Neural Sparse Representation for Image Restoration
NIPS 2020(2020)
摘要
Inspired by the robustness and efficiency of sparse representation in sparse coding based image restoration models, we investigate the sparsity of neurons in deep networks. Our method structurally enforces sparsity constraints upon hidden neurons. The sparsity constraints are favorable for gradient-based learning algorithms and attachable to convolution layers in various networks. Sparsity in neurons enables computation saving by only operating on non-zero components without hurting accuracy. Meanwhile, our method can magnify representation dimensionality and model capacity with negligible additional computation cost. Experiments show that sparse representation is crucial in deep neural networks for multiple image restoration tasks, including image super-resolution, image denoising, and image compression artifacts removal. Code is available at https://github.com/ychfan/nsr
更多查看译文
关键词
neural sparse representation,image
AI 理解论文
溯源树
样例
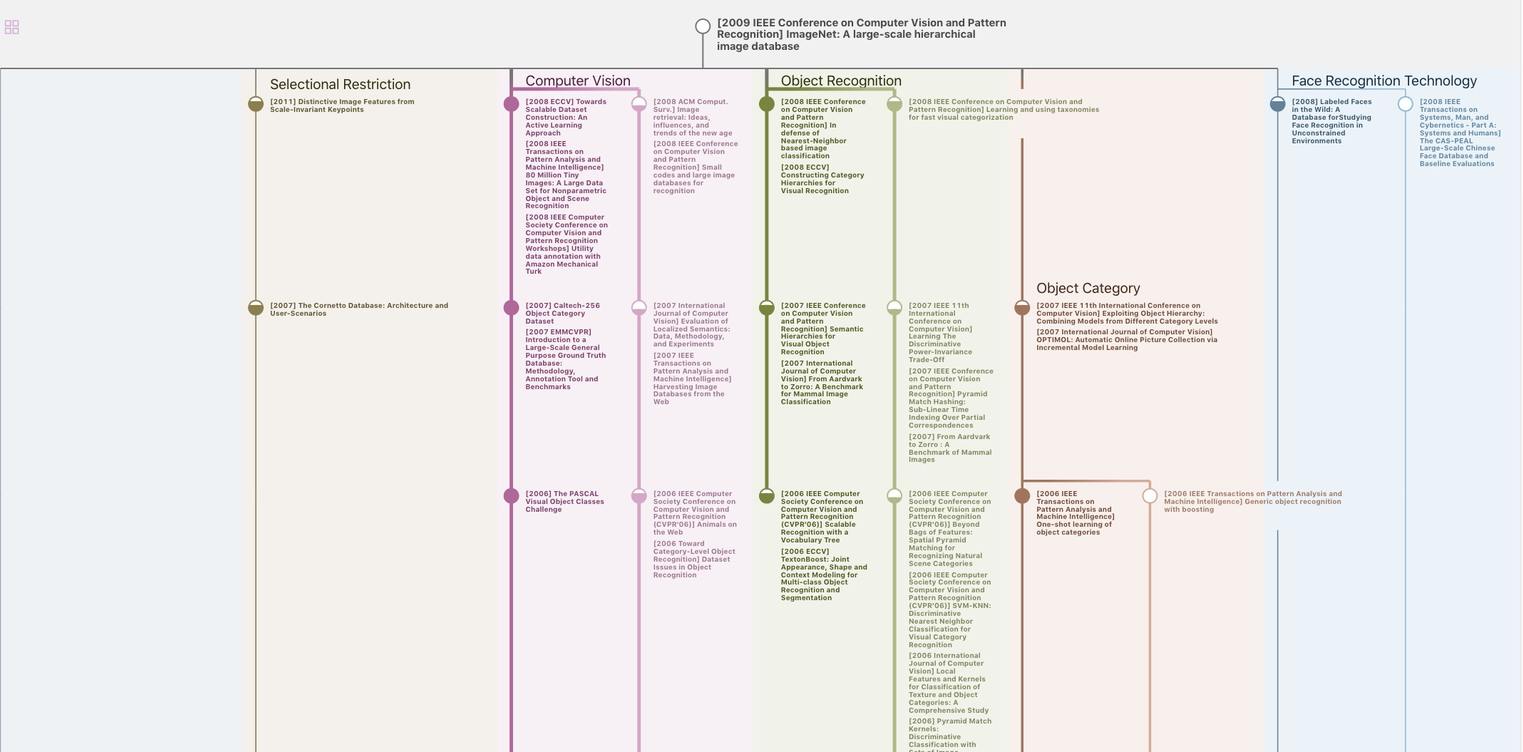
生成溯源树,研究论文发展脉络
Chat Paper
正在生成论文摘要