Counterfactual VQA: A Cause-Effect Look at Language Bias
2021 IEEE/CVF CONFERENCE ON COMPUTER VISION AND PATTERN RECOGNITION, CVPR 2021(2021)
摘要
VQA models may tend to rely on language bias as a shortcut and thus fail to sufficiently learn the multi-modal knowledge from both vision and language. Recent debiasing methods proposed to exclude the language prior during inference. However, they fail to disentangle the "good" language context and "bad" language bias from the whole. In this paper, we investigate how to mitigate language bias in VQA. Motivated by causal effects, we proposed a novel counterfactual inference framework, which enables us to capture the language bias as the direct causal effect of questions on answers and reduce the language bias by subtracting the direct language effect from the total causal effect. Experiments demonstrate that our proposed counterfactual inference framework 1) is general to various VQA backbones and fusion strategies, 2) achieves competitive performance on the language-bias sensitive VQA-CP dataset while performs robustly on the balanced VQA v2 dataset without any augmented data.
更多查看译文
关键词
language-bias sensitive VQA-CP dataset,language context,direct language effect,counterfactual VQA,cause-effect look,VQA backbones,balanced VQA v2 dataset,language bias,counterfactual inference framework,visual question answering
AI 理解论文
溯源树
样例
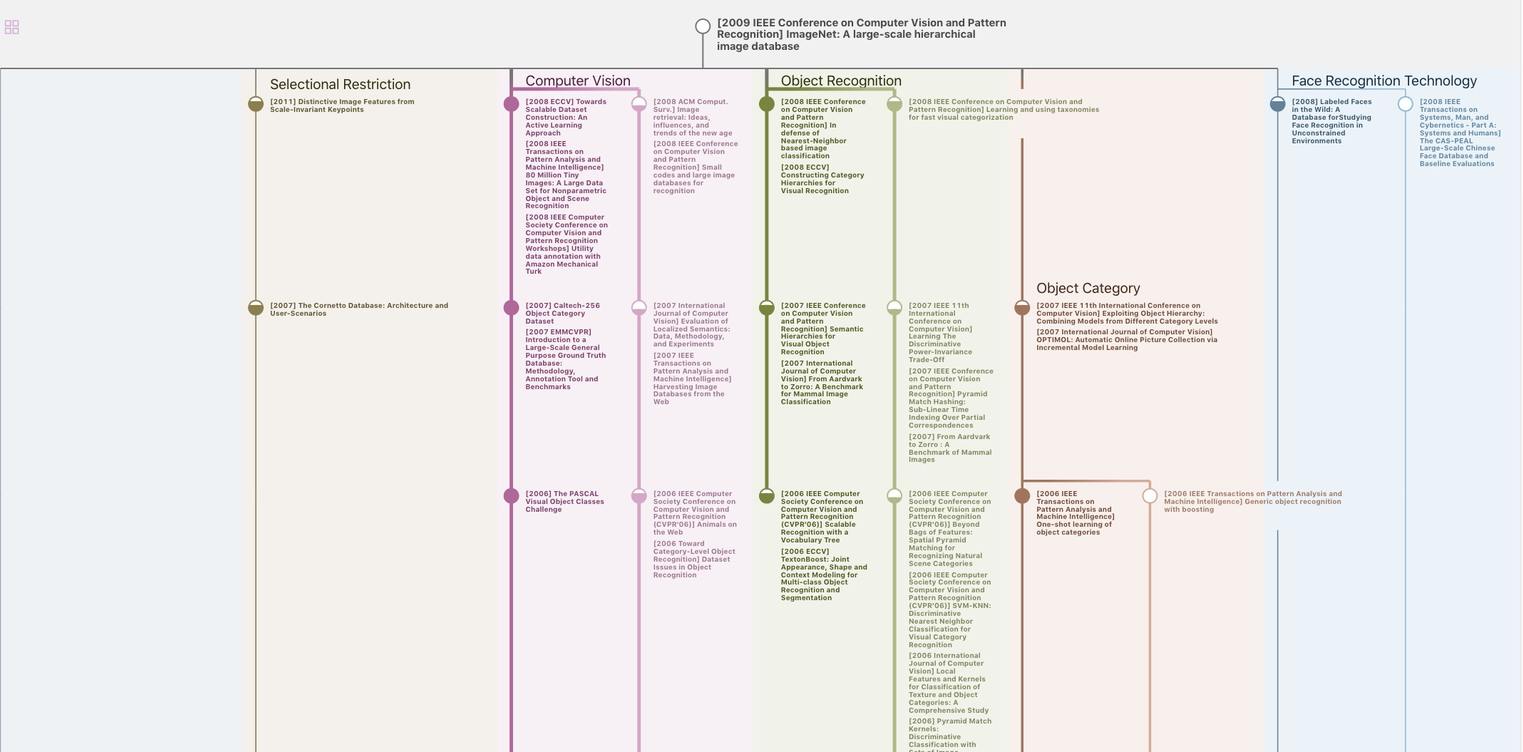
生成溯源树,研究论文发展脉络
Chat Paper
正在生成论文摘要