Improved Correlated Sampling for Join Size Estimation
2020 IEEE 36th International Conference on Data Engineering (ICDE)(2020)
摘要
Recent research on sampling-based join size estimation has focused on a promising new technique known as correlated sampling. While several variants of this technique have been proposed, there is a lack of a systematic study of this family of techniques. In this paper, we first introduce a framework to characterize its design space in terms of five parameters. Based on this framework, we propose a new correlated sampling based technique to address the limitations of existing techniques. Our new technique is based on using a discrete learning method for estimating the join size from samples. We experimentally compare the performance of multiple variants of our new technique and identify a hybrid variant that provides the best estimation quality. This hybrid variant not only outperforms the state-of-the-art correlated sampling technique, but it is also more robust to small samples and skewed data.
更多查看译文
关键词
query processing,database systems,sampling methods
AI 理解论文
溯源树
样例
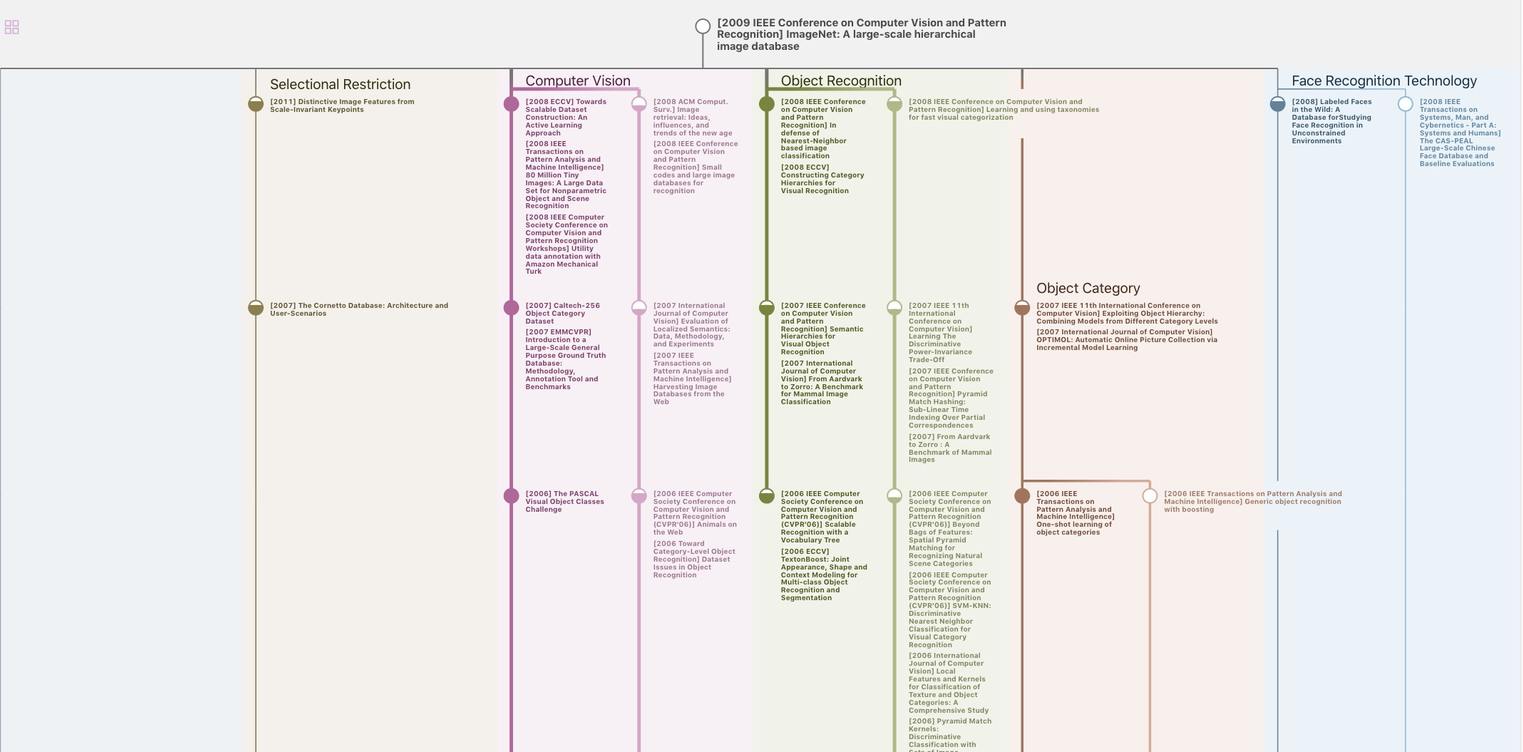
生成溯源树,研究论文发展脉络
Chat Paper
正在生成论文摘要