Decomposition In Decision And Objective Space For Multi-Modal Multi-Objective Optimization
SWARM AND EVOLUTIONARY COMPUTATION(2021)
摘要
Multi-modal multi-objective optimization problems (MMMOPs) have multiple subsets within the Pareto-optimal Set, each independently mapping to the same Pareto-Front. Prevalent multi-objective evolutionary algorithms are not purely designed to search for multiple solution subsets, whereas, algorithms designed for MMMOPs demon-strate degraded performance in the objective space. This motivates the design of better algorithms for addressing MMMOPs. The present work identifies the crowding illusion problem originating from using crowding distance globally over the entire decision space. Subsequently, an evolutionary framework, called graph Laplacian based Optimization using Reference vector assisted Decomposition (LORD), is proposed, which uses decomposition in both objective and decision space for dealing with MMMOPs. Its filtering step is further extended to present LORD-II algorithm, which demonstrates its dynamics on multi-modal many-objective problems. The efficacies of the frameworks are established by comparing their performance on test instances from the CEC 2019 multi-modal multi-objective test suite and polygon problems with the state-of-the-art algorithms for MMMOPs and other multi-and many-objective evolutionary algorithms. The manuscript is concluded by mentioning the limitations of the proposed frameworks and future directions to design still better algorithms for MMMOPs. The source code is available at https://worksupplements.droppages.com/lord .
更多查看译文
关键词
Multi-modal multi-objective Optimization, Non-dominated sorting, Crowding distance, Reference vector based decomposition, Graph Laplacian
AI 理解论文
溯源树
样例
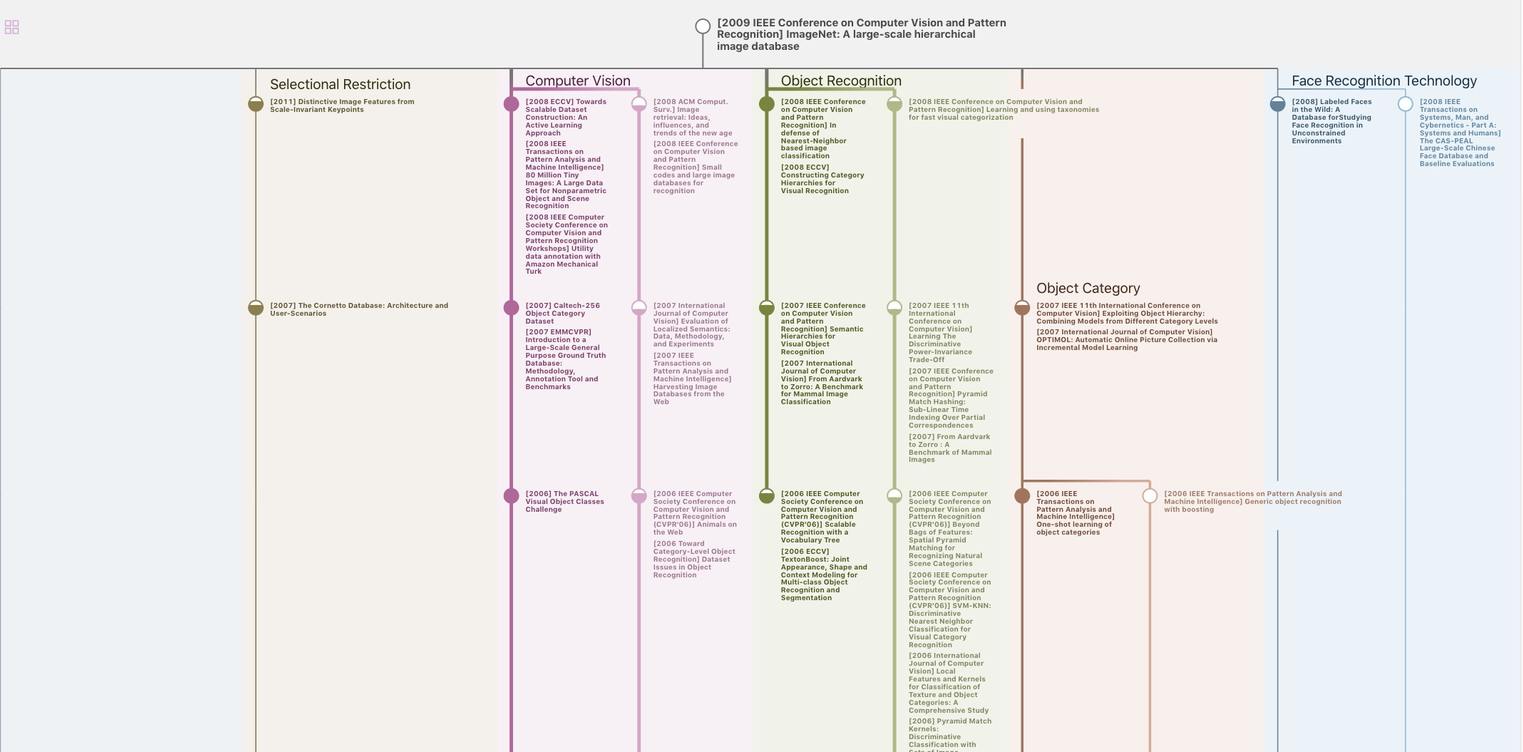
生成溯源树,研究论文发展脉络
Chat Paper
正在生成论文摘要