Dynamic maintenance of rough approximations in multi-source hybrid information systems.
Information Sciences(2020)
摘要
In many real-life applications, the data collected from different information sources are always located in diverse sites and characterized by different types of attributes. In this paper, we call this type of data as multi-source hybrid data. Existed rough set models only work well with single source data or multi-source data with one type of attributes, which are not suitable for processing multi-source hybrid data. Furthermore, in dynamic data environment, multi-source hybrid data are typically evolving over time, including the addition of objects and attributes and the revision of missing values in each information source. It will result in the update of information granulation and rough approximation structures. To address these issues, we present an extended rough set model, named as multi-source composite rough sets (MCRS), by integrating different types of attributes and fusing multiple composite relations derived from different information sources. Two novel matrix operators are proposed to construct the matrix-based representation of rough approximations. Then, under the variations of objectives, attributes and attribute values in the multi-source hybrid information system, we present incremental mechanisms based on matrices for the maintenance of approximations by utilizing the previously accumulated matrices information and transmitting location information of part elements in each composite relation matrix. In addition, we develop the incremental algorithms for updating composite rough approximations. A series of comparative experiments show that the proposed algorithm outperforms the static algorithm and other extended incremental algorithms in terms of the efficiency of computation and transmission.
更多查看译文
关键词
Multi-source hybrid data,Incremental learning,Rough sets,Matrix operators
AI 理解论文
溯源树
样例
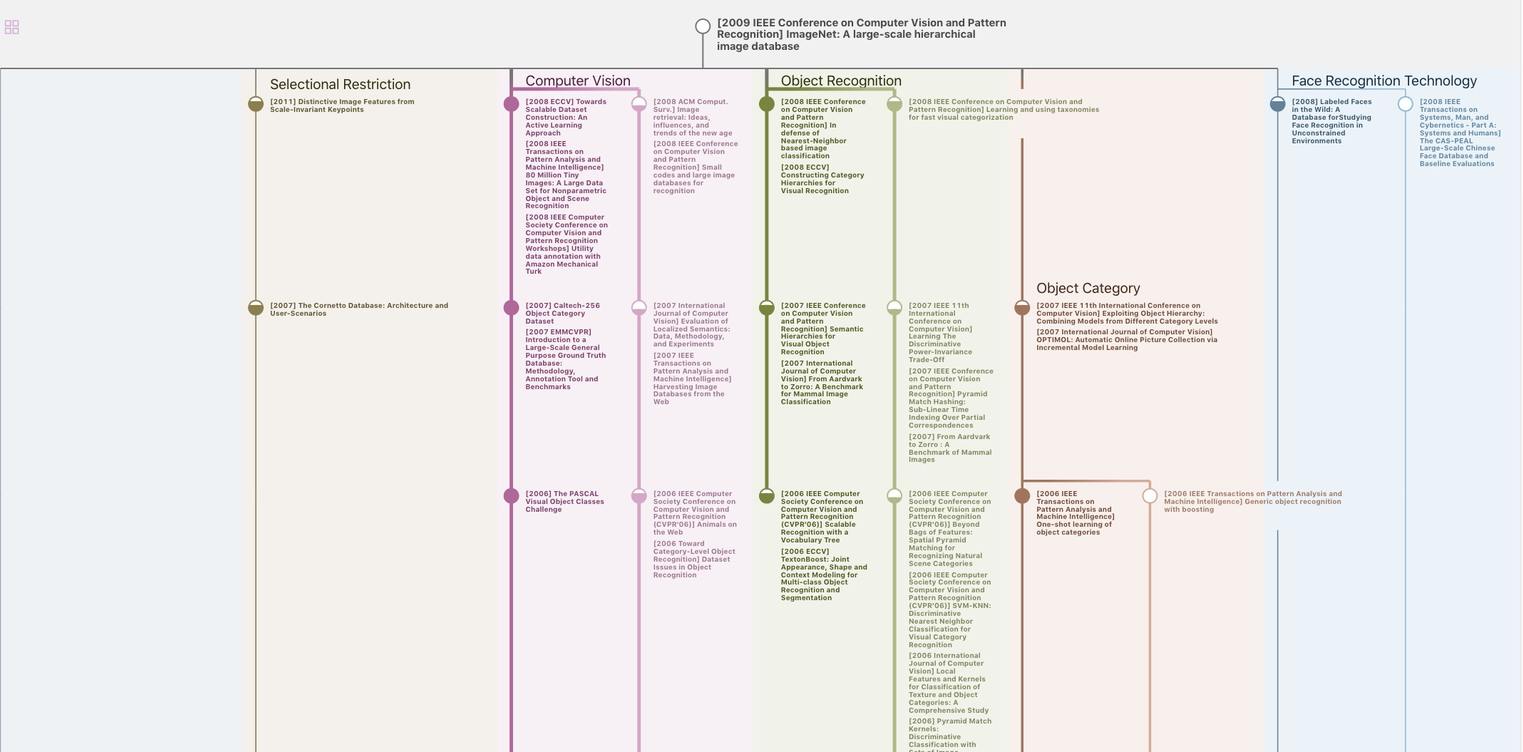
生成溯源树,研究论文发展脉络
Chat Paper
正在生成论文摘要