Airway Segmentation In Speech Mri Using The U-Net Architecture
2020 IEEE 17TH INTERNATIONAL SYMPOSIUM ON BIOMEDICAL IMAGING (ISBI 2020)(2020)
摘要
We develop a fully automated airway segmentation method to segment the vocal tract airway from surrounding soft tissue in speech MRI. We train a U-net architecture to learn the end to end mapping between a mid-sagittal image (at the input), and the manually segmented airway (at the output). We base our training on the open source University of Southern California's (USC) speech morphology MRI database consisting of speakers producing a variety of sustained vowel and consonant sounds. Once trained, our model performs fast airway segmentations on unseen images at the order of 210 ms/slice on a modern CPU with 12 cores. Using manual segmentation as a reference, we evaluate the performances of the proposed U-net airway segmentation, against existing seed-growing segmentation, and manual segmentation from a different user. We demonstrate improved DICE similarity with U-net compared to seed-growing, and minor differences in DICE similarity of U-net compared to manual segmentation from the second user.
更多查看译文
关键词
speech MRI,U-net architecture,fully automated airway segmentation method,vocal tract airway,end mapping,mid-sagittal image,manually segmented airway,open source University,Southern California's speech morphology MRI database,manual segmentation,U-net airway segmentation,existing seed-growing segmentation
AI 理解论文
溯源树
样例
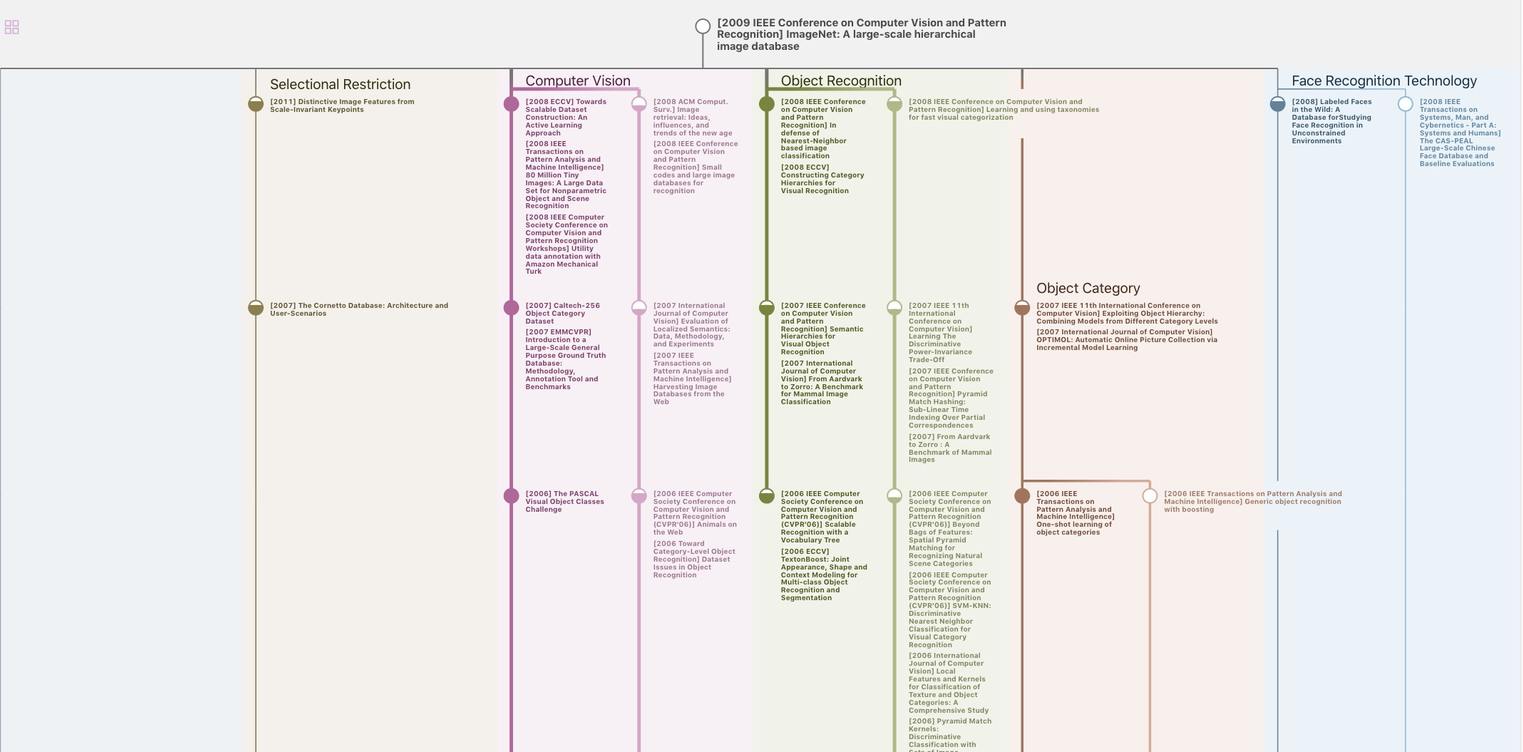
生成溯源树,研究论文发展脉络
Chat Paper
正在生成论文摘要