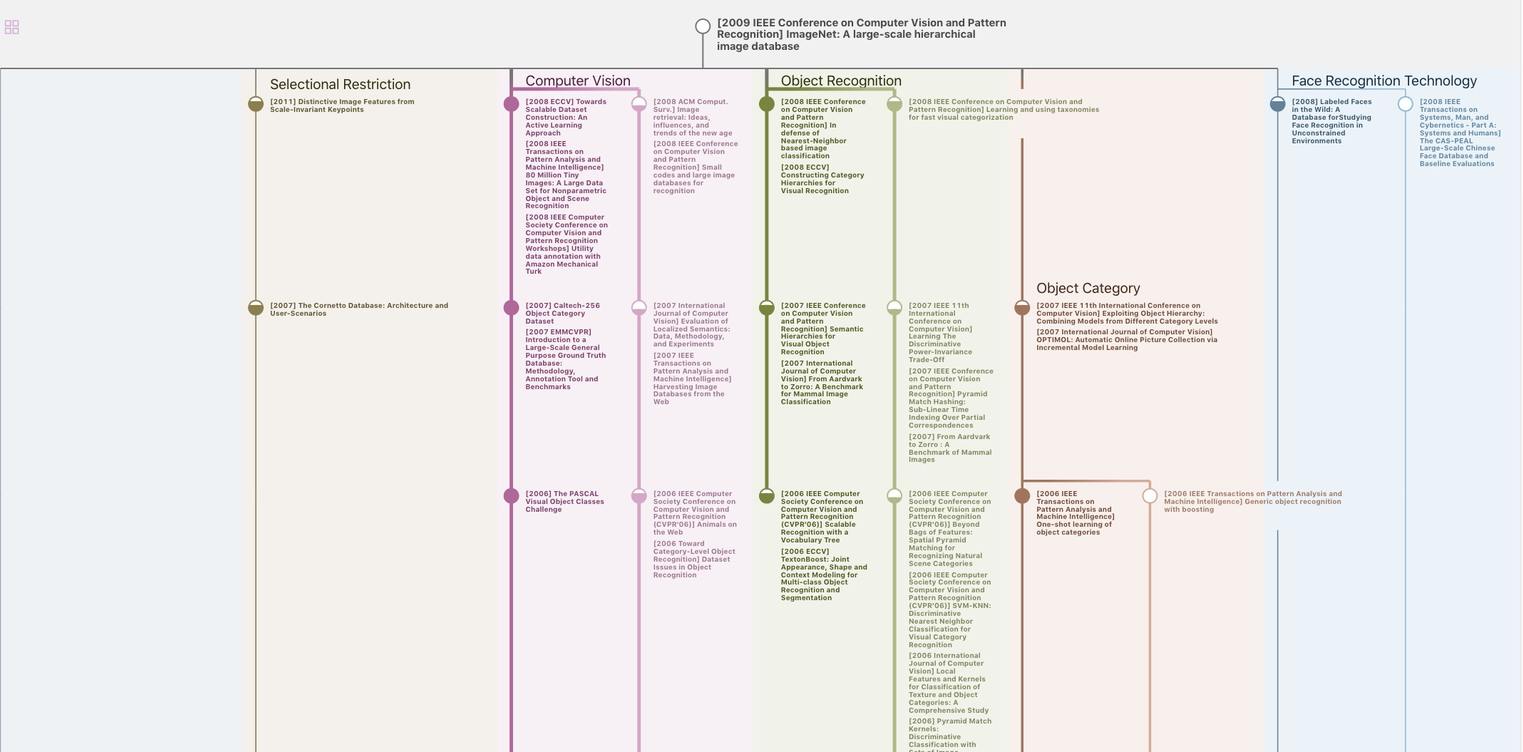
生成溯源树,研究论文发展脉络
Chat Paper
正在生成论文摘要