Interpolation-based Semi-supervised Learning for Object Detection
2021 IEEE/CVF CONFERENCE ON COMPUTER VISION AND PATTERN RECOGNITION, CVPR 2021(2021)
摘要
Despite the data labeling cost for the object detection tasks being substantially more than that of the classification tasks, semi-supervised learning methods for object detection have not been studied much. In this paper, we propose an Interpolation-based Semi-supervised learning method for object Detection (ISD), which considers and solves the problems caused by applying conventional Interpolation Regularization (IR) directly to object detection. We divide the output of the model into two types according to the objectness scores of both original patches that are mixed in IR. Then, we apply a separate loss suitable for each type in an unsupervised manner. The proposed losses dramatically improve the performance of semi-supervised learning as well as supervised learning. In the supervised learning setting, our method improves the baseline methods by a significant margin. In the semi-supervised learning setting, our algorithm improves the performance on a benchmark dataset (PASCAL VOC and MSCOCO) in a benchmark architecture (SSD).
更多查看译文
关键词
semisupervised learning setting,object detection tasks,semisupervised learning methods,Interpolation-based Semisupervised learning method,conventional Interpolation Regularization,objectness scores,supervised learning setting
AI 理解论文
溯源树
样例
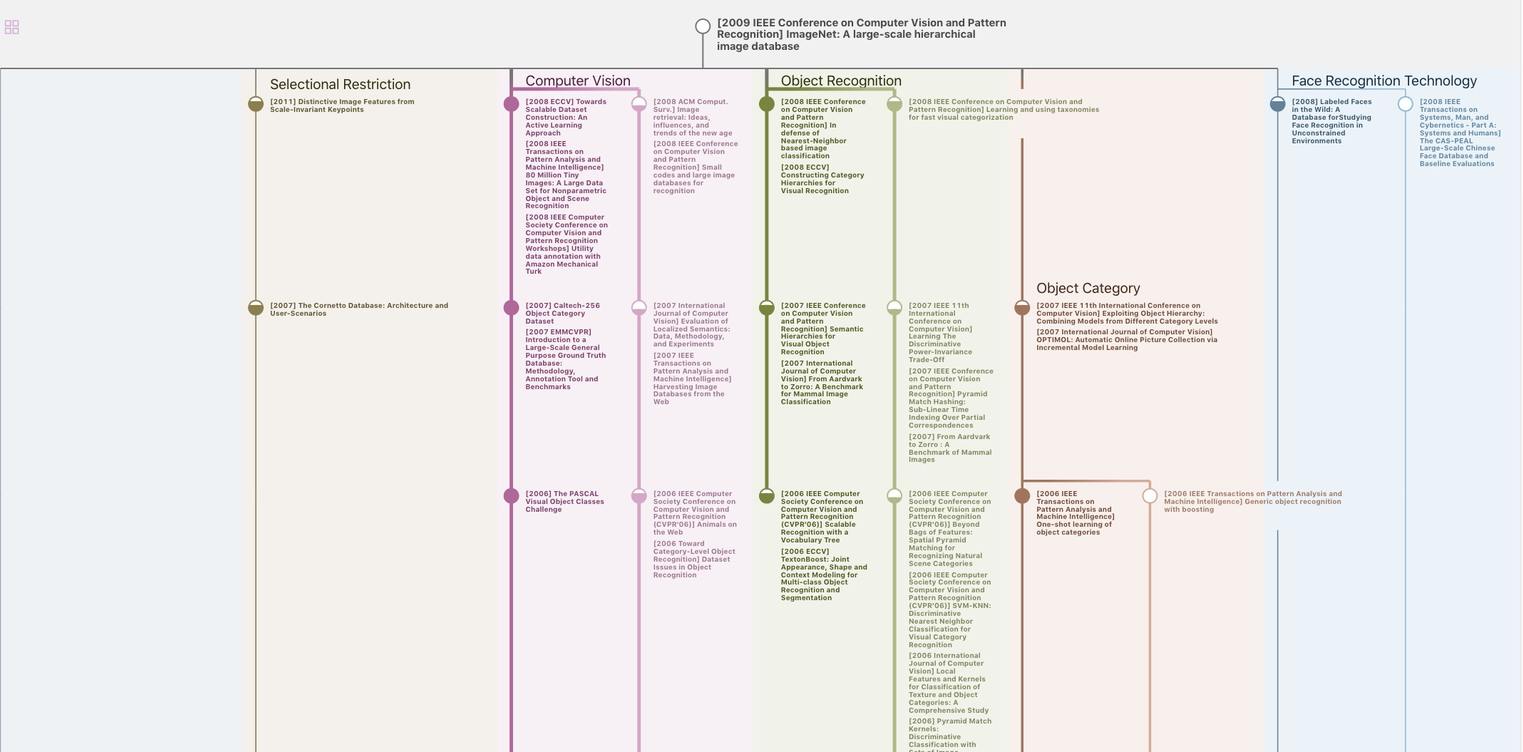
生成溯源树,研究论文发展脉络
Chat Paper
正在生成论文摘要