Online Anomalous Trajectory Detection with Deep Generative Sequence Modeling
2020 IEEE 36th International Conference on Data Engineering (ICDE)(2020)
摘要
Detecting anomalous trajectory has become an important and fundamental concern in many real-world applications. However, most of the existing studies 1) cannot handle the complexity and variety of trajectory data and 2) do not support efficient anomaly detection in an online manner. To this end, we propose a novel model, namely Gaussian Mixture Variational Sequence AutoEncoder (GM-VSAE), to tackle these challenges. Our GM-VSAE model is able to (1) capture complex sequential information enclosed in trajectories, (2) discover different types of normal routes from trajectories and represent them in a continuous latent space, and (3) support efficient online detection via trajectory generation. Our experiments on two real-world datasets demonstrate that GM-VSAE is more effective than the state-of-the-art baselines and is efficient for online anomalous trajectory detection.
更多查看译文
关键词
online anomalous trajectory detection,deep generative Sequence modeling,trajectory data,efficient anomaly detection,Gaussian Mixture Variational Sequence AutoEncoder,GM-VSAE model,trajectory generation,real-world datasets
AI 理解论文
溯源树
样例
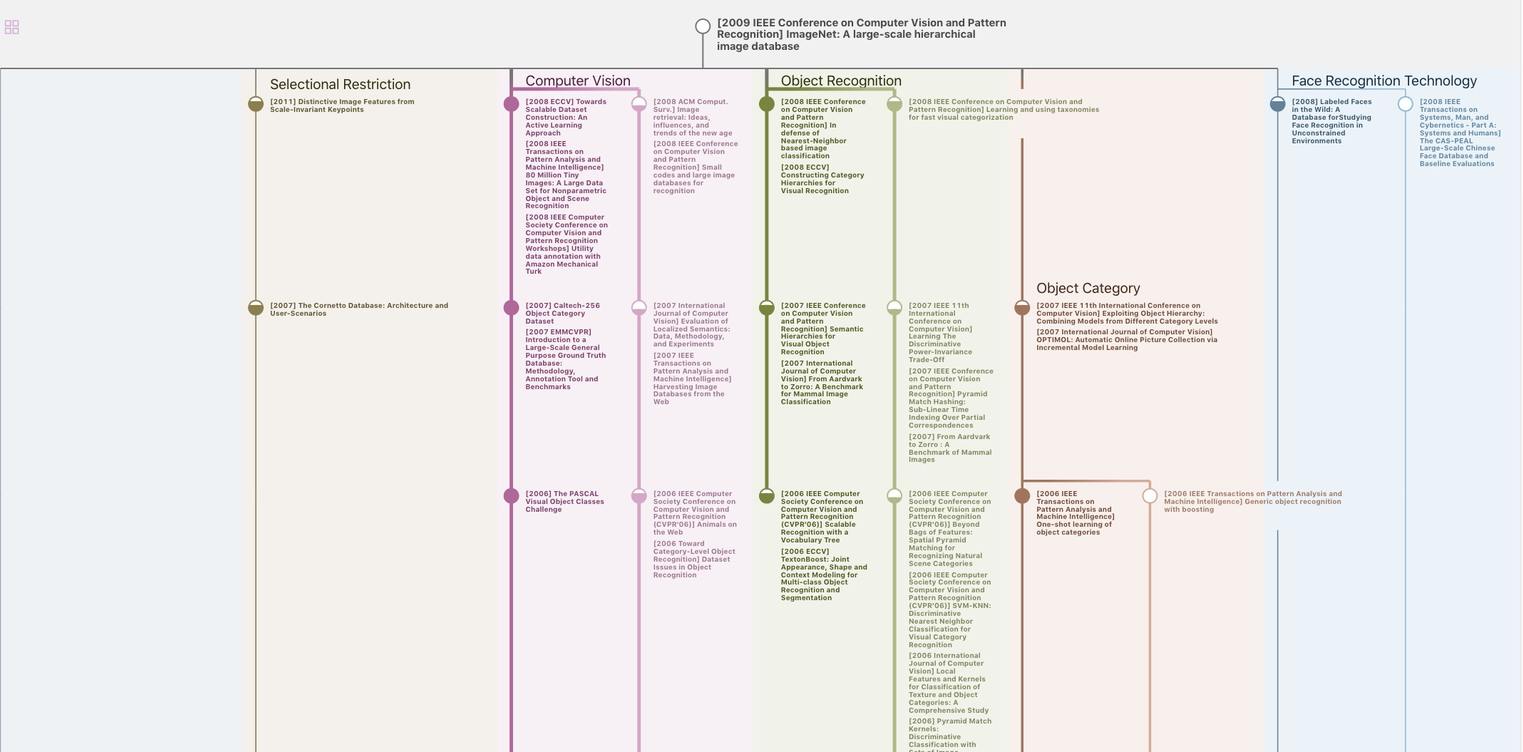
生成溯源树,研究论文发展脉络
Chat Paper
正在生成论文摘要