Tensor Representations for Object Classification and Detection
user-5ebe28d54c775eda72abcdf7(2013)
摘要
A novel method is proposed for robust detection and categorization of people from a wide-area distance by appearance and action. We adopt the tensor representation that is able to describe the interactions of multiple factors inherent to image formation and separately to encode the higher order statistics of each of these factors. Drawing inspiration by some successful works using covariance tensors [27, 28, 25], we propose two new kind of tensors that we have called EMI (Entropy and Mutual Information) tensor and SST (Self Similarity Tensor) that outperform the covariance representation on different classification and detection tasks. Then we present a complete framework for pedestrian detection based on the SST combined with Hausdorff distance that is able to manage object’s description of variable lengths. We combine different object models with tensor representation. For all those the basic ingredient is the patch which replaces the concept of part because, in a video surveillance context, it guarantees better performances in terms of robustness. Finally we test the proposed tensor representations and the classification and detection approaches on different object classification (LabelMe [24] and Pascal VOC 2009 [12]), object recognition (Cifar 10 and Cifar 100 [19]), and pedestrian detection (DaimlerChrysler [23] and Caltech Pedestrian [9]) datasets.
更多查看译文
AI 理解论文
溯源树
样例
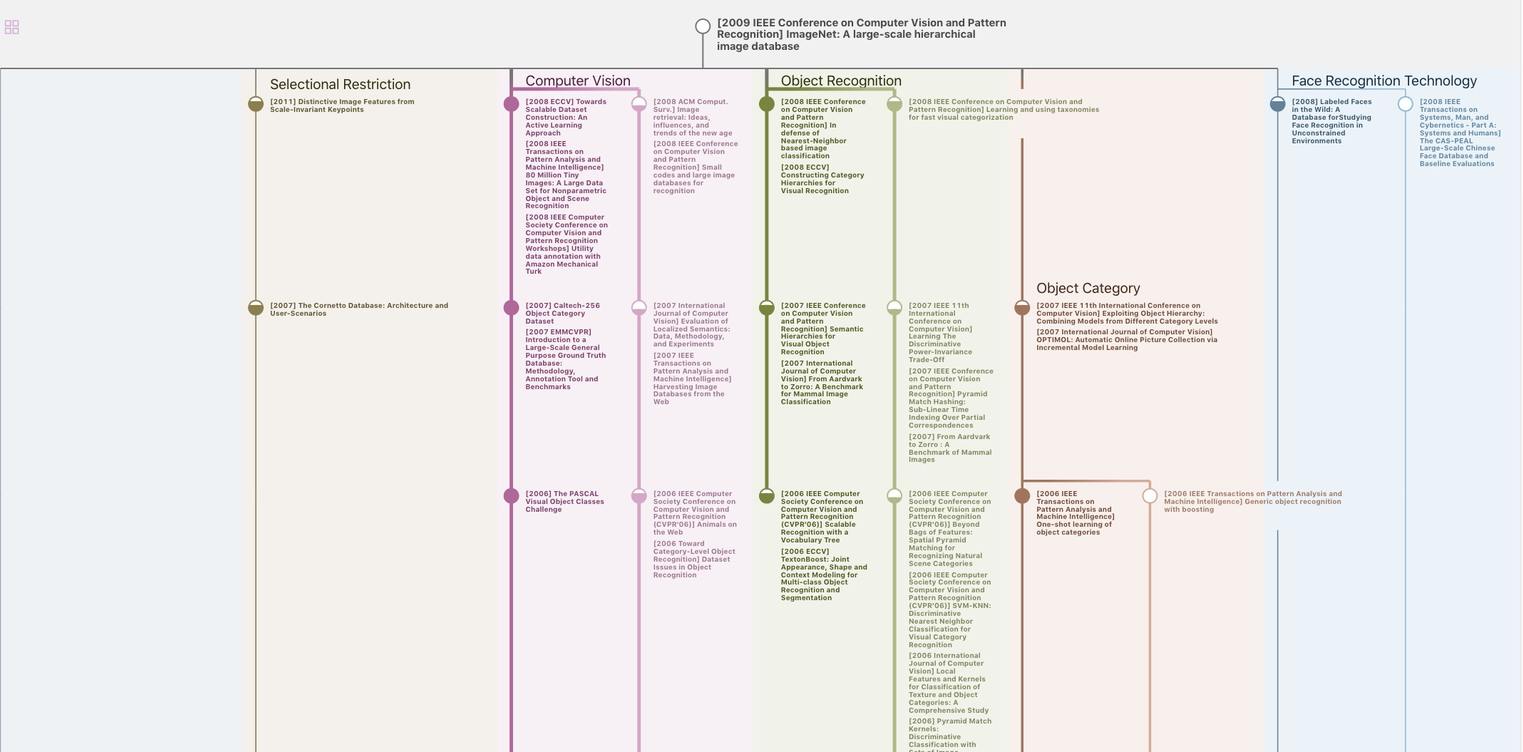
生成溯源树,研究论文发展脉络
Chat Paper
正在生成论文摘要