Region of Interest Identification for Cervical Cancer Images
2020 IEEE 17th International Symposium on Biomedical Imaging (ISBI)(2020)
摘要
Every two minutes one woman dies of cervical cancer globally, due to lack of sufficient screening. Given a whole slide image (WSI) obtained by scanning a microscope glass slide for a Liquid Based Cytology (LBC) based Pap test, our goal is to assist the pathologist to determine presence of precancerous or cancerous cervical anomalies. Inter-annotator variation, large image sizes, data imbalance, stain variations, and lack of good annotation tools make this problem challenging. Existing related work has focused on sub-problems like cell segmentation and cervical cell classification but does not provide a practically feasible holistic solution. We propose a practical system architecture which is based on displaying regions of interest on WSIs containing potential anomaly for review by pathologists to increase productivity. We build multiple deep learning classifiers as part of the proposed architecture. Our experiments with a dataset of ~19000 regions of interest provides an accuracy of ~89% for a balanced dataset both for binary as well as 6-class classification settings. Our deployed system provides a top-5 accuracy of ~94%.
更多查看译文
关键词
cancerous cervical anomalies,inter-annotator variation,image sizes,data imbalance,stain variations,good annotation tools,cell segmentation,cervical cell classification,practically feasible holistic solution,practical system architecture,potential anomaly,pathologist,multiple deep learning classifiers,interest identification,cervical cancer images,slide image,WSI,microscope glass slide,Pap test,precancerous anomalies
AI 理解论文
溯源树
样例
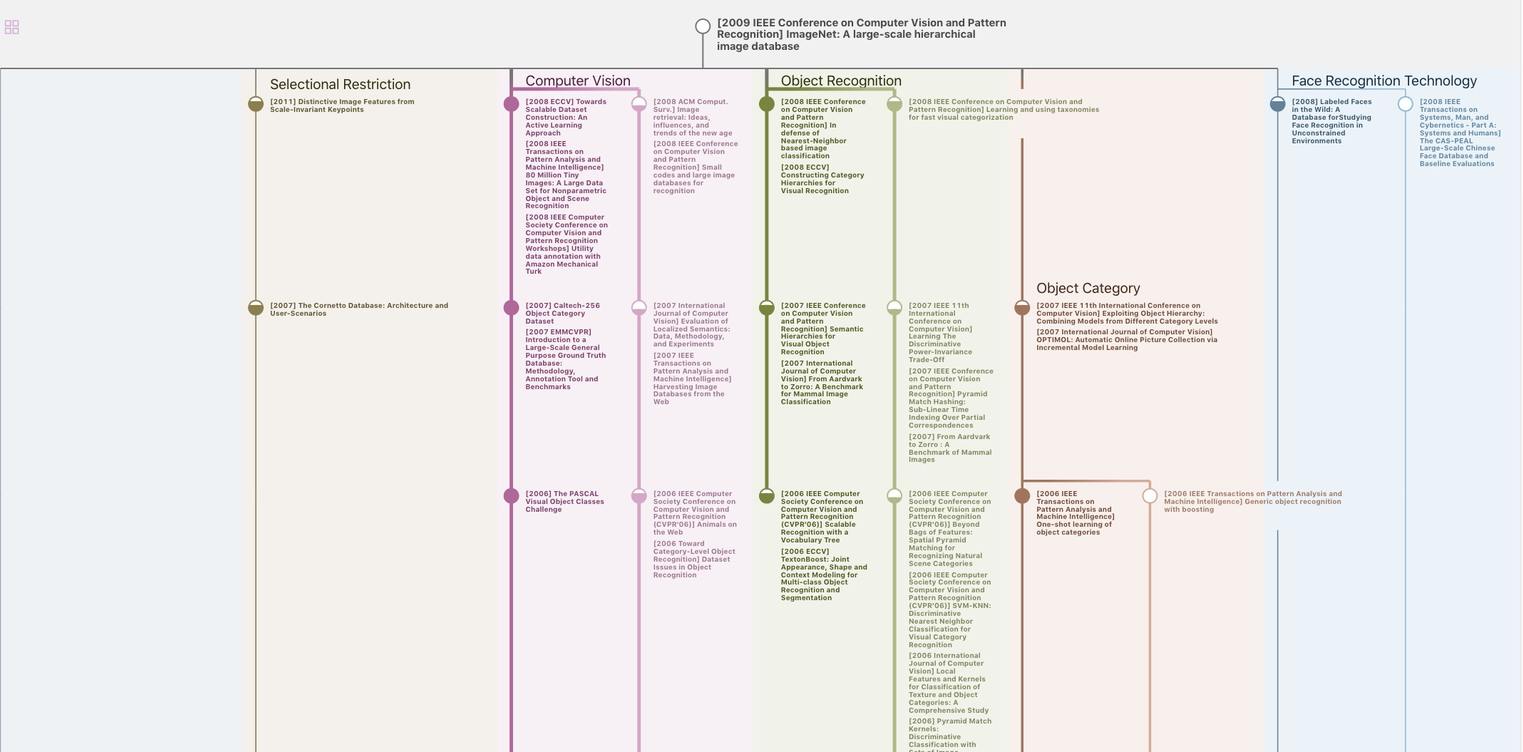
生成溯源树,研究论文发展脉络
Chat Paper
正在生成论文摘要