Per-Image Super-Resolution for Material BTFs
2020 IEEE International Conference on Computational Photography (ICCP)(2020)
摘要
Image-based appearance measurements are fundamentally limited in spatial resolution by the acquisition hardware. Due to the ever-increasing resolution of displaying hardware, high-resolution representations of digital material appearance are desireable for authentic renderings. In the present paper, we demonstrate that high-resolution bidirectional texture functions (BTFs) for materials can be obtained from low-resolution measurements using single-image convolutional neural network (CNN) architectures for image super-resolution. In particular, we show that this approach works for high-dynamic-range data and produces consistent BTFs, even though it operates on an image-by-image basis. Moreover, the CNN can be trained on down-sampled measured data, therefore no high-resolution ground-truth data, which would be difficult to obtain, is necessary. We train and test our method's performance on a large-scale BTF database and evaluate against the current state-of-the-art in BTF super-resolution, finding superior performance.
更多查看译文
关键词
Super-Resolution,Appearance Capture,Deep Learning
AI 理解论文
溯源树
样例
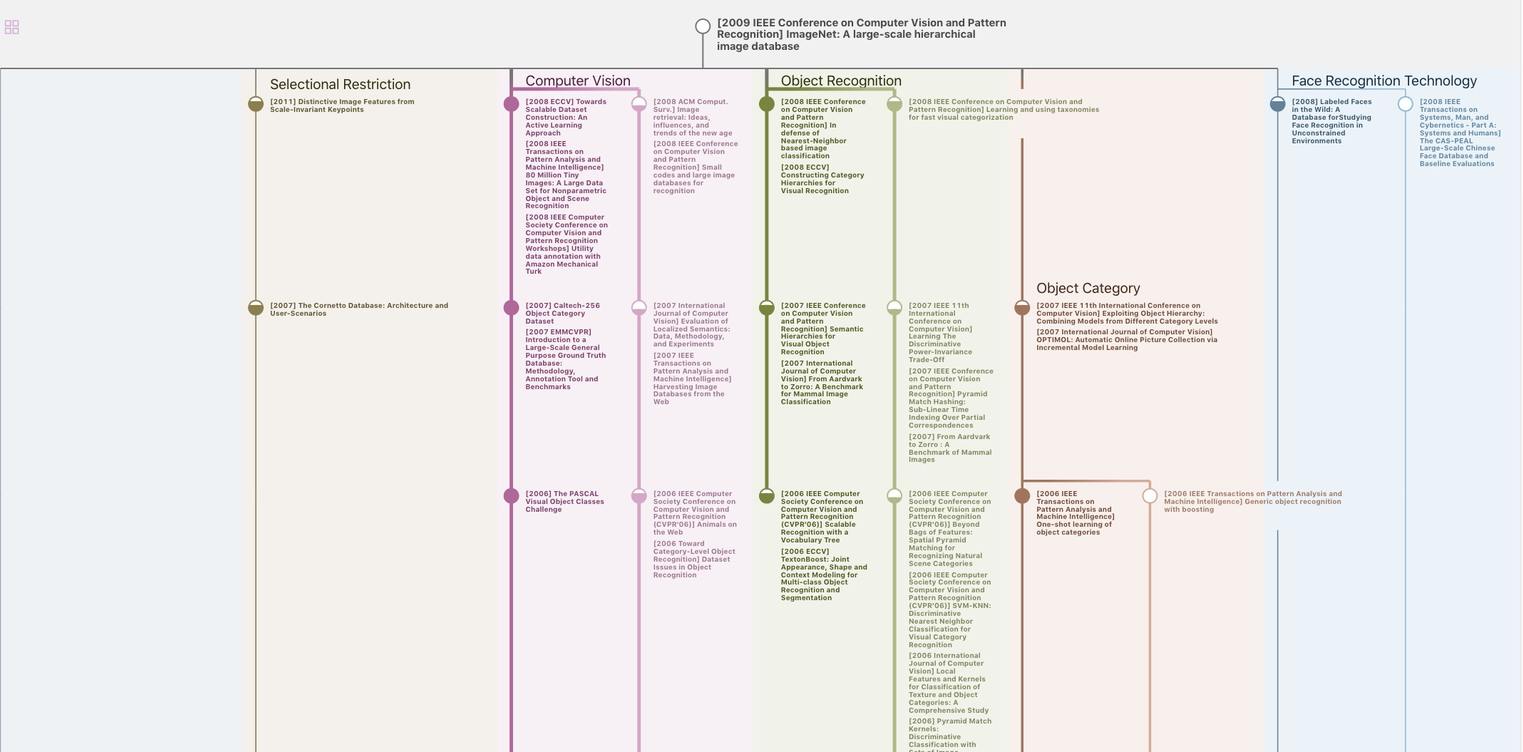
生成溯源树,研究论文发展脉络
Chat Paper
正在生成论文摘要