Low-dimensional Shape Analysis In the Space of Diffeomorphisms
Riemannian Geometric Statistics in Medical Image Analysis(2019)
摘要
Statistical shape analysis via diffeomorphic image registration is challenging due to the high-dimensional and nonlinear nature of the space of diffeomorphisms. In this chapter, we first introduce a low-dimensional representation of initial velocity fields (i.e., the tangent space of diffeomorphisms) in a bandlimited frequency domain, which leads to an efficient statistical analysis of anatomical shape changes characterized by diffeomorphic transformations. We then present a latent variable model for probabilistic principal geodesic analysis (PPGA) that quantifies shape variability using this new representation. We run our model on 3D brain MRI scans and demonstrate its efficiency in capturing the data variability across large populations. Experimental results show that our model estimates a more compact representation of group variation at a substantially lower computational cost than state-of-the-art methods, such as …
更多查看译文
关键词
shape,low-dimensional
AI 理解论文
溯源树
样例
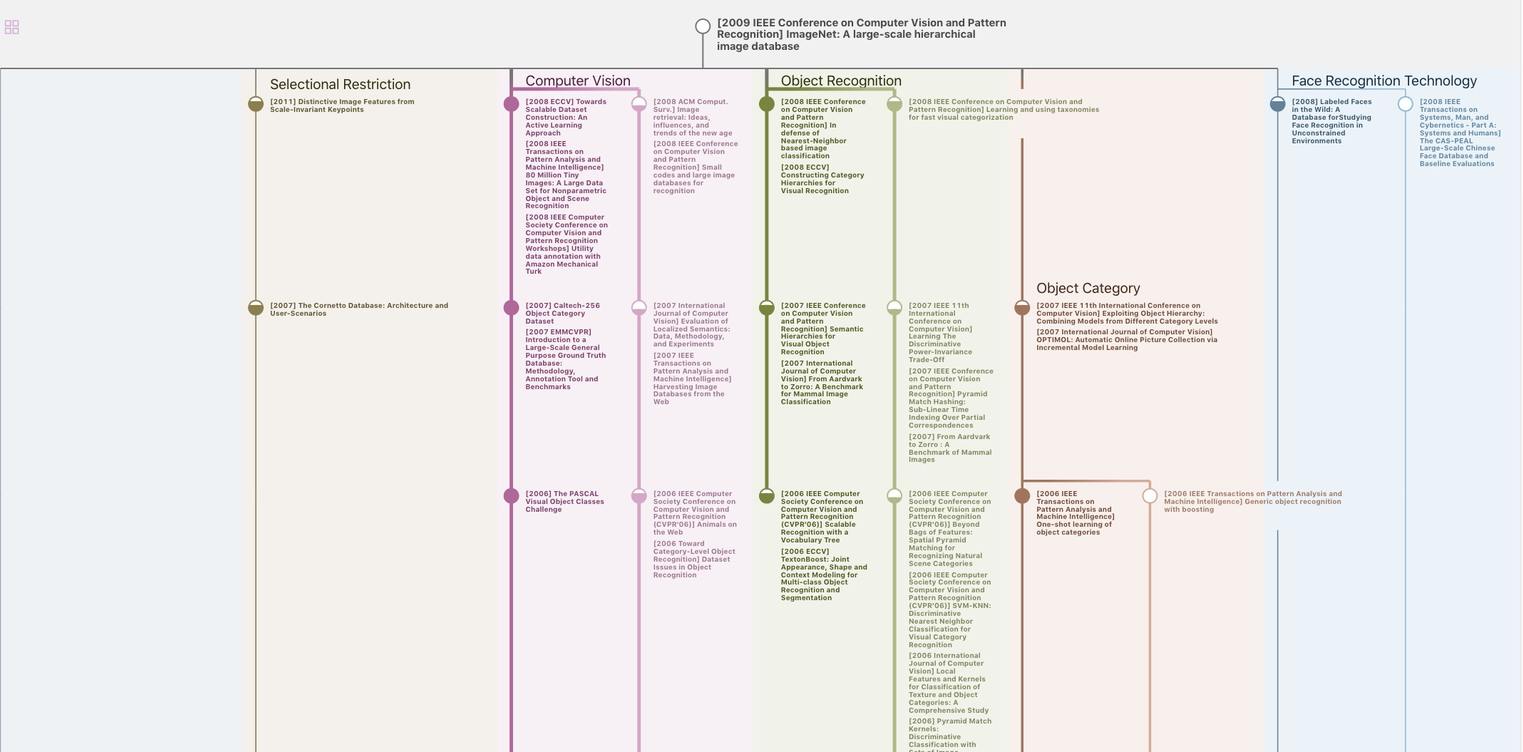
生成溯源树,研究论文发展脉络
Chat Paper
正在生成论文摘要