Ensemble Model with Batch Spectral Regularization and Data Blending for Cross-Domain Few-Shot Learning with Unlabeled Data
arxiv(2020)
摘要
Deep learning models are difficult to obtain good performance when data is scarce and there are domain gaps. Cross-domain few-shot learning (CD-FSL) is designed to improve this problem. We propose an Ensemble Model with Batch Spectral Regularization and Data Blending for the Track 2 of the CD-FSL challenge. We use different feature mapping matrices to obtain an Ensemble framework. In each branch, Batch Spectral Regularization is used to suppress the singular values of the feature matrix to improve the model's transferability. In the fine-tuning stage a data blending method is used to fuse the information of unlabeled data with the support set. The prediction result is refined with label propagation. We conduct experiments on the CD-FSL benchmark tasks and the results demonstrate the effectiveness of the proposed method.
更多查看译文
AI 理解论文
溯源树
样例
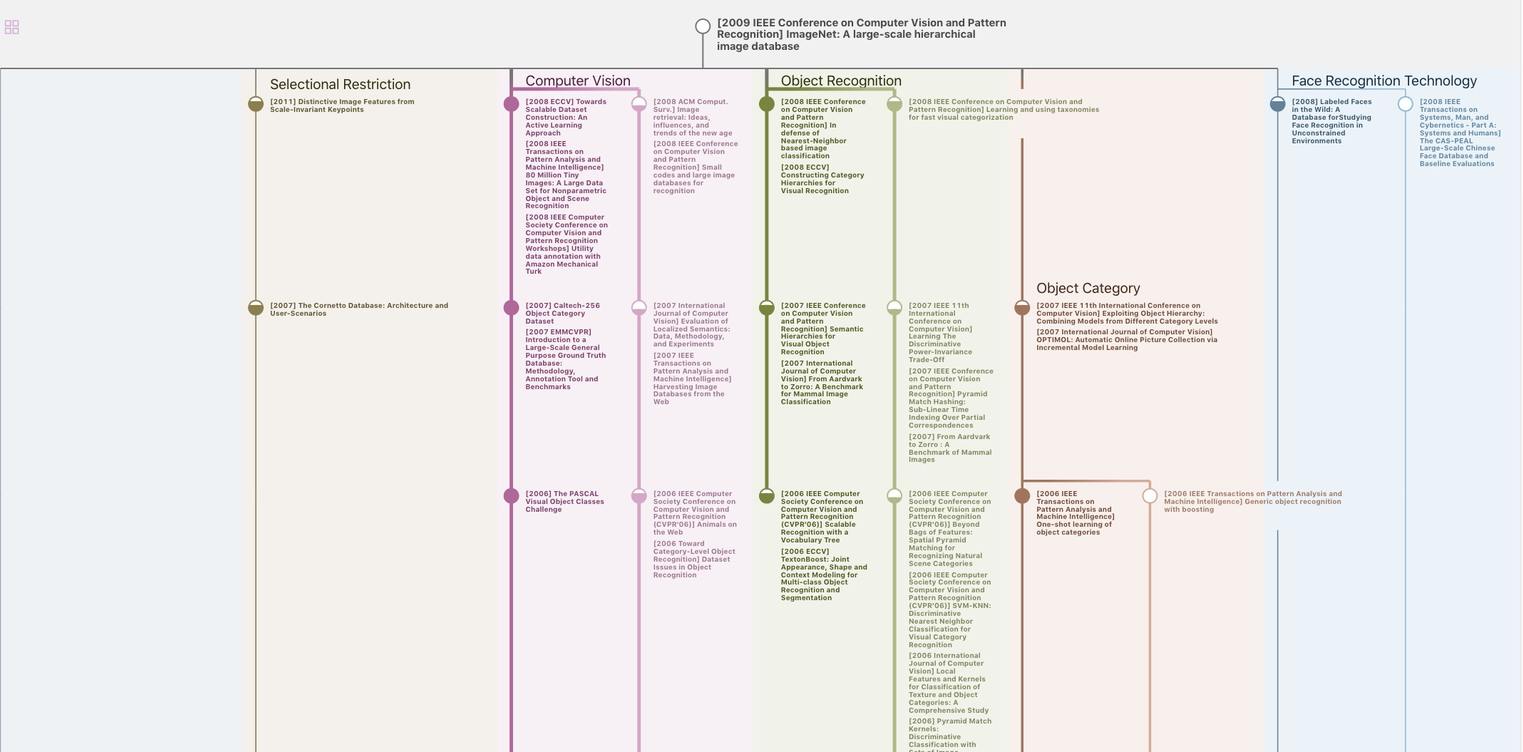
生成溯源树,研究论文发展脉络
Chat Paper
正在生成论文摘要