Generating Artificial Outliers in the Absence of Genuine Ones — A Survey
ACM Transactions on Knowledge Discovery from Data(2021)
摘要
AbstractBy definition, outliers are rarely observed in reality, making them difficult to detect or analyze. Artificial outliers approximate such genuine outliers and can, for instance, help with the detection of genuine outliers or with benchmarking outlier-detection algorithms. The literature features different approaches to generate artificial outliers. However, systematic comparison of these approaches remains absent. This surveys and compares these approaches. We start by clarifying the terminology in the field, which varies from publication to publication, and we propose a general problem formulation. Our description of the connection of generating outliers to other research fields like experimental design or generative models frames the field of artificial outliers. Along with offering a concise description, we group the approaches by their general concepts and how they make use of genuine instances. An extensive experimental study reveals the differences between the generation approaches when ultimately being used for outlier detection. This survey shows that the existing approaches already cover a wide range of concepts underlying the generation, but also that the field still has potential for further development. Our experimental study does confirm the expectation that the quality of the generation approaches varies widely, for example, in terms of the dataset they are used on. Ultimately, to guide the choice of the generation approach in a specific context, we propose an appropriate general-decision process. In summary, this survey comprises, describes, and connects all relevant work regarding the generation of artificial outliers and may serve as a basis to guide further research in the field.
更多查看译文
关键词
Artificial outlier, outlier detection, anomalies, artificial data
AI 理解论文
溯源树
样例
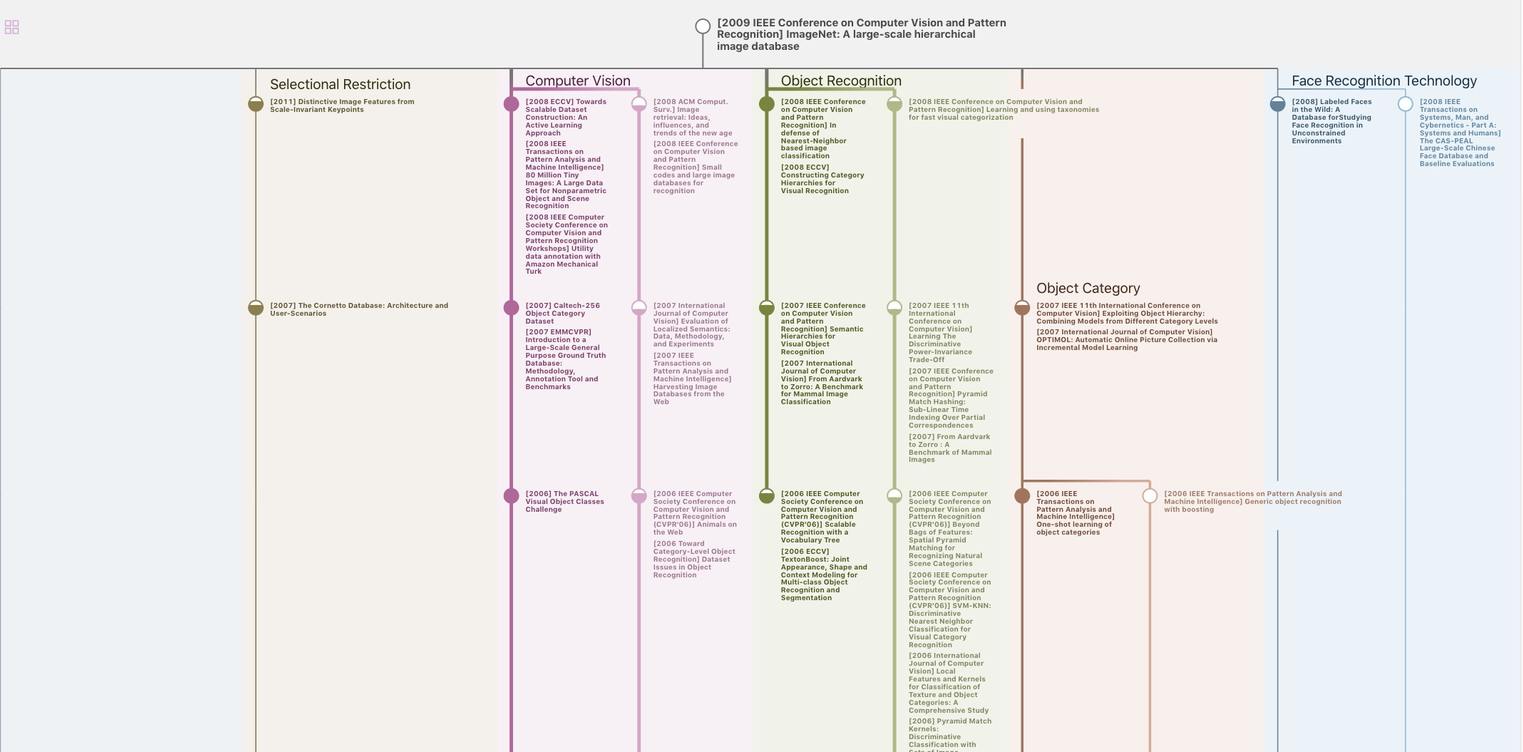
生成溯源树,研究论文发展脉络
Chat Paper
正在生成论文摘要