Improved predictability of peak periphyton in rivers using site-specific accrual periods and long-term water quality datasets.
Science of The Total Environment(2020)
摘要
Prevention of excessive periphyton standing crop (quantified as chlorophyll a) is among primary objectives for river management. Defensible instream nutrient criteria to achieve periphyton chlorophyll a targets at the site scale require robust predictive models. Such models have proved elusive because peak chlorophyll a depends on multiple factors in addition to nutrients. A key predictor may be accrual period, which depends on river flow variability and the flow magnitudes (effective flows, EF) at which periphyton biomass removal is initiated. In this study we used a seven-year dataset from 44 gravel-bed river sites in the Manawatū–Whanganui region, New Zealand, to explore the relative importance of accrual period, nutrients, and other variables in explaining peak chlorophyll a, using a regression approach. We also assessed the effect of combining data from multiple years. Previous empirical studies have used a universal flow metric (3 × median flow) to define accrual period (Da3). We calculated site-specific EF, which varied from 2 × to 15 × median flow. Accrual period based on EF (DaEF) outperformed Da3 in models. However, in the study region, more variance in chlorophyll a was explained by conductivity (EC) and dissolved inorganic nitrogen (DIN) than by DaEF. The best models derived from multi-year datasets included EC, DIN and DaEF as predictors and accounted for up to 82% of the variance in peak chlorophyll a. Models from annual data were weaker and more variable in strength and predictors. The models indicated that EC and DaEF should be considered when setting DIN criteria for periphyton outcomes in the study region. The principles we used in developing the models may have broad relevance to the management of periphyton in other regions.
更多查看译文
关键词
Chlorophyll a,Nutrient criteria,Conductivity,Accrual period,Empirical relationships
AI 理解论文
溯源树
样例
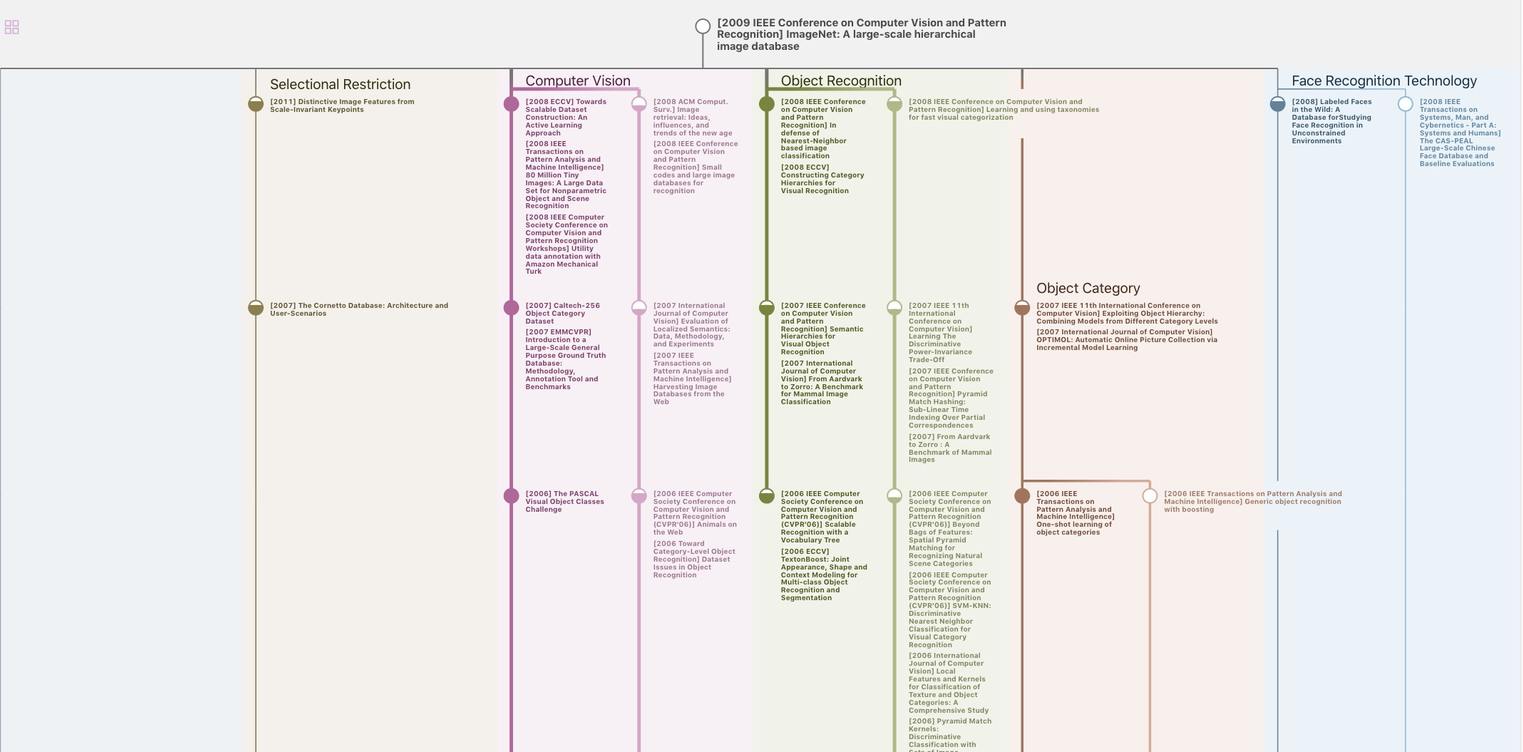
生成溯源树,研究论文发展脉络
Chat Paper
正在生成论文摘要